Distributed Online Gradient Boosting On Data Stream Over Multi-Agent Networks
SIGNAL PROCESSING(2021)
Abstract
In this paper, we study gradient boosting with distributed data streams over multi-agent networks, and propose a distributed online gradient boosting algorithm. Considering limited communication resources and privacy, each node aims to track the minimum of a global, time-varying cost function based on its own data stream and some information of neighbors. We first formulate the global cost function as a sum of local ones, and then convert distributed online gradient boosting into a distributed online optimization problem. At each time step, the local model is updated by a gradient descent step based on the current data, followed by a consensus step with the neighbors. Then, we use a dynamic regret to measure the performance of the proposed algorithm, and prove that the regret has an O(T) bound. Simulations with some practical datasets illustrate the performance of the proposed algorithm. (c) 2021 Elsevier B.V. All rights reserved.
MoreTranslated text
Key words
Data stream, Multi-agent networks, Online supervised learning, Online gradient boosting, Distributed online optimization
AI Read Science
Must-Reading Tree
Example
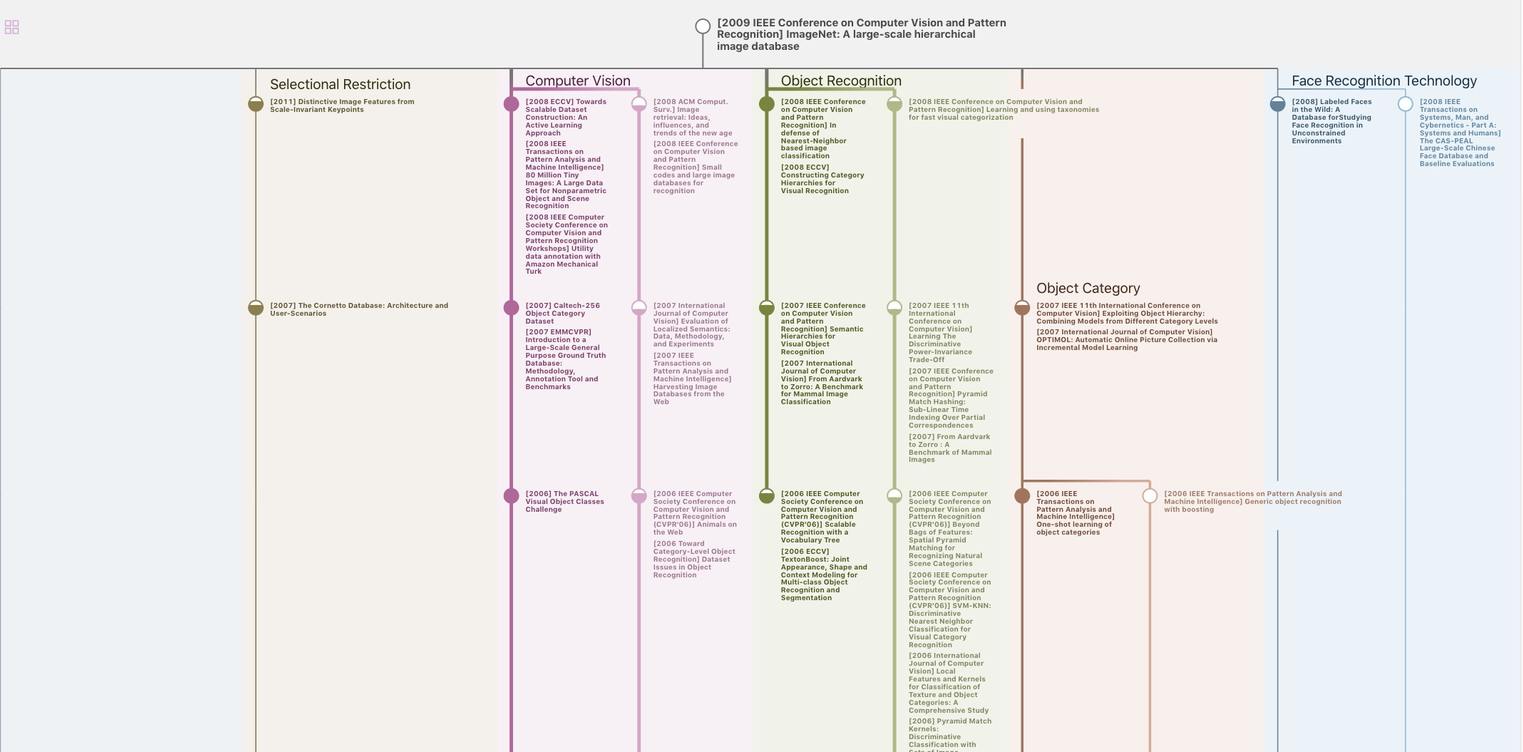
Generate MRT to find the research sequence of this paper
Chat Paper
Summary is being generated by the instructions you defined