Automatic Prior Selection For Image Deconvolution: Statistical Modeling On Natural Images
SIGNAL PROCESSING(2021)
摘要
The natural image prior generalizes the heavy-tailed gradient distributions of clear images to L-p regularized problems in the image deconvolution process. Employing a maximum a posteriori estimator, this prior should be carefully selected to precisely model the gradient statistics of the corresponding natural image. However, in several deconvolution algorithms, p has been randomly determined to obtain a high-quality image without considering the essence of the image prior. In this study, we proposed an automatic prior selection strategy based on the statistical properties of restored images. The probabilistic characteristics of the images were derived and investigated by statistically modeling the individual gradient distributions. Subsequently, the regularization term of the objective function was iteratively updated based on the analysis of image restoration. Instead of the unavailable original images, we focused on the utilization of the observed image to estimate the image prior. Overcoming the ill-posedness of the prior selection problem, the proposed algorithm achieved the optimal image prior and effectively restored the degraded image simultaneously. (C) 2021 Elsevier B.V. All rights reserved.
更多查看译文
关键词
Non-blind deconvolution, Regularization, Natural image priors, Statistical modeling, Maximum a posteriori, Prior selection
AI 理解论文
溯源树
样例
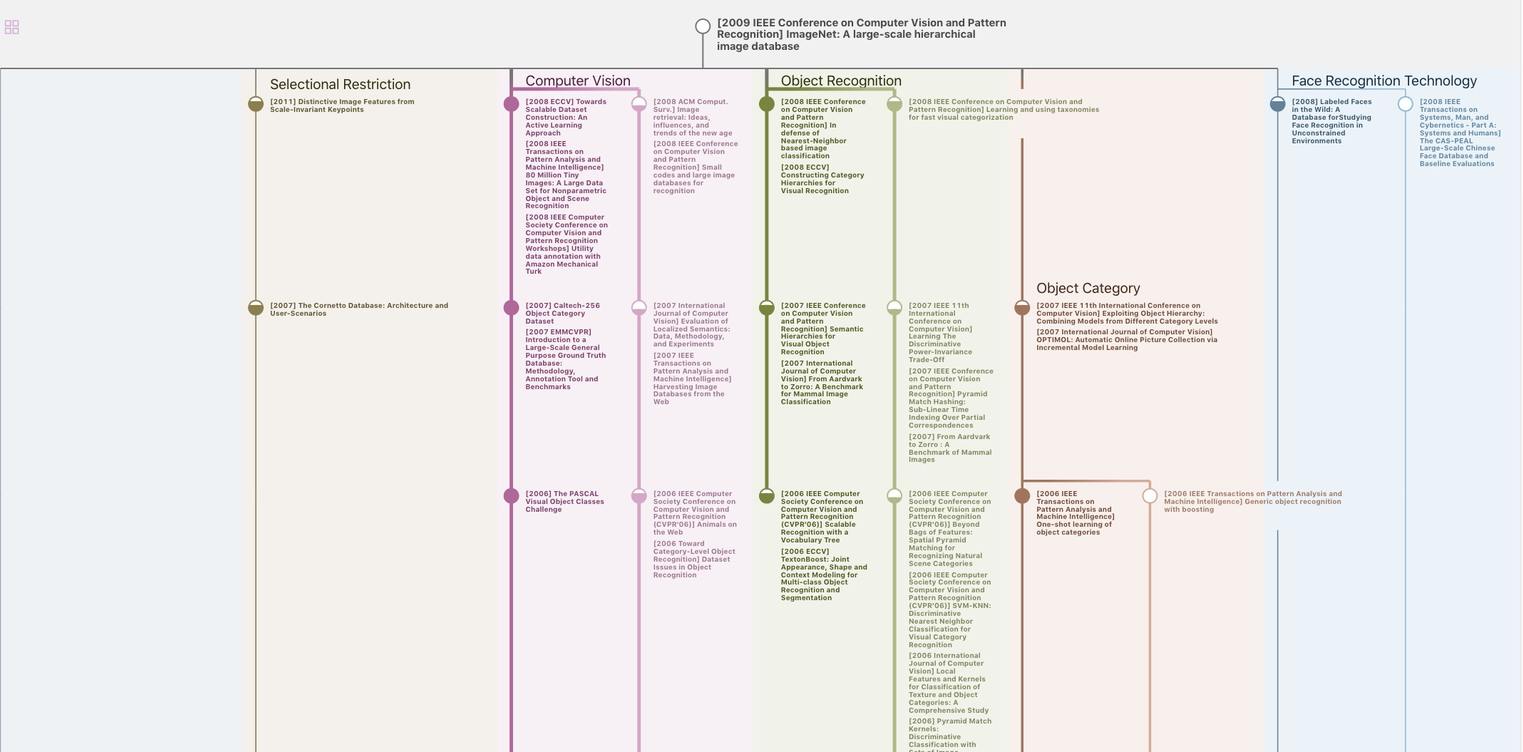
生成溯源树,研究论文发展脉络
Chat Paper
正在生成论文摘要