Self-Supervised Visual Representation Learning Using Lightweight Architectures
arxiv(2021)
摘要
In self-supervised learning, a model is trained to solve a pretext task, using a data set whose annotations are created by a machine. The objective is to transfer the trained weights to perform a downstream task in the target domain. We critically examine the most notable pretext tasks to extract features from image data and further go on to conduct experiments on resource constrained networks, which aid faster experimentation and deployment. We study the performance of various self-supervised techniques keeping all other parameters uniform. We study the patterns that emerge by varying model type, size and amount of pre-training done for the backbone as well as establish a standard to compare against for future research. We also conduct comprehensive studies to understand the quality of representations learned by different architectures.
更多查看译文
关键词
visual representation learning,lightweight architectures,self-supervised
AI 理解论文
溯源树
样例
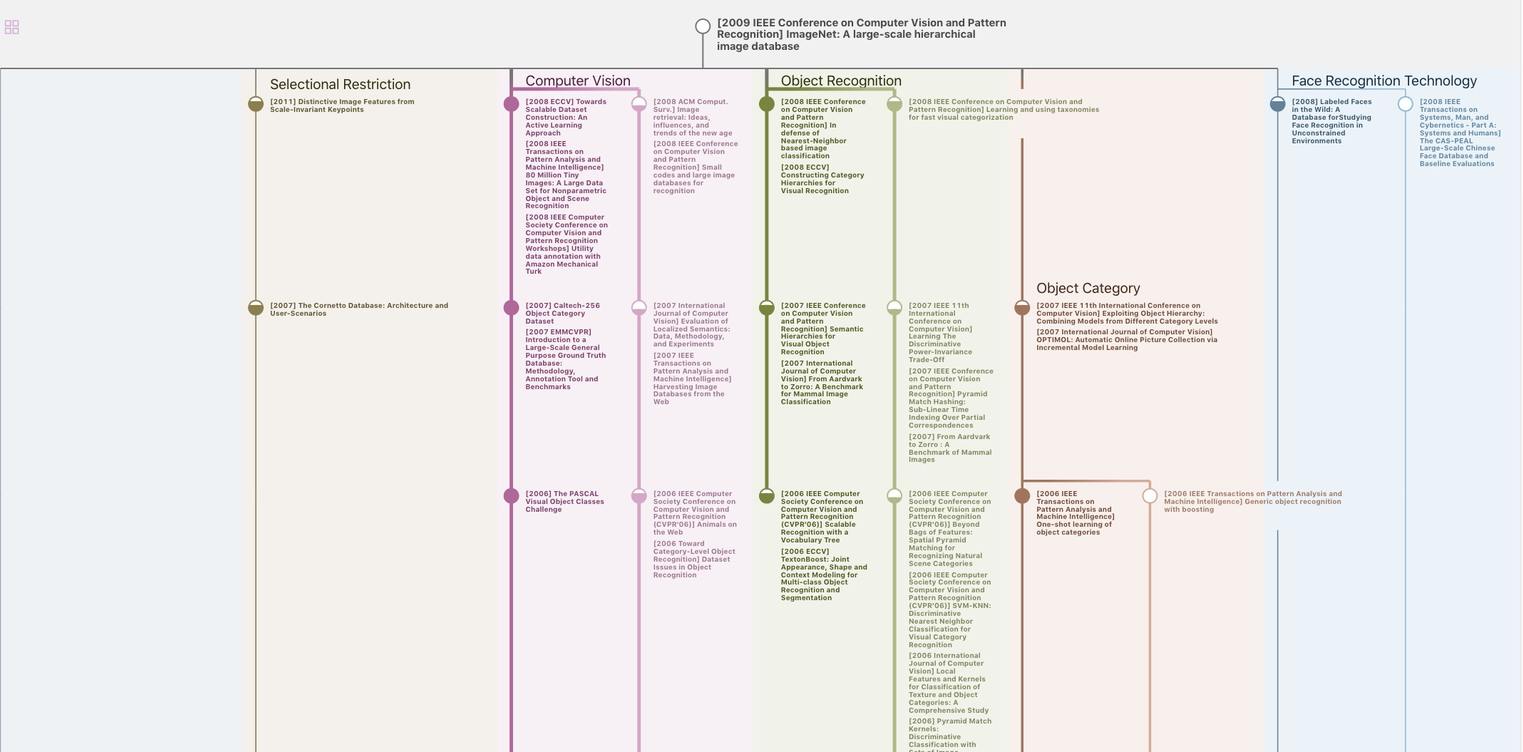
生成溯源树,研究论文发展脉络
Chat Paper
正在生成论文摘要