Information-Growth Attention Network for Image Super-Resolution
PROCEEDINGS OF THE 29TH ACM INTERNATIONAL CONFERENCE ON MULTIMEDIA, MM 2021(2021)
Key words
deep learning,image super-resolution,attention mechanism
AI Read Science
Must-Reading Tree
Example
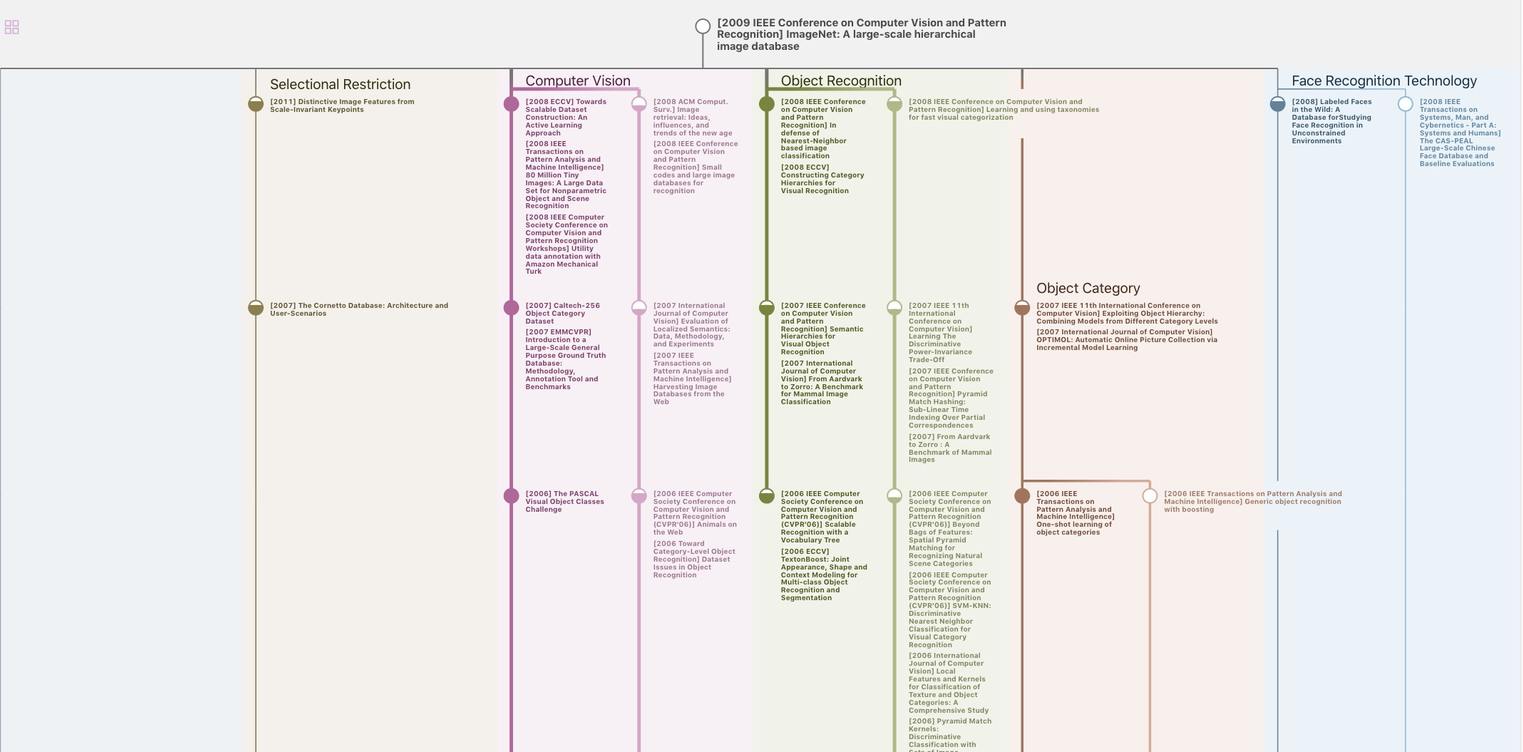
Generate MRT to find the research sequence of this paper
Chat Paper
Summary is being generated by the instructions you defined