Spatio-Temporal Super-Resolution Reconstruction of Remote Sensing Data.
IGARSS(2021)
摘要
We present a spatio-temporal super-resolution method for reconstructing a sequence of observations collected by imaging satellites. A sequence of observations is assumed to be defined on a low resolution spatio-temporal grid. It is further assumed that the sequence is generated by blurring of a captured scene with a spatio-temporal convolution kernel and is degraded by noise. Our method simultaneously exhibits deconvolution of the sequence of images from the effects of spatio-temporal blur, denoising of the data, and upsampling of the low-resolution sequence to a high resolution spatiotemporal grid. We perform the super-resolution in the spacetime domain, as opposed to super-resolving the sequence separately and sequentially to a higher spatial and then temporal resolution grid. Simultaneous space-time optimization achieves a more efficient and more accurate reconstruction than reconstructing a sequence frame by frame. The proposed super-resolution methodology is based on total variation regularization and computes the solution using the alternating direction method of multipliers. Numerical results show our approach to be robust and computationally efficient.
更多查看译文
关键词
Alternating direction method of multipliers,upsampling,satellite images,super-resolution
AI 理解论文
溯源树
样例
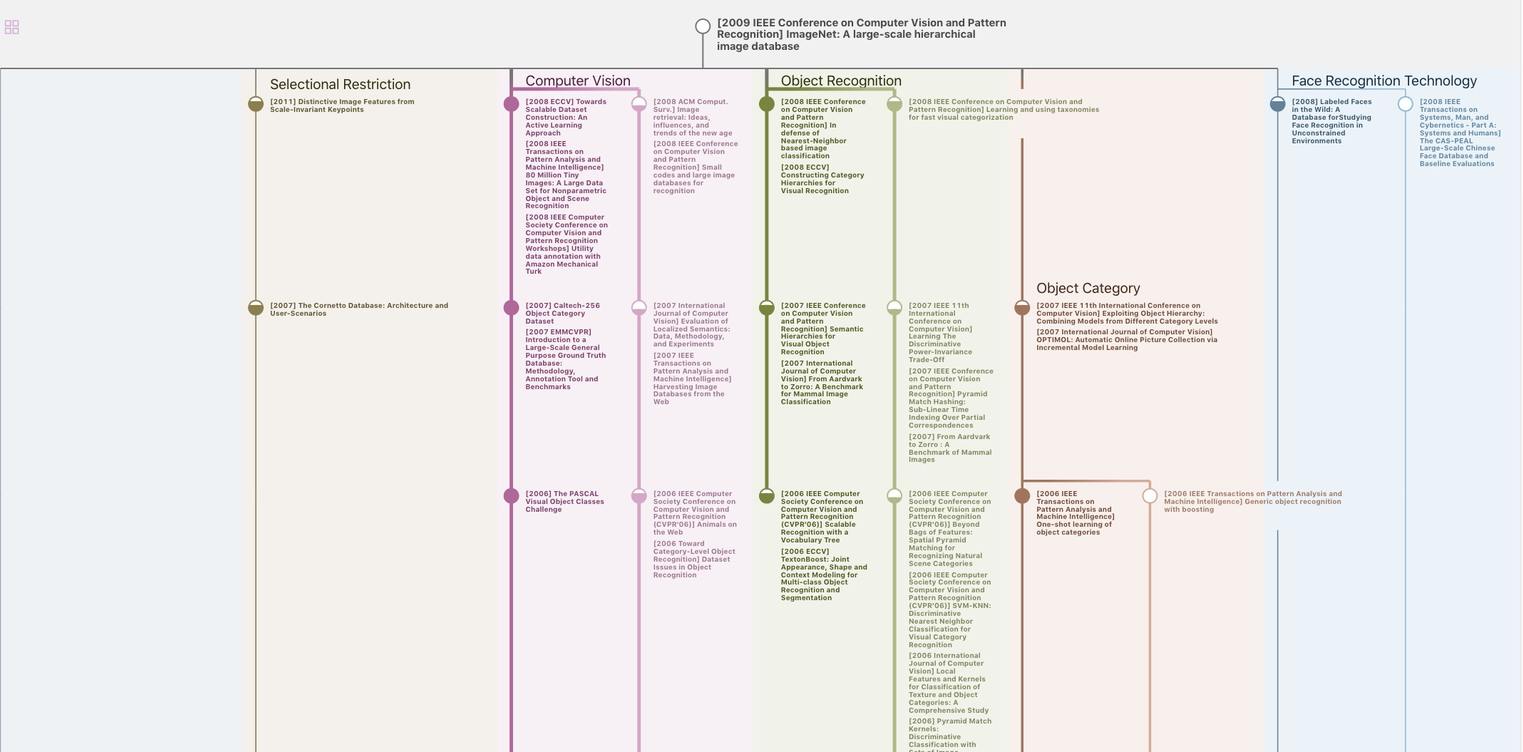
生成溯源树,研究论文发展脉络
Chat Paper
正在生成论文摘要