Adversarial Attack across Datasets
arxiv(2022)
摘要
Existing transfer attack methods commonly assume that the attacker knows the training set (e.g., the label set, the input size) of the black-box victim models, which is usually unrealistic because in some cases the attacker cannot know this information. In this paper, we define a Generalized Transferable Attack (GTA) problem where the attacker doesn't know this information and is acquired to attack any randomly encountered images that may come from unknown datasets. To solve the GTA problem, we propose a novel Image Classification Eraser (ICE) that trains a particular attacker to erase classification information of any images from arbitrary datasets. Experiments on several datasets demonstrate that ICE greatly outperforms existing transfer attacks on GTA, and show that ICE uses similar texture-like noises to perturb different images from different datasets. Moreover, fast fourier transformation analysis indicates that the main components in each ICE noise are three sine waves for the R, G, and B image channels. Inspired by this interesting finding, we then design a novel Sine Attack (SA) method to optimize the three sine waves. Experiments show that SA performs comparably to ICE, indicating that the three sine waves are effective and enough to break DNNs under the GTA setting.
更多查看译文
关键词
datasets
AI 理解论文
溯源树
样例
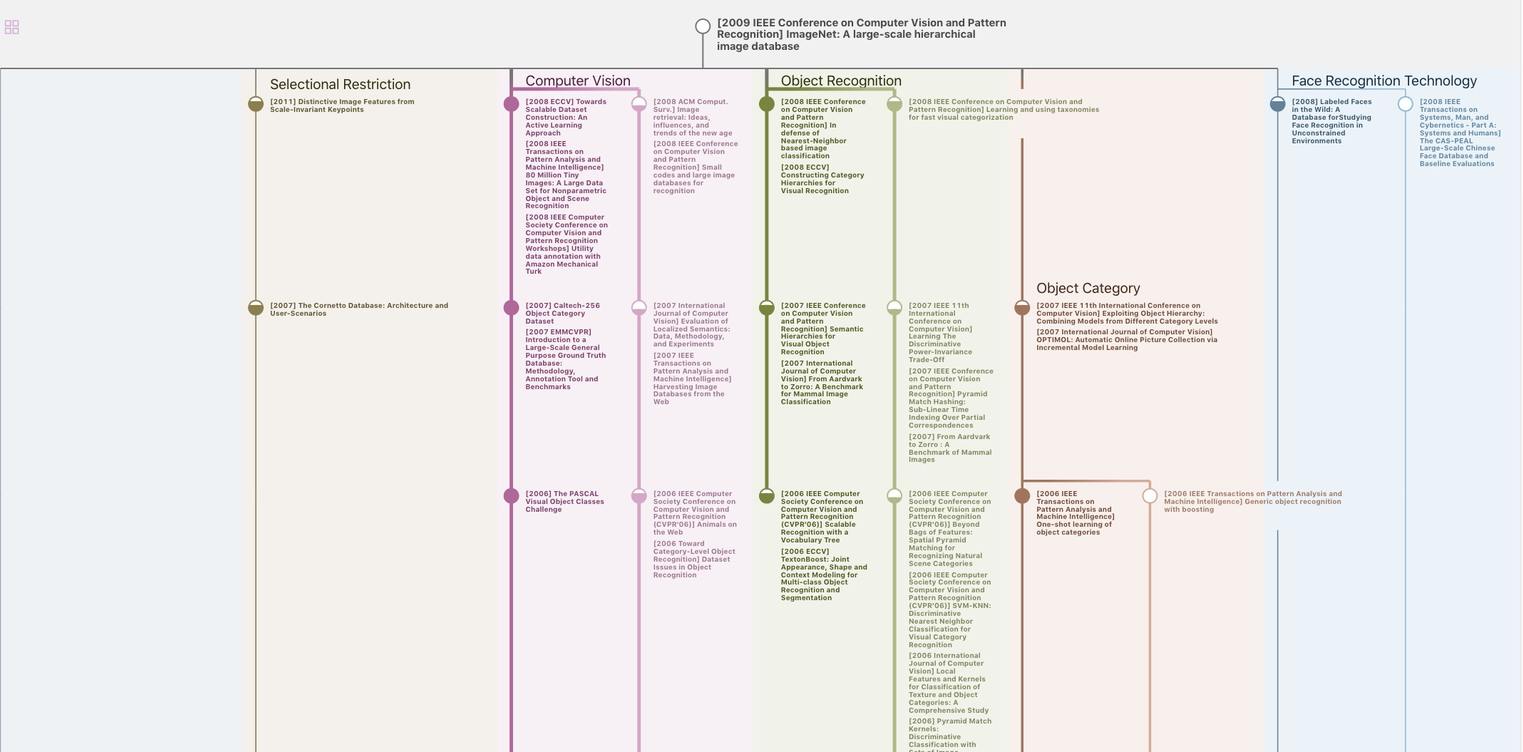
生成溯源树,研究论文发展脉络
Chat Paper
正在生成论文摘要