Learning mean-field equations from particle data using WSINDy
Physica D: Nonlinear Phenomena(2022)
摘要
We develop a weak-form sparse identification method for interacting particle systems (IPS) with the primary goals of reducing computational complexity for large particle number N and offering robustness to either intrinsic or extrinsic noise. In particular, we use concepts from mean-field theory of IPS in combination with the weak-form sparse identification of nonlinear dynamics algorithm (WSINDy) to provide a fast and reliable system identification scheme for recovering the governing stochastic differential equations for an IPS when the number of particles per experiment N is on the order of several thousands and the number of experiments M is less than 100. This is in contrast to existing work showing that system identification for N less than 100 and M on the order of several thousand is feasible using strong-form methods. We prove that under some standard regularity assumptions the scheme converges with rate O(N−1/2) in the ordinary least squares setting and we demonstrate the convergence rate numerically on several systems in one and two spatial dimensions. Our examples include a canonical problem from homogenization theory (as a first step towards learning coarse-grained models), the dynamics of an attractive–repulsive swarm, and the IPS description of the parabolic–elliptic Keller–Segel model for chemotaxis. Code is available at https://github.com/MathBioCU/WSINDy_IPS.
更多查看译文
关键词
Data-driven modeling,Interacting particle systems,Weak form,Mean-field limit,Sparse regression
AI 理解论文
溯源树
样例
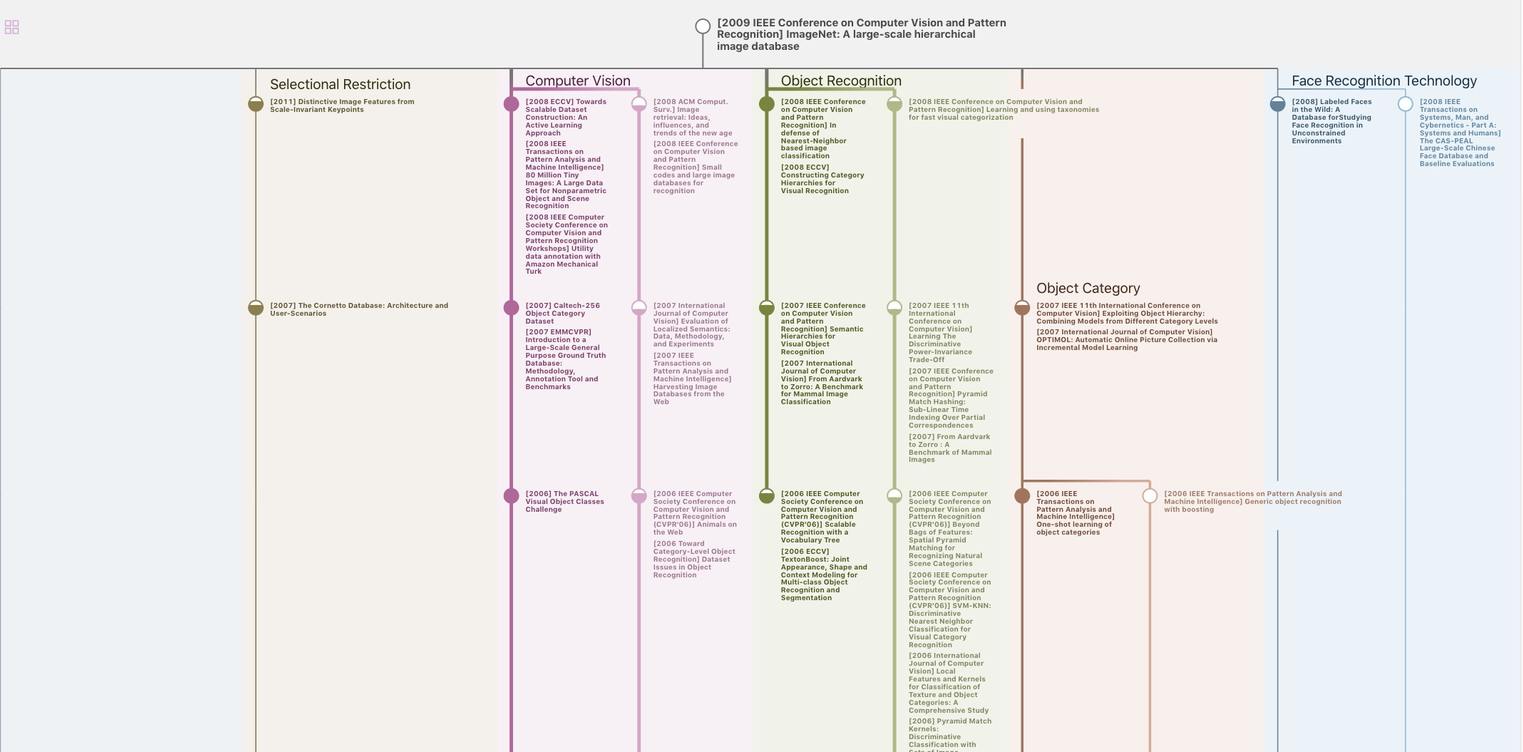
生成溯源树,研究论文发展脉络
Chat Paper
正在生成论文摘要