Hyperspectral Image Denoising with Collaborative Total Variation and Low Rank Regularization.
IGARSS(2021)
摘要
Variational regularization methods are the mainstream methods typically adopted for hyperspectral images (HSIs) denoising, which borrow architectures originally developed for RG-B images, exhibiting limitations when cope with HSI data cubes. To overcome this limitation, this paper proposes a new collaborative total variation and low-rank regularization model (LRCTV) to remove mixed noise from HSI data. Specifically, the proposed method unfolds the HSI cube into 2D extended spectral-matrix, then obtains the horizontal and vertical gradient matrices, and applies 2D collaborative norm to the gradient matrix to model the directional selective smoothness, while the matrix nuclear norm is used to model the low rank structure. Experimental results on both simulated and real HSI datasets validated that the proposed method outperformed several state-of-the-art methods.
更多查看译文
关键词
hyperspectral image,denoising,mixed noise,low-rank regularization,collaborative total variation
AI 理解论文
溯源树
样例
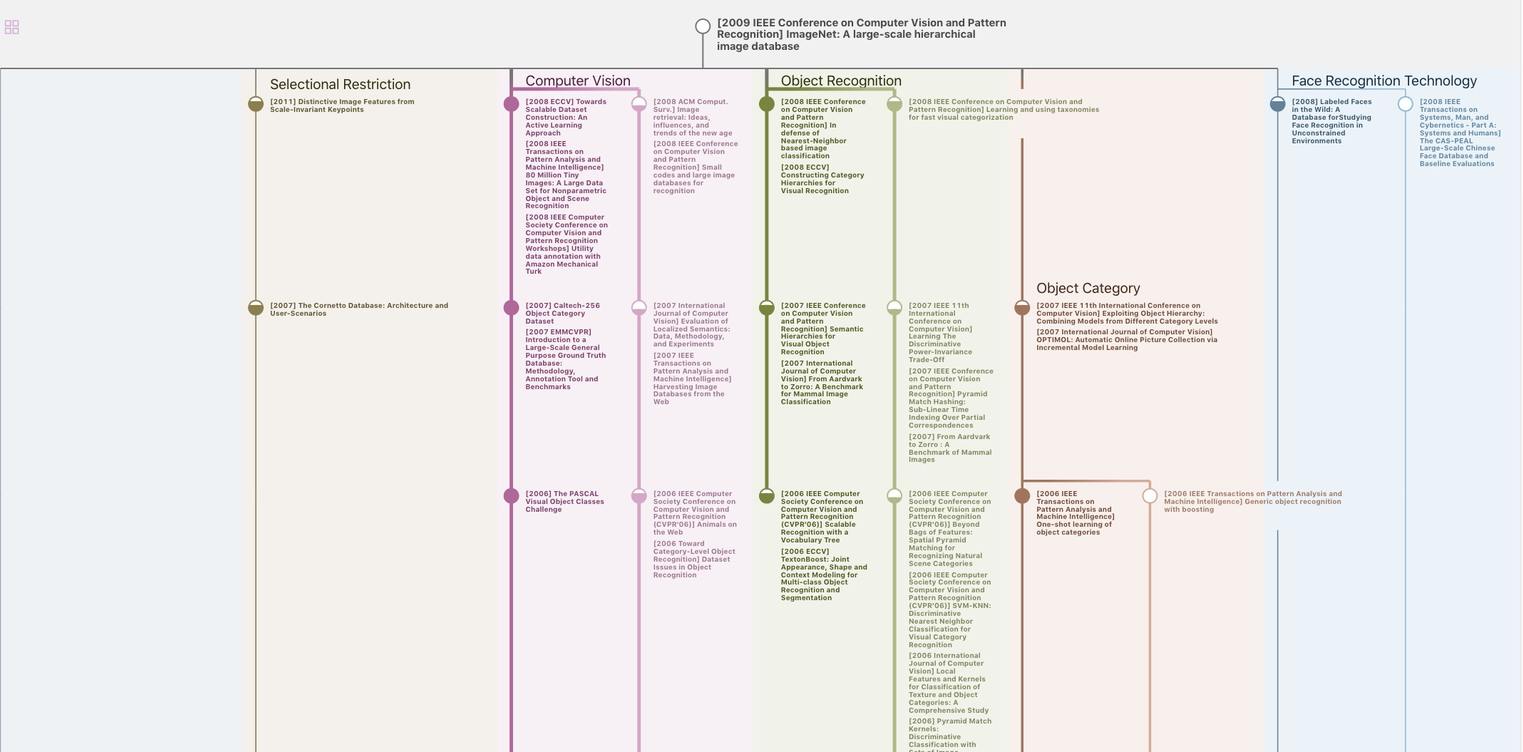
生成溯源树,研究论文发展脉络
Chat Paper
正在生成论文摘要