BBQ: A Hand-Built Bias Benchmark for Question Answering
Findings of the Association for Computational Linguistics ACL 2022(2022)
关键词
Topic Modeling,Language Modeling
AI 理解论文
溯源树
样例
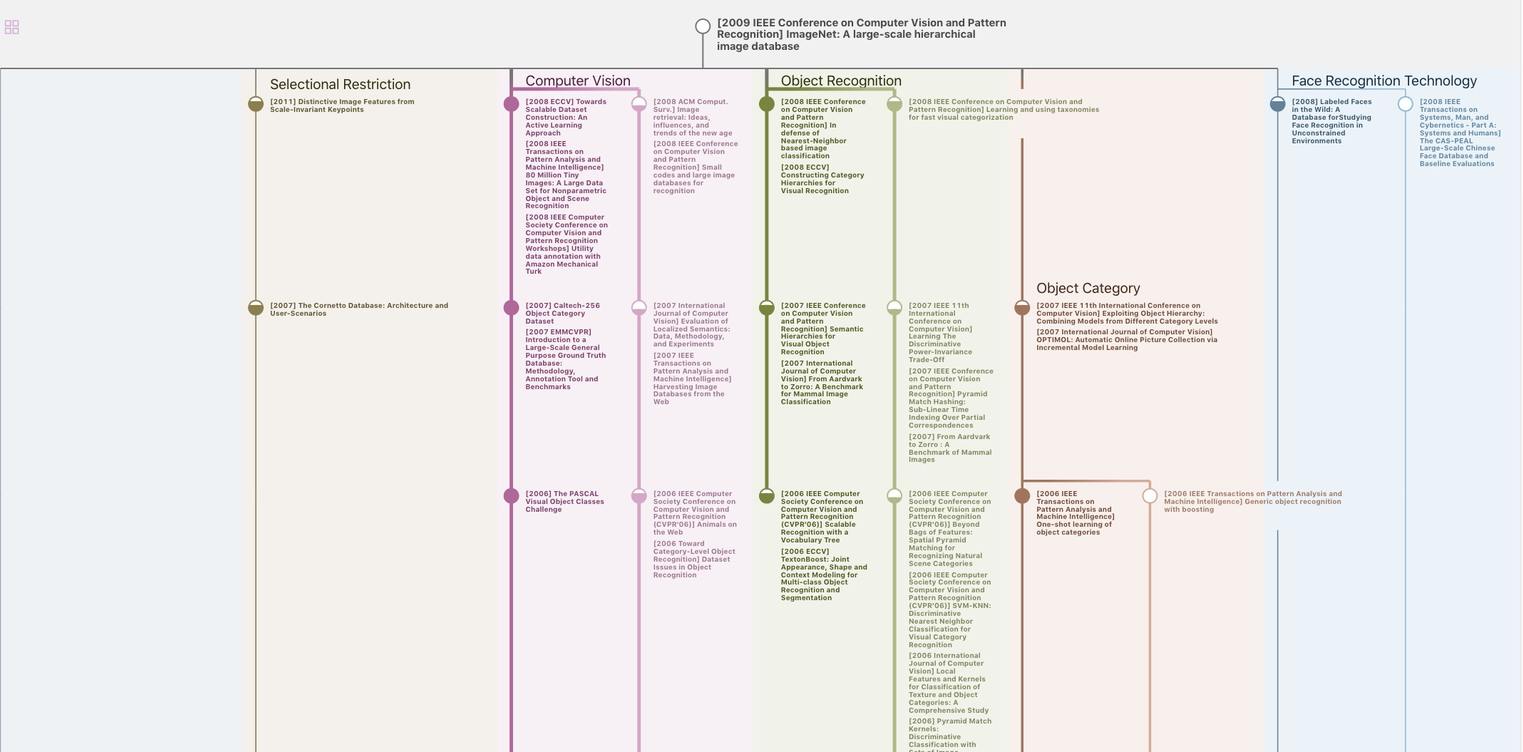
生成溯源树,研究论文发展脉络
Chat Paper
正在生成论文摘要
Findings of the Association for Computational Linguistics ACL 2022(2022)