Sentence-State LSTMs For Sequence-to-Sequence Learning.
NLPCC(2021)
摘要
Transformer is currently the dominant method for sequence to sequence problems. In contrast, RNNs have become less popular due to the lack of parallelization capabilities and the relatively lower performance. In this paper, we propose to use a parallelizable variant of bi-directional LSTMs (BiLSTMs), namely sentence-state LSTMs (S-LSTM), as an encoder for sequence-to-sequence tasks. The complexity of S-LSTM is only O ( n ) as compared to O ( n 2 ) of Transformer. On four neural machine translation benchmarks, we empirically find that S-SLTM can achieve significantly better performances than BiLSTM and convolutional neural networks (CNNs). When compared to Transformer, our model gives competitive performance while being 1.6 times faster during inference.
更多查看译文
关键词
Neural machine translation, Sentence-State LSTMs, Bi-directional LSTMs, CNN, Transformers
AI 理解论文
溯源树
样例
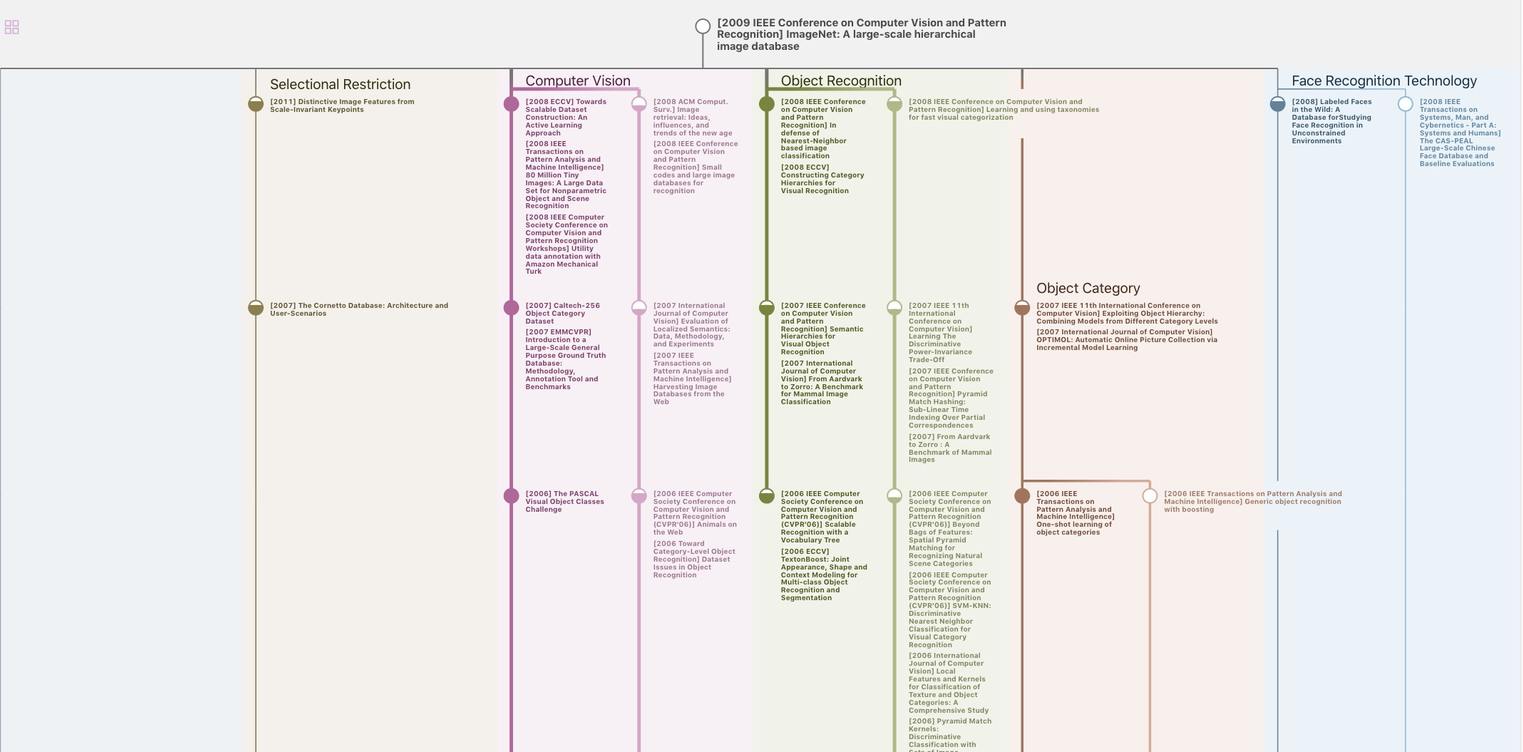
生成溯源树,研究论文发展脉络
Chat Paper
正在生成论文摘要