Explainable Diabetic Retinopathy Classification Based on Neural-Symbolic Learning
NESY 2021: NEURAL-SYMBOLIC LEARNING AND REASONING(2021)
摘要
In this paper, we propose an explainable diabetic retinopathy (ExplainDR) classification model based on neural-symbolic learning. To gain explainability, a high-level symbolic representation should be considered in decision making. Specifically, we introduce a human-readable symbolic representation, which follows a taxonomy style of diabetic retinopathy characteristics related to eye health conditions to achieve explainability. We then include human-readable features obtained from the symbolic representation in the disease prediction. Experimental results on a diabetic retinopathy classification dataset show that our proposed ExplainDR method exhibits promising performance when compared to that from state-of-the-art methods applied to the IDRiD dataset, while also providing interpretability and explainability.
更多查看译文
关键词
Explainable Artificial Intelligence, Diabetic Retinopathy, Neural-Symbolic Learning
AI 理解论文
溯源树
样例
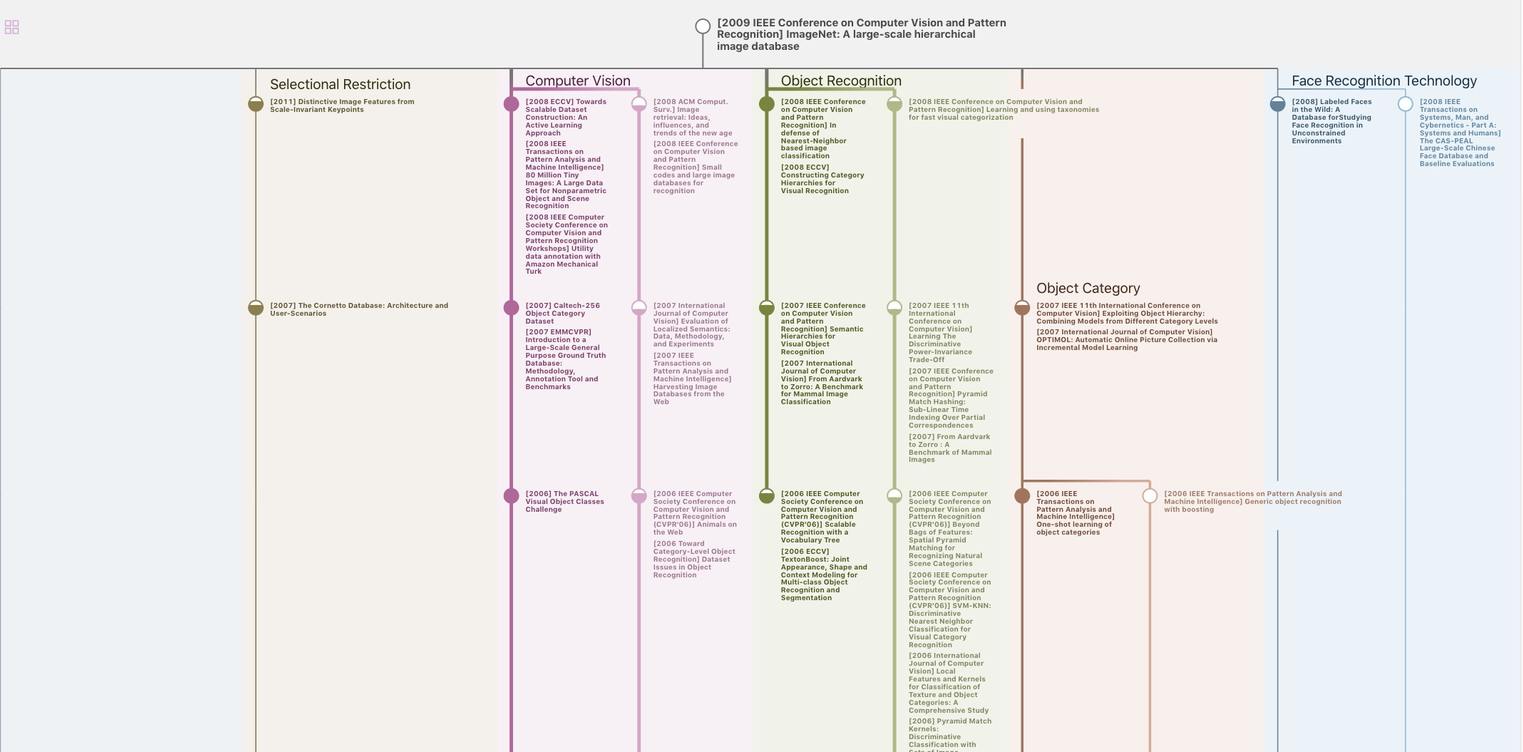
生成溯源树,研究论文发展脉络
Chat Paper
正在生成论文摘要