Imbalanced Source-free Domain Adaptation
International Multimedia Conference(2021)
摘要
ABSTRACTConventional Unsupervised Domain Adaptation (UDA) aims to transfer knowledge from a well-labeled source domain to an unlabeled target domain only when data from both domains is simultaneously accessible, which is challenged by the recent Source-free Domain Adaptation (SFDA). However, we notice that the performance of existing SFDA methods would be dramatically degraded by intra-domain class imbalance and inter-domain label shift. Unfortunately, class-imbalance is a common phenomenon in real-world domain adaptation applications. To address this issue, we present Imbalanced Source-free Domain Adaptation (ISFDA) in this paper. Specifically, we first train a uniformed model from the source domain, and then propose secondary label correction, curriculum sampling, plus intra-class tightening and inter-class separation to overcome the joint presence of covariate shift and label shift. Extensive experiments on three imbalanced benchmarks verify that ISFDA could perform favorably against existing UDA and SFDA methods under various conditions of class-imbalance, and outperform existing SFDA methods by over 15% in terms of per-class average accuracy on a large-scale long-tailed imbalanced dataset.
更多查看译文
关键词
domain adaptation,source-free
AI 理解论文
溯源树
样例
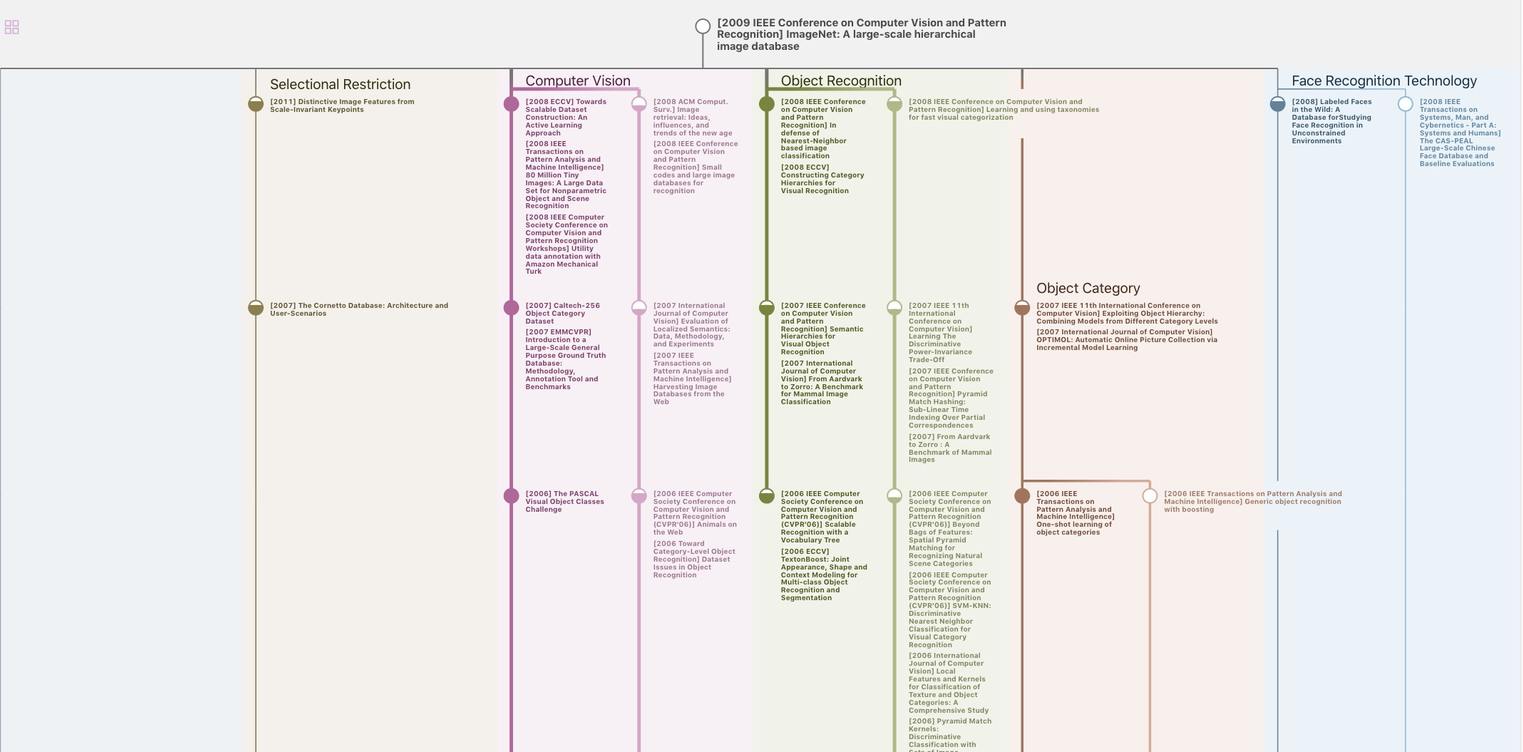
生成溯源树,研究论文发展脉络
Chat Paper
正在生成论文摘要