Rgbxd: Learning Depth-Weighted Rgb Patches For Rgb-D Indoor Semantic Segmentation
NEUROCOMPUTING(2021)
摘要
Significant advances have been made in designing CNNs for RGB semantic segmentation. However, these CNNs are not widely adopted for RGB-D segmentation, due to the asymmetry between the RGB and depth modalities. Instead, dedicated architectures are designed to fuse them for effective RGB-D segmentation, wherein complex structures are often employed, resulting in much increased computational cost. In this paper, we propose a novel way to learn the fusion of RGB and depth information in an early stage. This enables our method to easily adopt existing RGB segmentation networks with minimal modification. Our method is simple yet effective to build a bridge between RGB and RGBD semantic segmentation, so as to avoid designing a far more complex network structure for RGBD segmentation. The proposed method treats RGB and depth information in an inherently asymmetric manner, and to the best of our knowledge, this is the first approach that learns to fuse them in a multiplicative manner for RGB-D segmentation; thus, we call it RGBxD. Extensive experiments and ablation studies on the challenging NYUDv2, SUN RGB-D and Cityscapes semantic segmentation benchmarks show that the proposed RGBxD offers a consistent improvement over several baselines. (c) 2021 Elsevier B.V. All rights reserved.
更多查看译文
关键词
RGB-D indoor semantic segmentation, Depth information, Deep learning
AI 理解论文
溯源树
样例
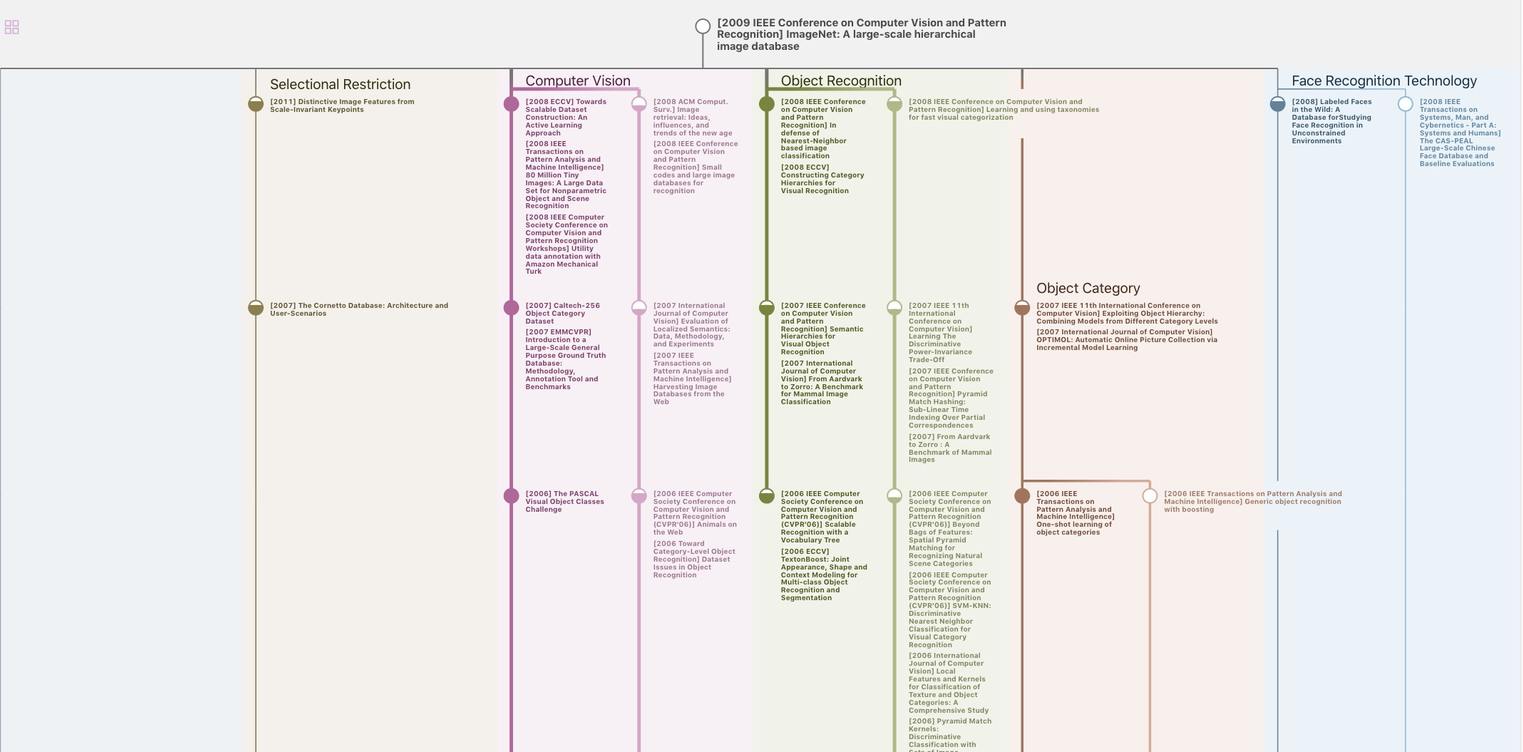
生成溯源树,研究论文发展脉络
Chat Paper
正在生成论文摘要