An evolvable adversarial network with gradient penalty for COVID-19 infection segmentation.
Appl. Soft Comput.(2021)
摘要
COVID-19 infection segmentation has essential applications in determining the severity of a COVID-19 patient and can provide a necessary basis for doctors to adopt a treatment scheme. However, in clinical applications, infection segmentation is performed by human beings, which is time-consuming and generally introduces bias. In this paper, we developed a novel evolvable adversarial framework for COVID-19 infection segmentation. Three generator networks compose an evolutionary population to accommodate the current discriminator, i.e., generator networks evolved with different mutations instead of the single adversarial objective to provide sufficient gradient feedback Compared with the existing work that enforces a Lipschitz constraint by weight clipping, which may lead to gradient exploding or vanishing, the proposed model also incorporates the gradient penalty into the network, penalizing the discriminator's gradient norm input. Experiments on several COVID-19 CT scan datasets verified that the proposed method achieved superior effectiveness and stability for COVID-19 infection segmentation.
更多查看译文
关键词
COVID-19,Evolutionary algorithm,Gradient penalty,Infection segmentation,Wasserstein generative adversarial network
AI 理解论文
溯源树
样例
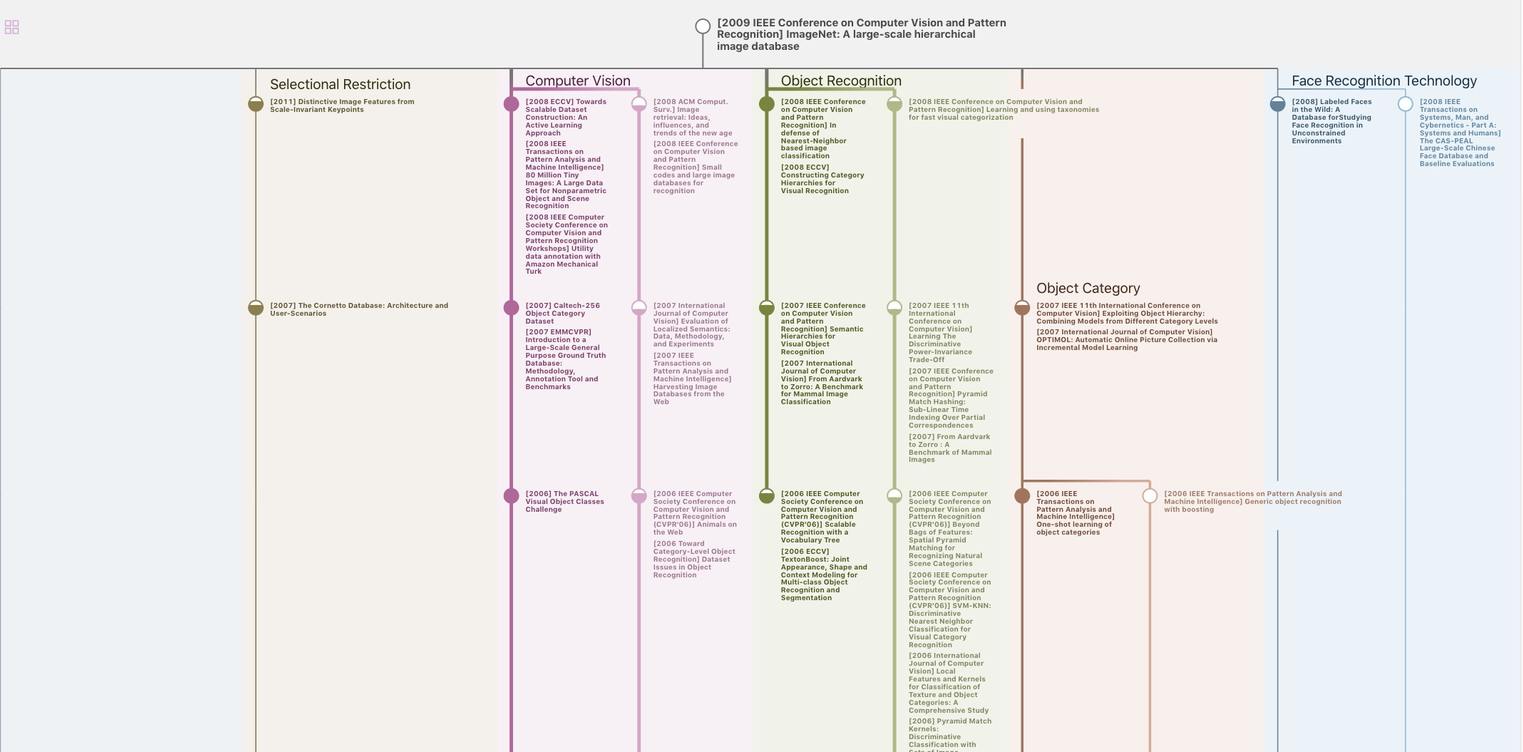
生成溯源树,研究论文发展脉络
Chat Paper
正在生成论文摘要