Towards Better Plasticity-Stability Trade-off in Incremental Learning: A Simple Linear Connector
2022 IEEE/CVF Conference on Computer Vision and Pattern Recognition (CVPR)(2022)
摘要
Plasticity-stability dilemma is a main problem for incremental learning, where plasticity is referring to the ability to learn new knowledge, and stability retains the knowledge of previous tasks. Many methods tackle this problem by storing previous samples, while in some applications, training data from previous tasks cannot be legally stored. In this work, we propose to employ mode connectivity in loss landscapes to achieve better plasticity-stability trade-off without any previous samples. We give an analysis of why and how to connect two independently optimized optima of networks, null-space projection for previous tasks and simple SGD for the current task, can attain a meaningful balance between preserving already learned knowledge and granting sufficient flexibility for learning a new task. This analysis of mode connectivity also provides us a new perspective and technology to control the trade-off between plasticity and stability. We evaluate the proposed method on several benchmark datasets. The results indicate our simple method can achieve notable improvement, and perform well on both the past and current tasks. On 10-split-CIFAR-100 task, our method achieves 79.79% accuracy, which is 6.02% higher. Our method also achieves 6.33% higher accuracy on TinyImageNet. Code is available at https://github.com/lingl1024/Connector.
更多查看译文
关键词
Machine learning, Deep learning architectures and techniques, Efficient learning and inferences, Recognition: detection,categorization,retrieval, Representation learning, Transfer/low-shot/long-tail learning
AI 理解论文
溯源树
样例
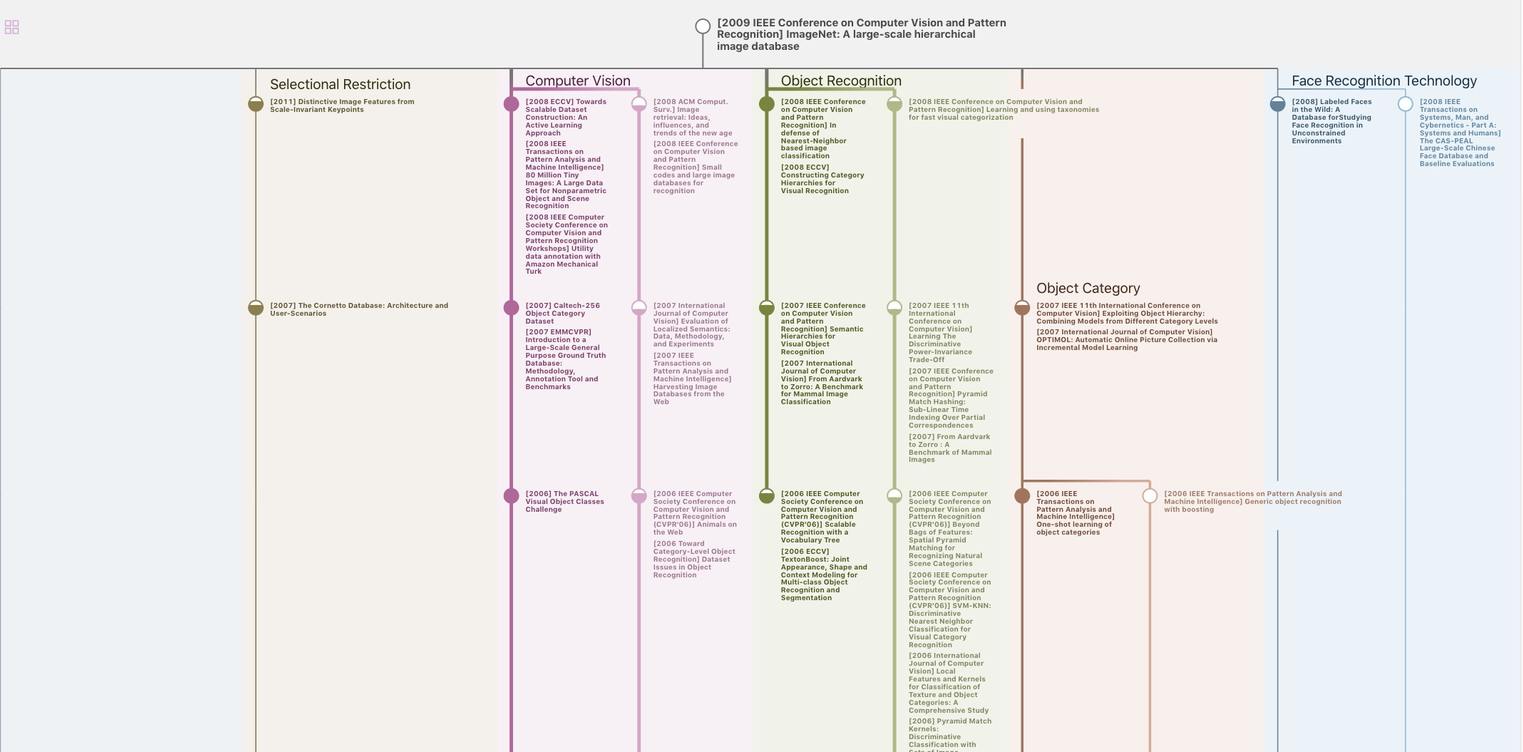
生成溯源树,研究论文发展脉络
Chat Paper
正在生成论文摘要