Computing Semilinear Sparse Models for Approximately Eventually Periodic Signals
IFAC-PapersOnLine(2022)
摘要
Some elements of the theory and algorithmics corresponding to the computation of semilinear sparse models for discrete-time signals are presented. In this study, we will focus on approximately eventually periodic discrete-time signals, that is, signals that can exhibit an aperiodic behavior for an initial amount of time, and then become approximately periodic afterwards. The semilinear models considered in this study are obtained by combining sparse representation methods, linear autoregressive models and GRU neural network models. Firstly, each block model is fitted independently, using some reference data corresponding to some signal under consideration. Secondly, some mixing parameters are fitted, in order to obtain a signal model consisting of a linear combination of the previously fitted blocks, using the aforementioned reference data. Along the process, sparse representations of some of the matrices of parameters of the resulting model are computed. Some prototypical computational implementations are presented as well.
更多查看译文
关键词
Autoregressive models,neural-network models,parameter identification,least-squares approximation,time-series analysis
AI 理解论文
溯源树
样例
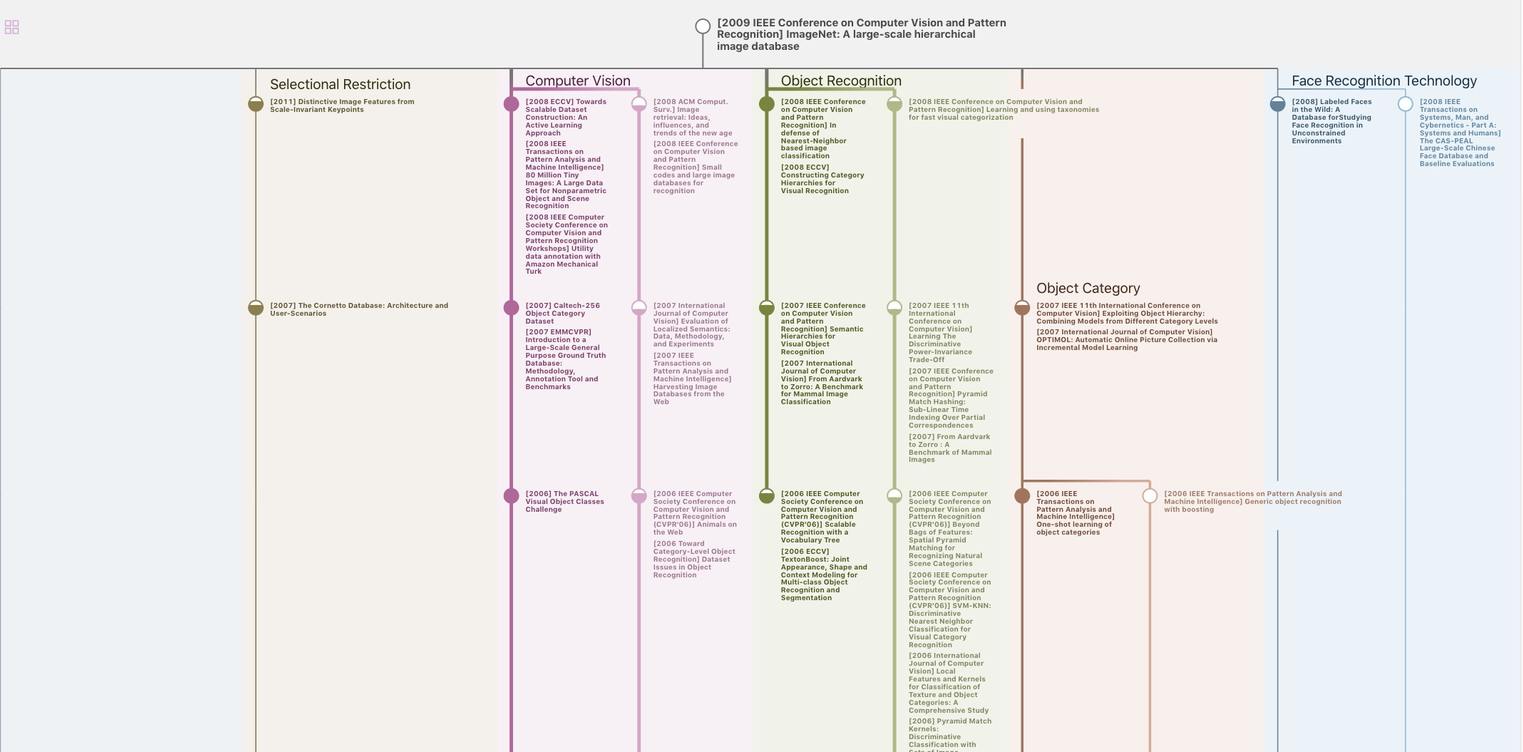
生成溯源树,研究论文发展脉络
Chat Paper
正在生成论文摘要