Multi-Category SAR Images Generation Based on Improved Generative Adversarial Network.
IGARSS(2021)
摘要
The generative adversarial network (GAN) provides a different way for SAR data augmentation. The traditional GAN model is mainly based on the Jensen-Shannon (JS) divergence or Wasserstein distance. The former faces mode collapse, while the latter is not suitable for multi-category image generation. In this paper, an improved model based on WGAN-GP is proposed. An encoder is used to learn the features of real samples as the input of the generator to control training to a certain extent and make the generated image quality better. In addition, a pre-trained classifier is introduced as the constraint of the generator to ensure the generated images have the correct category information. MSTAR dataset is used to verify the generation capability of the proposed model. The results show that the proposed model has the stable generation capability to provide high-quality SAR images as a supplementary training dataset, which could assist in achieving good classification accuracy.
更多查看译文
关键词
P-band,calibration,BIOMASS,parabolic antenna,target of opportunity
AI 理解论文
溯源树
样例
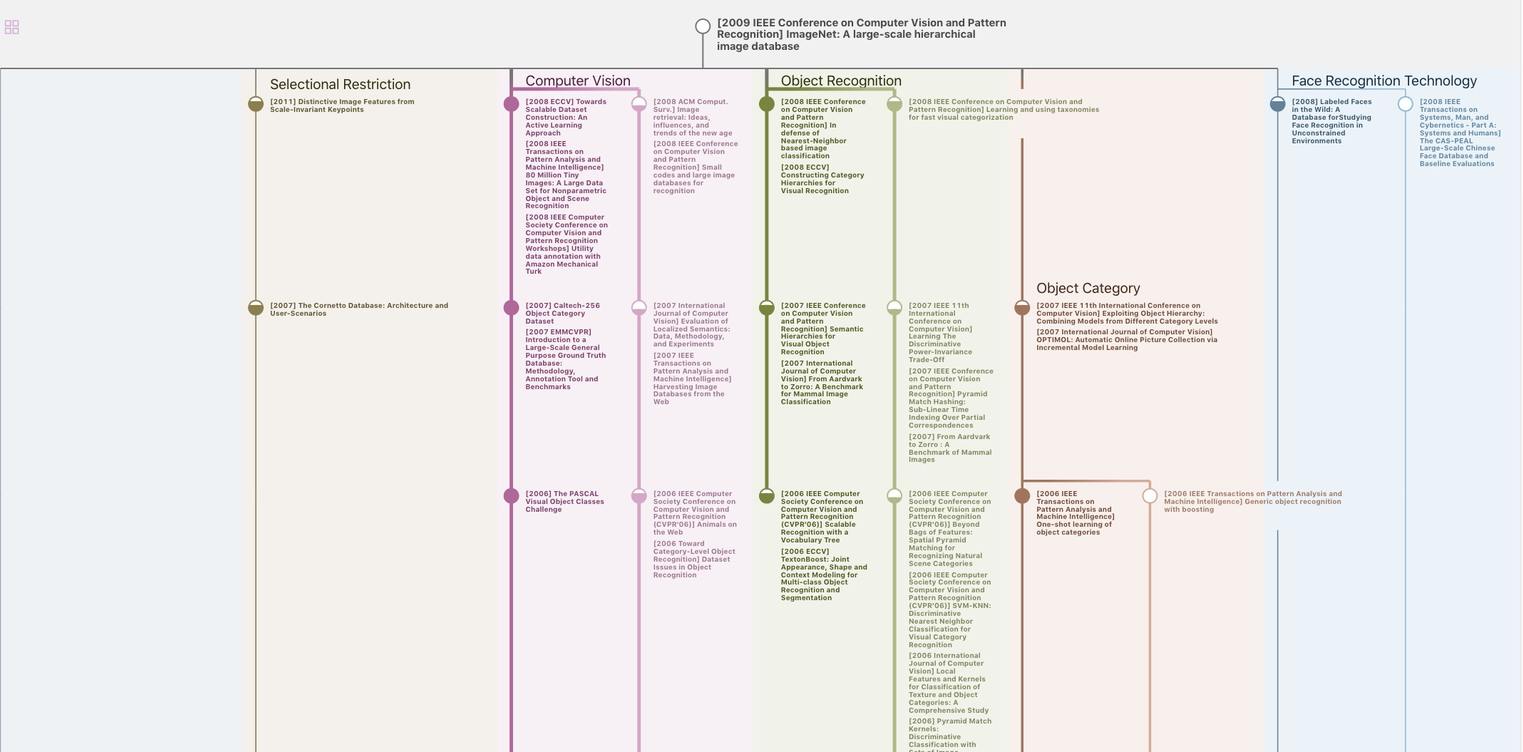
生成溯源树,研究论文发展脉络
Chat Paper
正在生成论文摘要