A Strong Baseline for Semi-Supervised Incremental Few-Shot Learning.
British Machine Vision Conference(2021)
摘要
Few-shot learning (FSL) aims to learn models that generalize to novel classes with limited training samples. Recent works advance FSL towards a scenario where unlabeled examples are also available and propose semi-supervised FSL methods. Another line of methods also cares about the performance of base classes in addition to the novel ones and thus establishes the incremental FSL scenario. In this paper, we generalize the above two under a more realistic yet complex setting, named by Semi-Supervised Incremental Few-Shot Learning (S2 I-FSL). To tackle the task, we propose a novel paradigm containing two parts: (1) a well-designed meta-training algorithm for mitigating ambiguity between base and novel classes caused by unreliable pseudo labels and (2) a model adaptation mechanism to learn discriminative features for novel classes while preserving base knowledge using few labeled and all the unlabeled data. Extensive experiments on standard FSL, semi-supervised FSL, incremental FSL, and the firstly built S2 I-FSL benchmarks demonstrate the effectiveness of our proposed method.
更多查看译文
关键词
learning,strong baseline,semi-supervised,few-shot
AI 理解论文
溯源树
样例
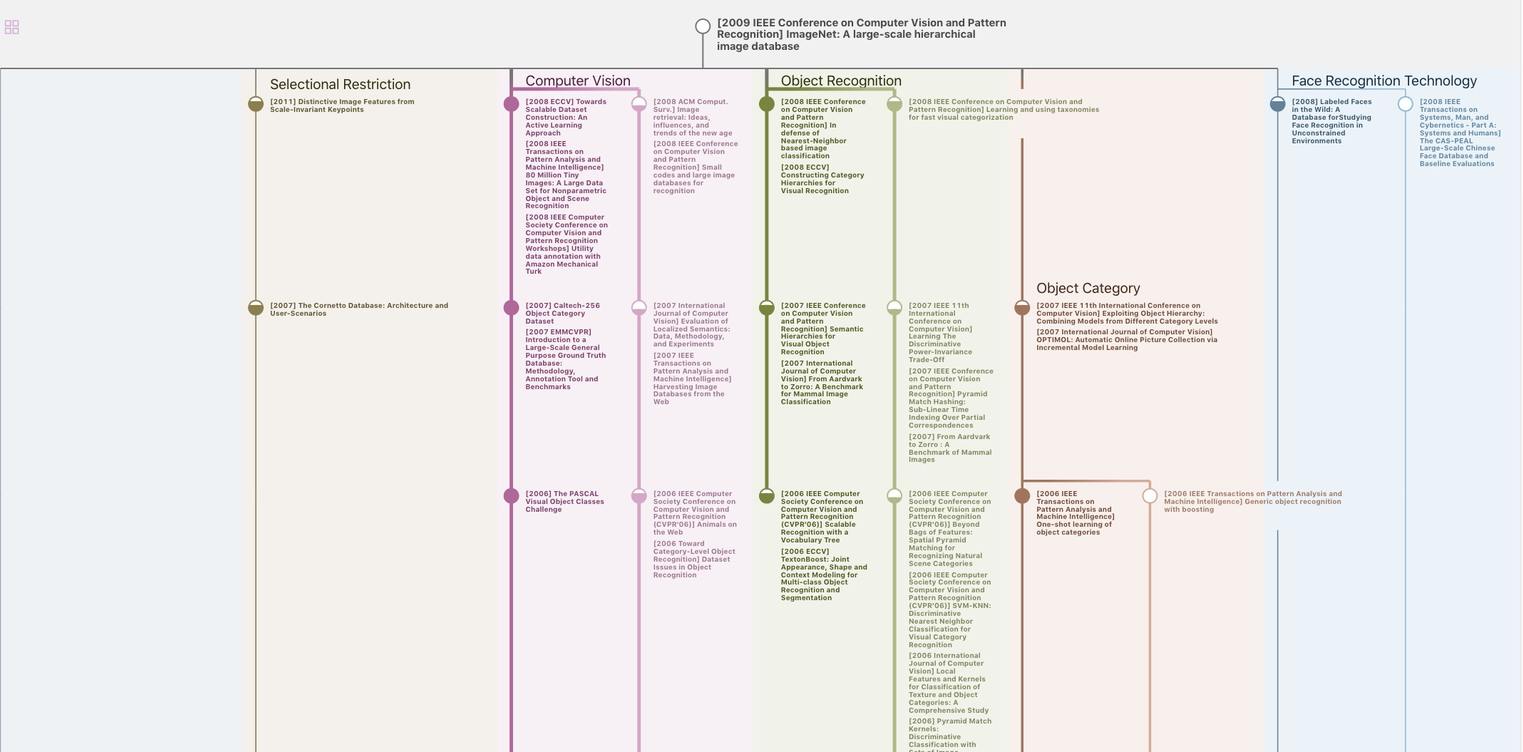
生成溯源树,研究论文发展脉络
Chat Paper
正在生成论文摘要