User-friendly Introduction to PAC-Bayes Bounds
FOUNDATIONS AND TRENDS IN MACHINE LEARNING(2024)
摘要
Aggregated predictors are obtained by making a set of basic predictors vote according to some weights, that is, to some probability distribution. Randomized predictors are obtained by sampling in a set of basic predictors, according to some prescribed probability distribution. Thus, aggregated and randomized predictors have in common that their definition rely on a probability distribution on the set of predictors. In statistical learning theory, there is a set of tools designed to understand the generalization ability of such predictors: PAC-Bayesian or PAC-Bayes bounds. Since the original PAC-Bayes bounds (Shawe-Taylor and Williamson, 1997; McAllester, 1998), these tools have been considerably improved in many directions. We will for example describe a simplified version of the localization technique (Catoni, 2003; Catoni, 2007) that was missed by the community, and later rediscovered as "mutual information bounds". Very recently, PAC-Bayes bounds received a considerable attention. There was workshop on PAC-Bayes at NIPS 2017, (Almost) 50 Shades of Bayesian Learning: PAC-Bayesian trends and insights, organized by B. Guedj, F. Bach and P. Germain. One of the reasons of this recent interest is the successful application of these bounds to neural networks (Dziugaite and Roy, 2017). Since then, this is a recurring topic of workshops in the major machine learning conferences. The objective of these notes is to provide an elementary introduction to PAC-Bayes bounds.
更多查看译文
AI 理解论文
溯源树
样例
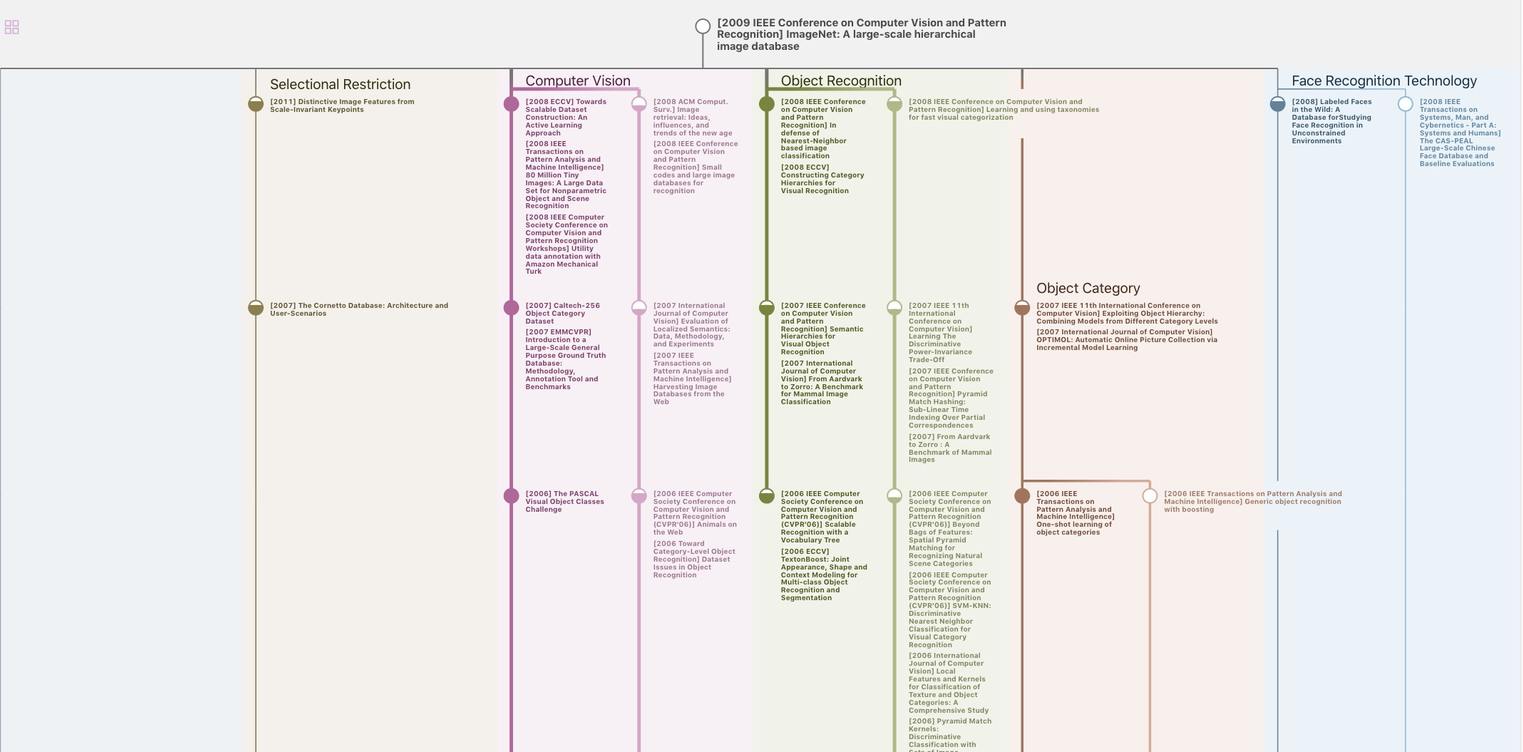
生成溯源树,研究论文发展脉络
Chat Paper
正在生成论文摘要