Miniexpnet: A Small And Effective Facial Expression Recognition Network Based On Facial Local Regions
NEUROCOMPUTING(2021)
Abstract
Deep networks based Facial Expression Recognition (FER) have shown excellent performance. Due to high computational cost and memory resources, it is hard to develop these FER models in practical scenarios. In this paper, we design a small and effective deep network for FER based on facial local regions. Firstly, Regions of Interest (ROIs) related to facial Action Units (AUs) are cropped by facial landmarks and represented by Histogram Local Binary Pattern (HLBP) feature. Secondly, an effective and efficient light-weight network named MiniExpNet, is proposed by human-machine collaborative strategy, and only has 60 K parameters. To further improve the performance, we extend the MiniExpNet by integrating the Attention Block and self-distillation mechanism. The Attention Block exploits the importance of different ROIs for FER. The self-distillation mechanism is used for enhancing generalization ability during training. Extensive comparisons are made on three widely-used FER datasets, CK+, RaFD and Oulu-CASIA. Compared to exiting light-weight networks, the proposed model provides a better balance between accuracy, model size and speed. (c) 2021 Elsevier B.V. All rights reserved.
MoreTranslated text
Key words
Facial expression recognition, Facial local regions, Action Unit, HLBP feature, Light-weight network
AI Read Science
Must-Reading Tree
Example
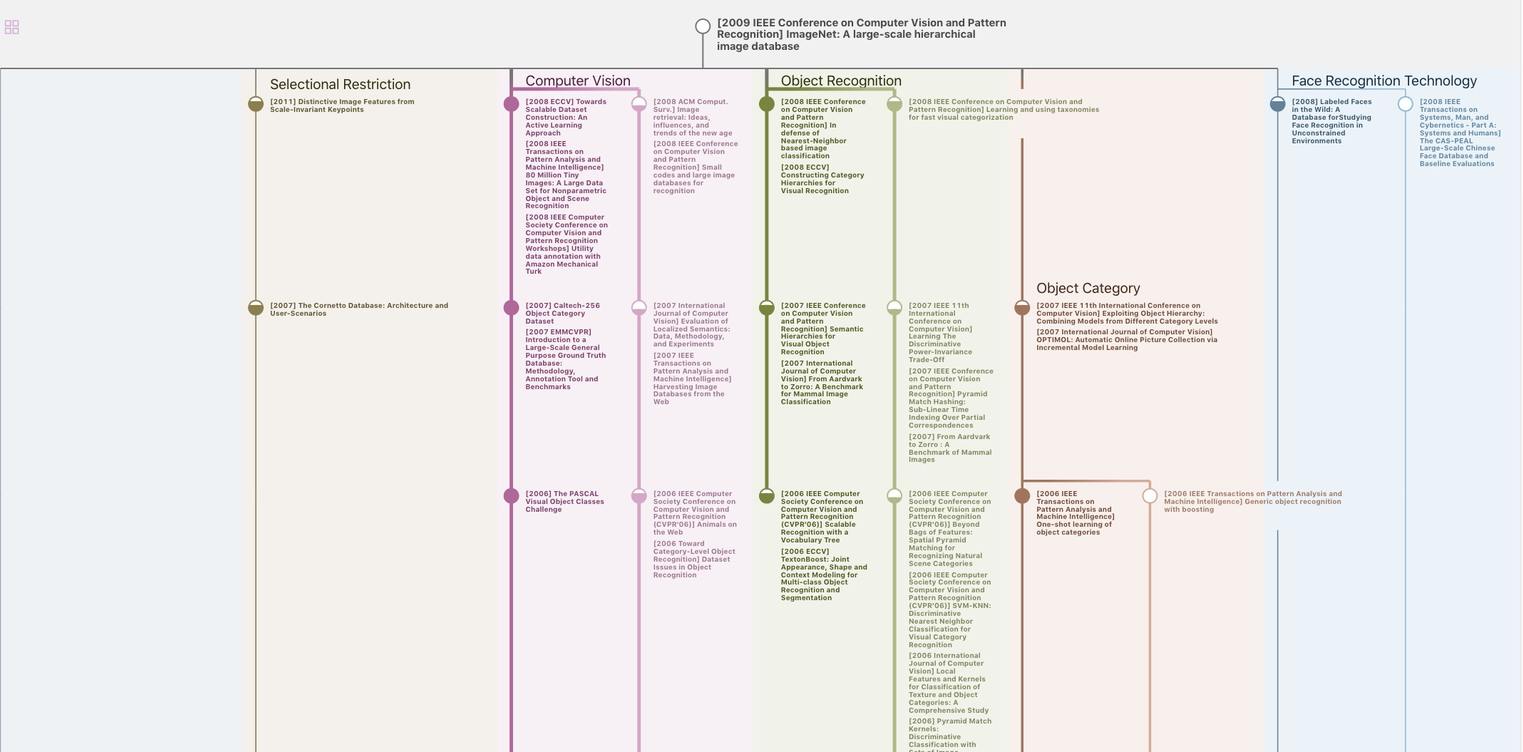
Generate MRT to find the research sequence of this paper
Chat Paper
Summary is being generated by the instructions you defined