Pixel-wise Graph Attention Networks for Person Re-identification
International Multimedia Conference(2021)
摘要
ABSTRACTGraph convolutional networks (GCN) is widely used to handle irregular data since it updates node features by using the structure information of graph. With the help of iterated GCN, high-order information can be obtained to further enhance the representation of nodes. However, how to apply GCN to structured data (such as pictures) has not been deeply studied. In this paper, we explore the application of graph attention networks (GAT) in image feature extraction. First of all, we propose a novel graph generation algorithm to convert images into graphs through matrix transformation. It is one magnitude faster than the algorithm based on K Nearest Neighbors (KNN). Then, GAT is used on the generated graph to update the node features. Thus, a more robust representation is obtained. These two steps are combined into a module called pixel-wise graph attention module (PGA). Since the graph obtained by our graph generation algorithm can still be transformed into a picture after processing, PGA can be well combined with CNN. Based on these two modules, we consulted the ResNet and design a pixel-wise graph attention network (PGANet). The PGANet is applied to the task of person re-identification in the datasets Market1501, DukeMTMC-reID and Occluded-DukeMTMC (outperforms state-of-the-art by 0.8%, 1.1% and 11% respectively, in mAP scores). Experiment results show that it achieves the state-of-the-art performance.
更多查看译文
关键词
attention,graph,person,pixel-wise,re-identification
AI 理解论文
溯源树
样例
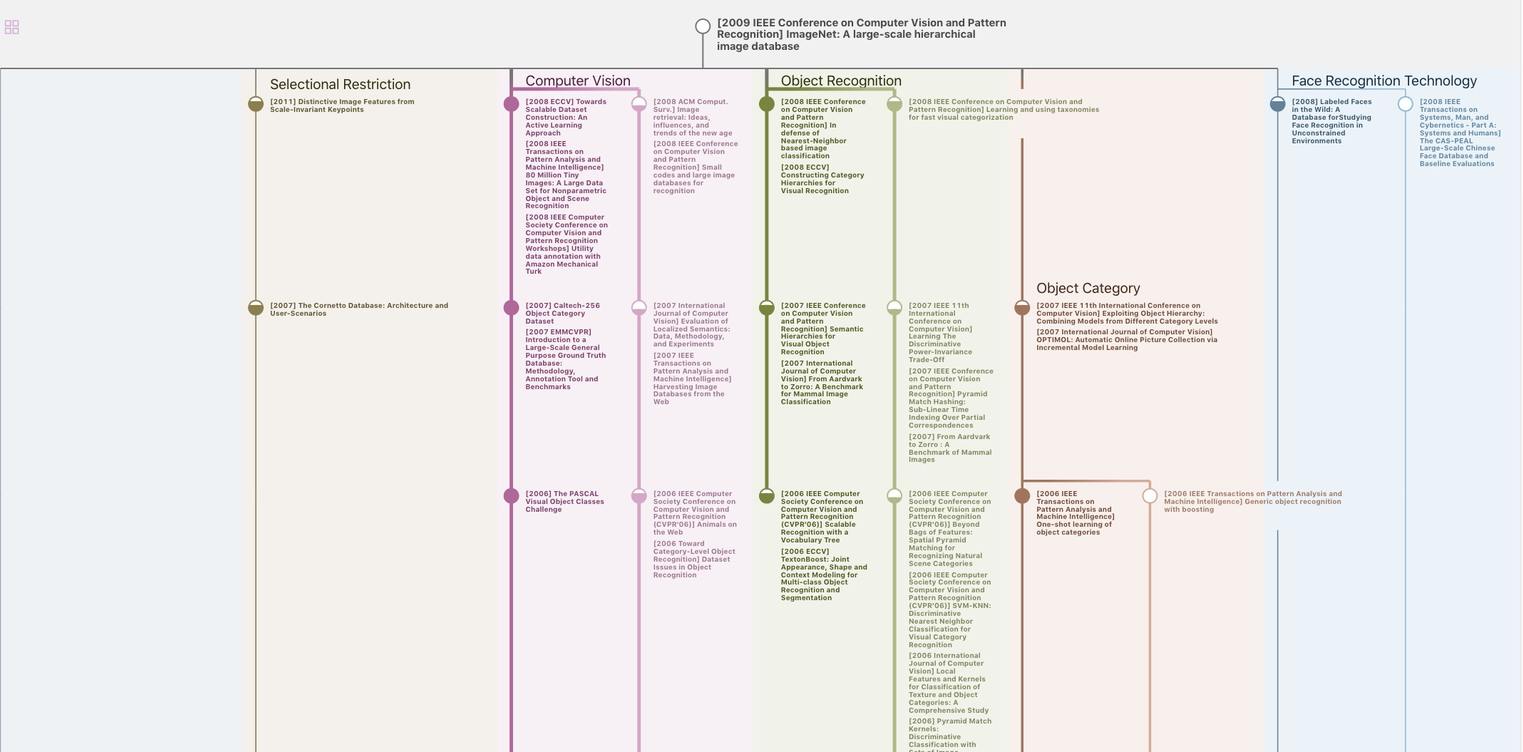
生成溯源树,研究论文发展脉络
Chat Paper
正在生成论文摘要