Nonlinear Transform Induced Tensor Nuclear Norm for Tensor Completion
Journal of Scientific Computing(2022)
摘要
The linear transform-based tensor nuclear norm (TNN) methods have recently obtained promising results for tensor completion. The main idea of these methods is exploiting the low-rank structure of frontal slices of the targeted tensor under the linear transform along the third mode. However, the low-rankness of frontal slices is not significant under the linear transforms family. To better pursue the low-rank approximation, we propose a nonlinear transform-based TNN (NTTNN). More concretely, the proposed nonlinear transform is a composite transform consisting of the linear semi-orthogonal transform along the third mode and the element-wise nonlinear transform on frontal slices of the tensor under the linear semi-orthogonal transform. The two transforms in the composite transform are indispensable and complementary to fully exploit the underlying low-rankness. Based on the suggested low-rankness metric, i.e., NTTNN, we propose a low-rank tensor completion model. To tackle the resulting nonlinear and nonconvex optimization model, we elaborately design a proximal alternating minimization algorithm and establish the theoretical convergence guarantee. Extensive experimental results on hyperspectral images, multispectral images, and videos show that our method outperforms the linear transform-based state-of-the-art LRTC methods in terms of PSNR, SSIM, SAM values and visual quality.
更多查看译文
关键词
Nonlinear transform,Tensor nuclear norm,Proximal alternating minimization,Tensor completion.
AI 理解论文
溯源树
样例
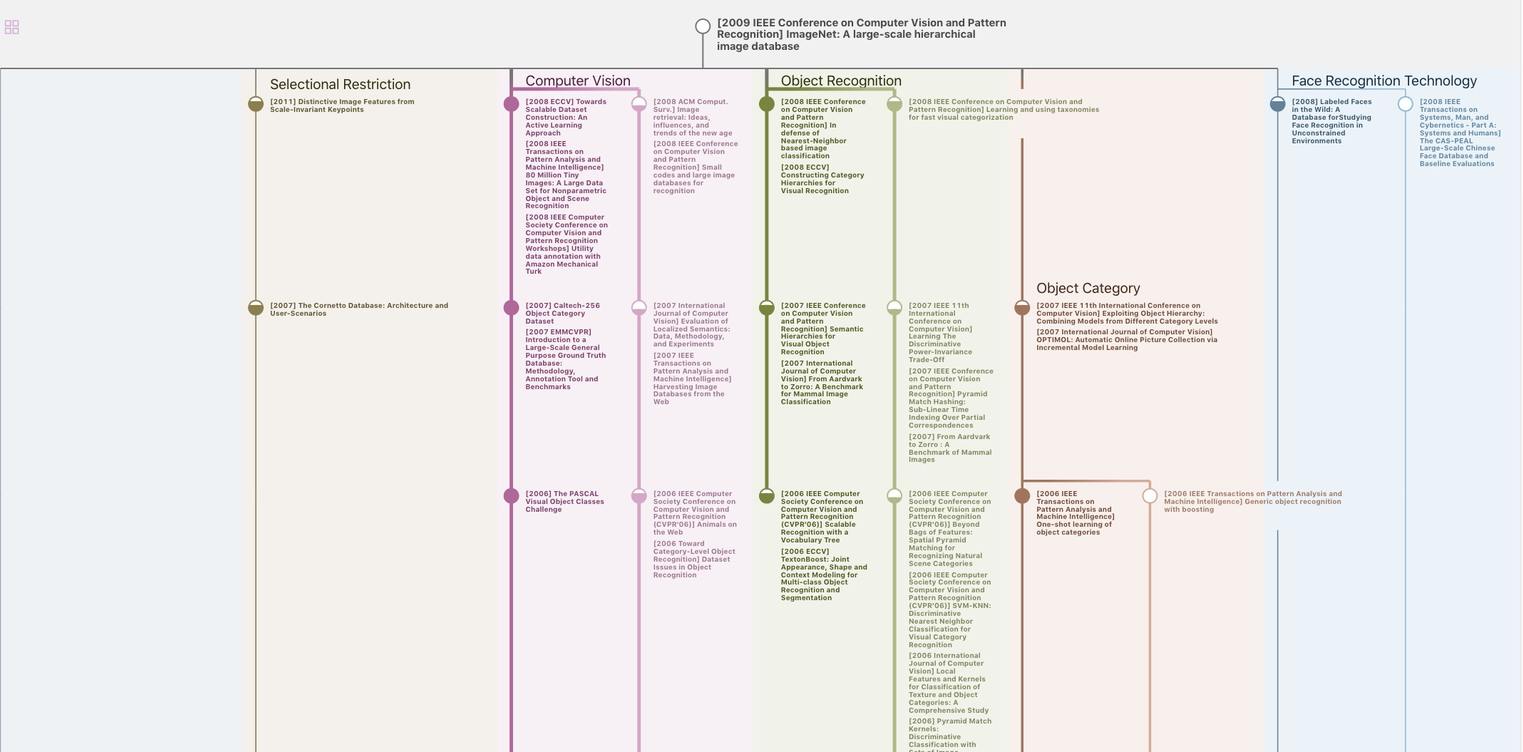
生成溯源树,研究论文发展脉络
Chat Paper
正在生成论文摘要