Data-driven and Automatic Surface Texture Analysis Using Persistent Homology
ICMLA(2021)
摘要
Surface roughness plays an important role in analyzing engineering surfaces. It quantifies the surface topography and can be used to determine whether the resulting surface finish is acceptable or not. Nevertheless, while several existing tools and standards are available for computing surface roughness, these methods rely heavily on user input thus slowing down the analysis and increasing manufacturing costs. Therefore, fast and automatic determination of the roughness level is essential to avoid costs resulting from surfaces with unacceptable finish, and user-intensive analysis. In this study, we propose a Topological Data Analysis (TDA) based approach to classify the roughness level of synthetic surfaces using both their areal images and profiles. We utilize persistent homology from TDA to generate persistence diagrams that encapsulate information on the shape of the surface. We then obtain feature matrices for each surface or profile using Carlsson coordinates, persistence images, and template functions. We compare our results to two widely used methods in the literature: Fast Fourier Transform (FFT) and Gaussian filtering. The results show that our approach yields mean accuracies as high as 97%. We also show that, in contrast to existing surface analysis tools, our TDA-based approach is fully automatable and provides adaptive feature extraction.
更多查看译文
关键词
Surface texture analysis,machine learning,topological data analysis,Gaussian filter,fast fourier transform
AI 理解论文
溯源树
样例
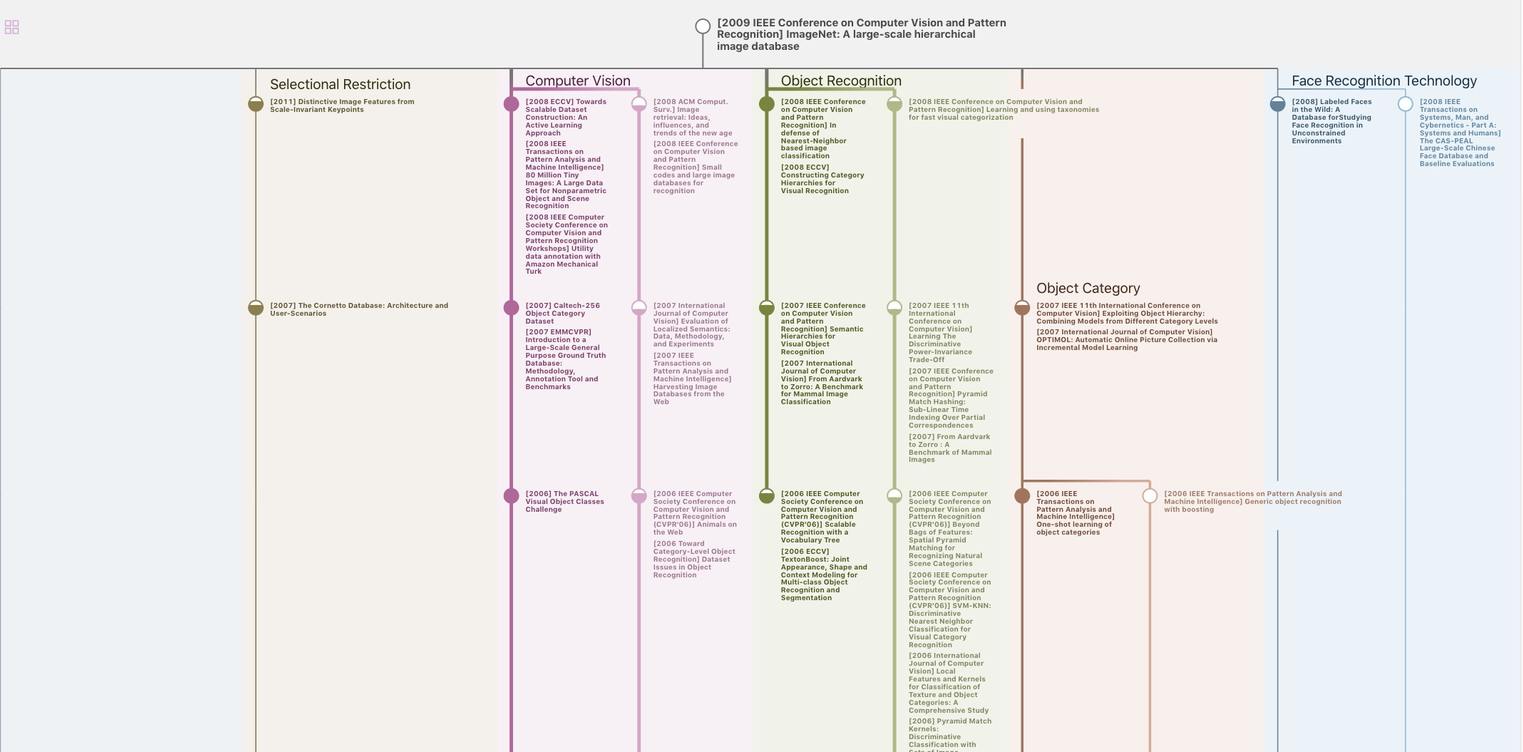
生成溯源树,研究论文发展脉络
Chat Paper
正在生成论文摘要