DIESEL+: Accelerating Distributed Deep Learning Tasks on Image Datasets
IEEE Transactions on Parallel and Distributed Systems(2022)
摘要
We observe that data access and processing takes a significant amount of time in large-scale deep learning training tasks (DLTs) on image datasets. Three factors contribute to this problem: (1) the massive and recurrent accesses to large numbers of small files; (2) the repeated, expensive decoding computation on each image, and (3) the frequent communication between computation nodes and storage nodes. Existing work has addressed some aspects of these problems; however, no end-to-end solutions have been proposed. In this article, we propose DIESEL+, an all-in-one system which accelerates the entire I/O pipeline of deep learning training tasks. DIESEL+ contains several components: (1) local metadata snapshot; (2) per-task distributed caching; (3) chunk-wise shuffling; (4) GPU-assisted image decoding and (5) online region-of-interest (ROI) decoding. The metadata snapshot removes the bottleneck on metadata access in frequent reading of large numbers of files. The per-task distributed cache across the worker nodes of a DLT task to reduce the I/O pressure on the underlying storage. The chunk-based shuffle method converts small file reads into large chunk reads, so that the performance is improved without sacrificing the training accuracy. The GPU-assisted image decoding and the online ROI method minimize the image decoding workloads and reduce the cost of data movement between nodes. These techniques are seamlessly integrated into the system. In our experiments, DIESEL+ outperforms existing systems by a factor of two to three times on the overall training time.
更多查看译文
关键词
Storage system,dataset management,deep learning,distributed cache,dataset shuffling,image decoding,GPU
AI 理解论文
溯源树
样例
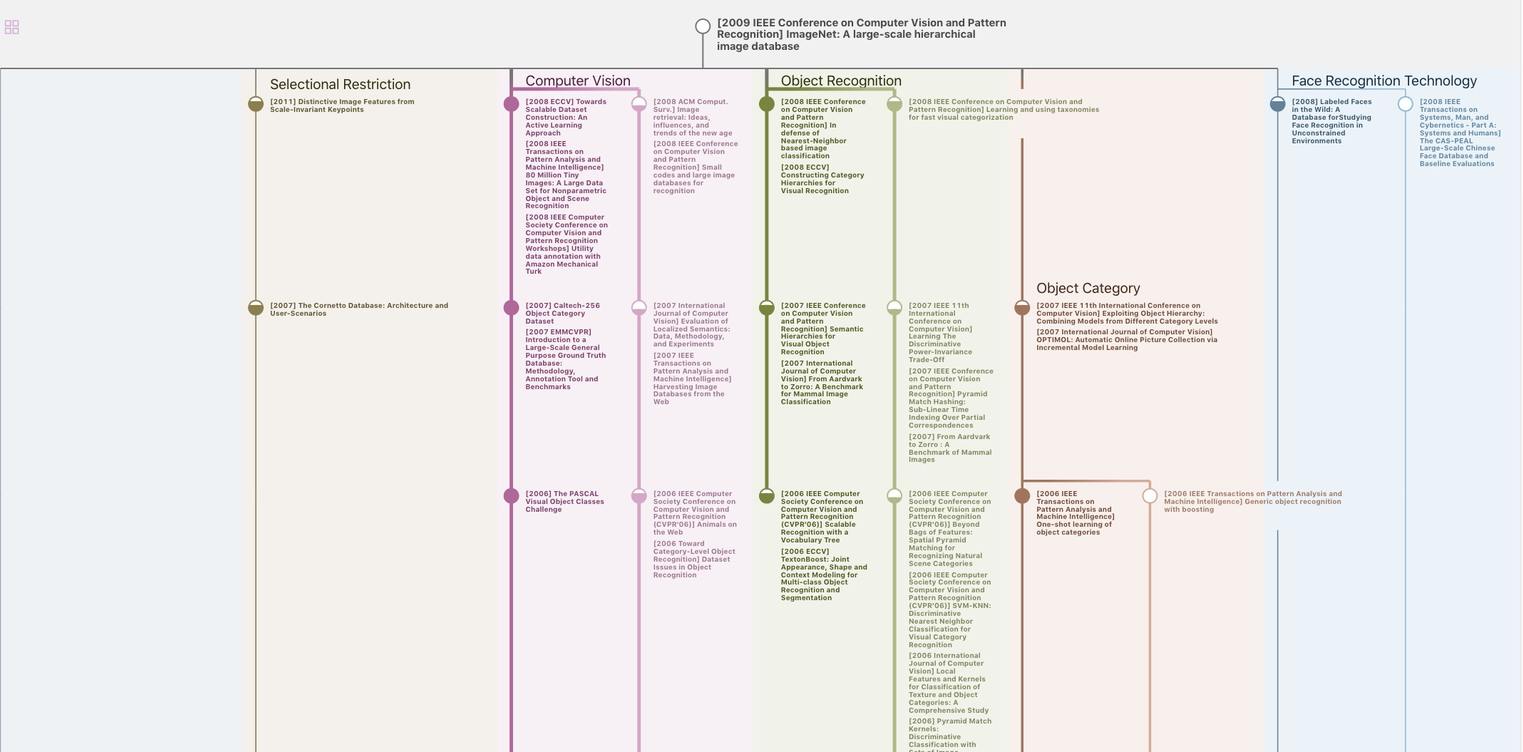
生成溯源树,研究论文发展脉络
Chat Paper
正在生成论文摘要