Exploring Spatial Diversity for Region-Based Active Learning
IEEE TRANSACTIONS ON IMAGE PROCESSING(2021)
摘要
State-of-the-art methods for semantic segmentation are based on deep neural networks trained on large-scale labeled datasets. Acquiring such datasets would incur large annotation costs, especially for dense pixel-level prediction tasks like semantic segmentation. We consider region-based active learning as a strategy to reduce annotation costs while maintaining high performance. In this setting, batches of informative image regions instead of entire images are selected for labeling. Importantly, we propose that enforcing local spatial diversity is beneficial for active learning in this case, and to incorporate spatial diversity along with the traditional active selection criterion, e.g., data sample uncertainty, in a unified optimization framework for region-based active learning. We apply this framework to the Cityscapes and PASCAL VOC datasets and demonstrate that the inclusion of spatial diversity effectively improves the performance of uncertainty-based and feature diversity-based active learning methods. Our framework achieves 95% performance of fully supervised methods with only 5 - 9% of the labeled pixels, outperforming all state-of-the-art region-based active learning methods for semantic segmentation.
更多查看译文
关键词
Uncertainty, Spatial diversity, Semantics, Image segmentation, Task analysis, Labeling, Annotations, Active learning, semantic segmentation
AI 理解论文
溯源树
样例
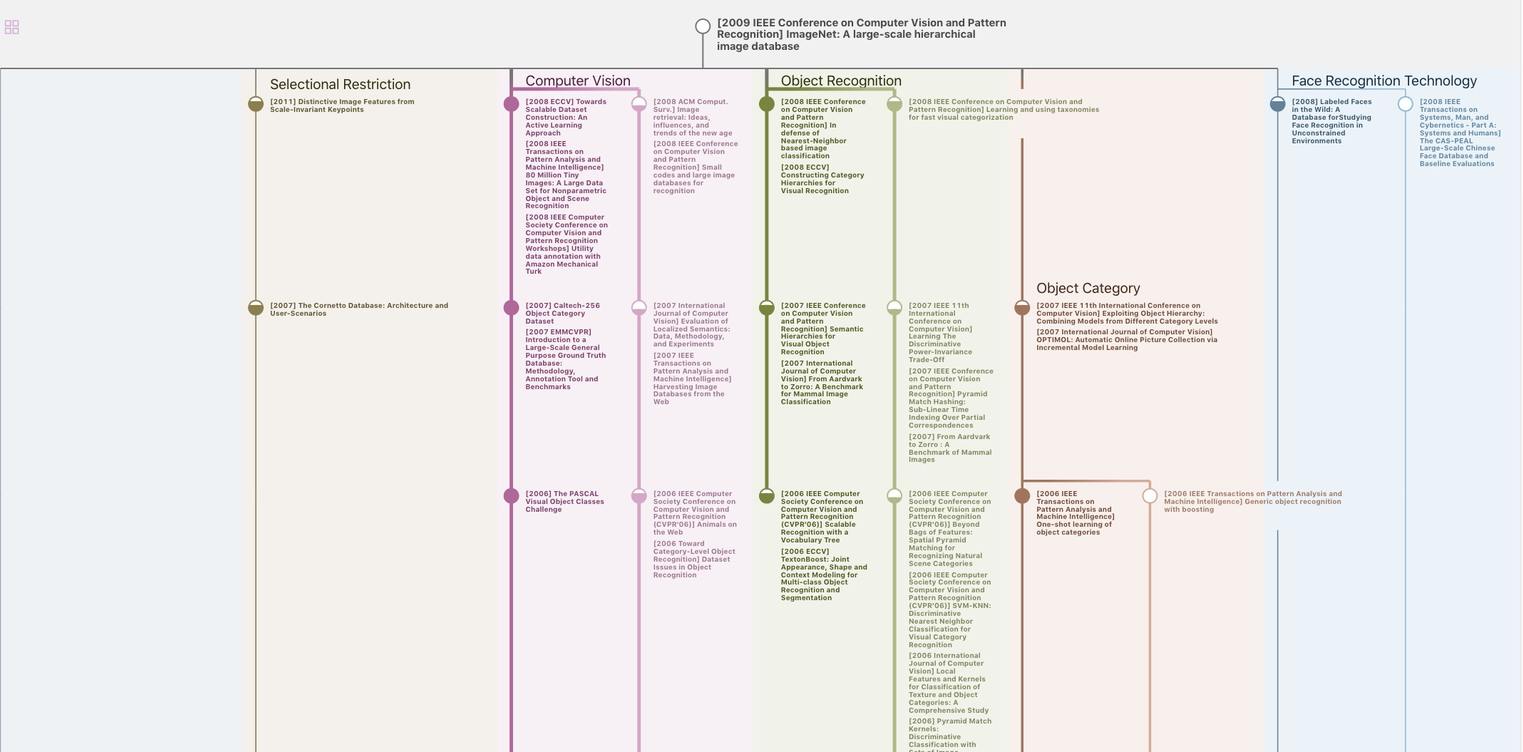
生成溯源树,研究论文发展脉络
Chat Paper
正在生成论文摘要