VLDeformer: Learning Visual-Semantic Embeddings by Vision-Language Transformer Decomposing
Knowledge-Based Systems(2021)
Abstract
Vision-language transformers (VL transformers) have shown impressive accuracy in cross-modal retrieval. However, most of the existing VL transformers use early-interaction dataflow that computes a joint representation for the text-image input. In the retrieval stage, such models need to infer on all the matched text-image combinations, which causes high computing costs. The goal of this paper is to decompose the early-interaction dataflow inside the pre-trained VL transformer to achieve acceleration while maintaining its outstanding accuracy. To achieve this, we propose a novel Vision-language Transformer Decomposing (VLDeformer) to modify the VL transformer as an individual encoder for a single image or text through contrastive learning, which accelerates retrieval speed by thousands of times. Meanwhile, we propose to compose bi-modal hard negatives for the contrastive learning objective, which enables the VLDeformer to maintain the outstanding accuracy of the backbone VL transformer. Extensive experiments on COCO and Flickr30k datasets demonstrate the superior performance of the proposed method. Considering both effectiveness and efficiency, VLDeformer provides a superior selection for cross-modal retrieval in the similar pre-training datascale.
MoreTranslated text
Key words
learning,visual-semantic,vision-language
AI Read Science
Must-Reading Tree
Example
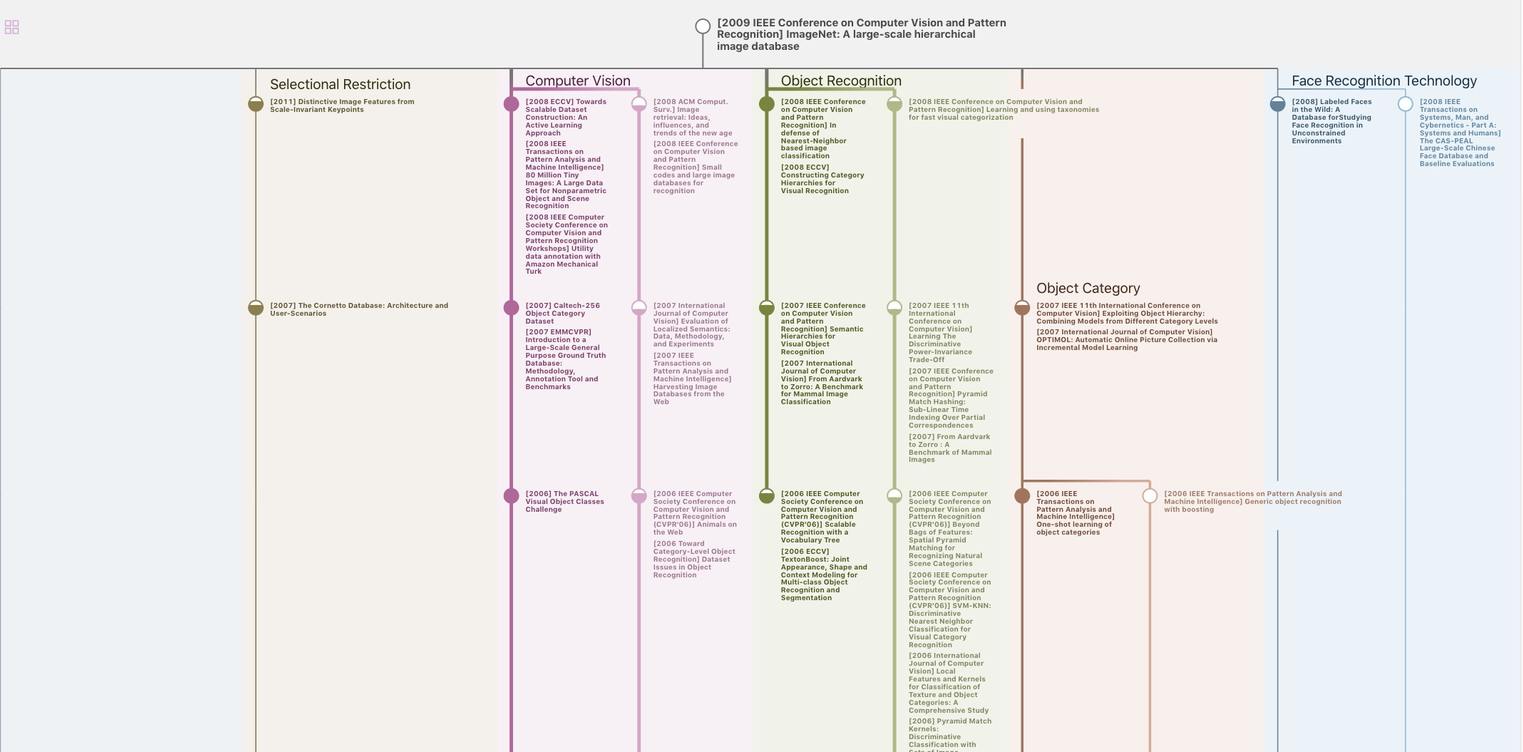
Generate MRT to find the research sequence of this paper
Chat Paper
Summary is being generated by the instructions you defined