Graph-Theoretic Post-Processing Of Segmentation With Application To Dense Biofilms
IEEE TRANSACTIONS ON IMAGE PROCESSING(2021)
摘要
Recent deep learning methods have provided successful initial segmentation results for generalized cell segmentation in microscopy. However, for dense arrangements of small cells with limited ground truth for training, the deep learning methods produce both over-segmentation and under-segmentation errors. Post-processing attempts to balance the trade-off between the global goal of cell counting for instance segmentation, and local fidelity to the morphology of identified cells. The need for post-processing is especially evident for segmenting 3D bacterial cells in densely-packed communities called biofilms. A graph-based recursive clustering approach, m-LCuts, is proposed to automatically detect collinearly structured clusters and applied to post-process unsolved cells in 3D bacterial biofilm segmentation. Construction of outlier-removed graphs to extract the collinearity feature in the data adds additional novelty to m-LCuts. The superiority of m-LCuts is observed by the evaluation in cell counting with over 90% of cells correctly identified, while a lower bound of 0.8 in terms of average single-cell segmentation accuracy is maintained. This proposed method does not need manual specification of the number of cells to be segmented. Furthermore, the broad adaptation for working on various applications, with the presence of data collinearity, also makes m-LCuts stand out from the other approaches.
更多查看译文
关键词
Multi-dimensional, data clustering, graph cut, post-processing, cell segmentation
AI 理解论文
溯源树
样例
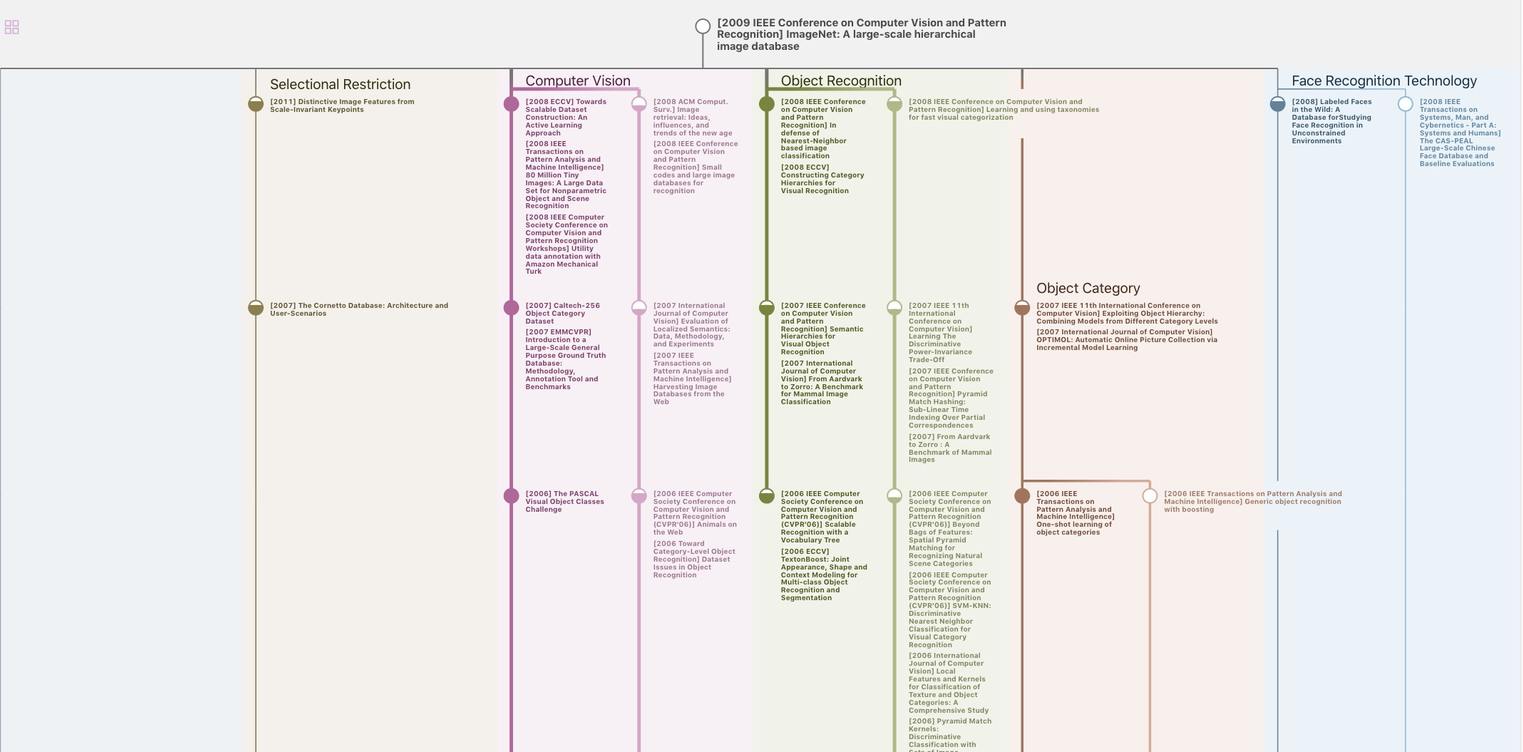
生成溯源树,研究论文发展脉络
Chat Paper
正在生成论文摘要