VmAP: A Fair Metric for Video Object Detection
International Multimedia Conference(2021)
摘要
ABSTRACTVideo object detection is the task of detecting objects in a sequence of frames, typically, with a significant overlap in content among consecutive frames. Mean Average Precision (mAP) was originally proposed for evaluating object detection techniques in independent frames, but has been used for evaluating video based object detectors as well. This is undesirable since the average precision over all frames masks the biases that a certain object detector might have against certain types of objects depending on the number of frames for which the object is present in a video sequence. In this paper we show several disadvantages of mAP as a metric for evaluating video based object detection. Specifically, we show that: (a) some object detectors could be severely biased against some specific kind of objects, such as small, blurred, or low contrast objects, and such differences may not reflect in mAP based evaluation, (b) operating a video based object detector at the best frame based precision/recall value (high F1 score) may lead to many false positives without a significant increase in the number of objects detected. (c) mAP does not take into account that tracking can be potentially used to recover missed detections in the temporal neighborhood while this can be account for while evaluating detectors. As an alternate, we suggest a novel evaluation metric (VmAP) which takes the focus away from evaluating detections on every frame. Unlike mAP, VmAP rewards a high recall of different object views throughout the video. We form sets of bounding boxes having similar views of an object in a temporal neighborhood and use a set-level recall for evaluation. We show that VmAP is able to address all the challenges with the mAP listed above. Our experiments demonstrate hidden biases in object detectors, shows upto 99% reduction in false positives while maintaining similar object recall and shows a 9% improvement in correlation with post-tracking performance.
更多查看译文
AI 理解论文
溯源树
样例
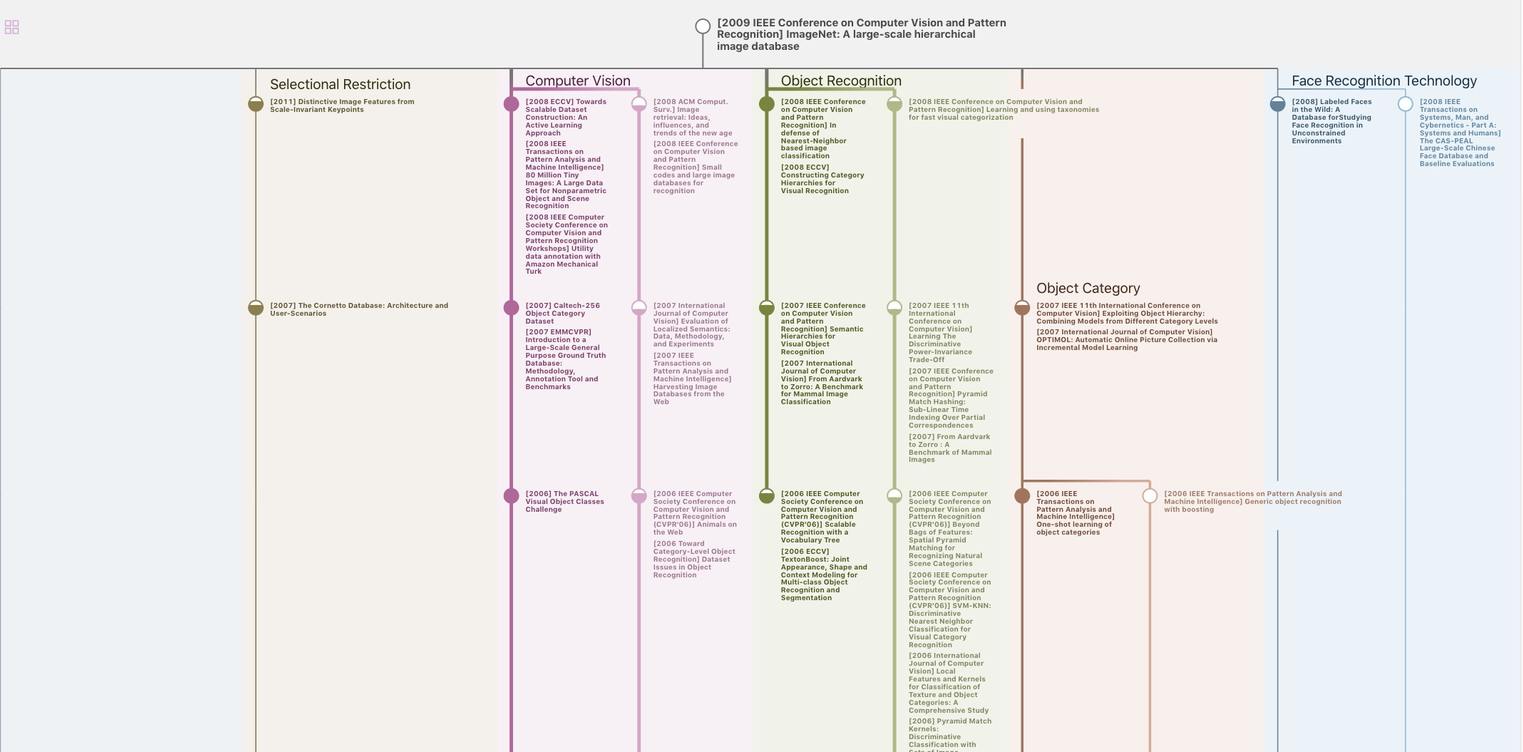
生成溯源树,研究论文发展脉络
Chat Paper
正在生成论文摘要