A Siamese Global Learning Framework for Multi-Class Change Detection
IGARSS(2021)
摘要
Change detection is one of the main tasks in remote sensing field, and is essential for the accurate processing and understanding of the available earth observation data. However, most of the works focus on traditional binary change detection, without considering the change classes information. To make use of the semantic information and analyze change classes comprehensively, we proposed a Siamese Global Learning Framework (Siam-GL) for multi-class change detection. In Siam-GL, the global hierarchically (G-H) sampling strategy is designed to address the imbalanced training sample problem. In addition, the change mask was created and designed to distinguish the changed object for bi-temporal remote sensing images simultaneously. The proposed Siam-GL framework was validated on the Guangzhou dataset of two high spatial resolution remote sensing images in 2013 and 2015, and achieved a better performance than the other state-of-the art methods.
更多查看译文
关键词
Multi-class change detection,remote sensing,imbalanced sample,Siamese network
AI 理解论文
溯源树
样例
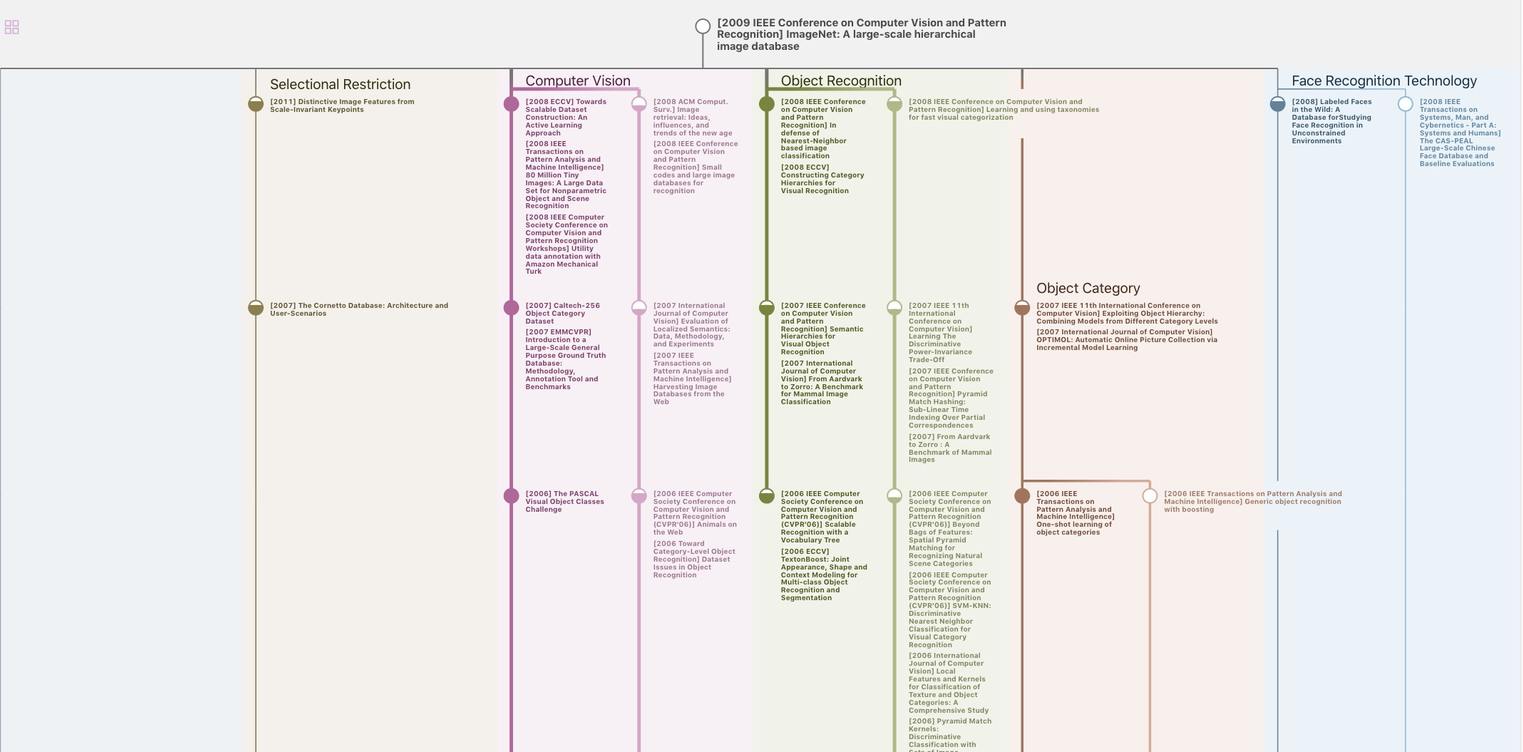
生成溯源树,研究论文发展脉络
Chat Paper
正在生成论文摘要