Evaluation of Active Learning Techniques on Medical Image Classification with Unbalanced Data Distributions.
DGM4MICCAI/DALI@MICCAI(2021)
摘要
In supervised image classification, convolutional deep neural networks have become the dominant methodology showing excellent performance in a number of tasks. These models typically require a very large number of labelled data samples to achieve required performance and generalisability. While data acquisition is relatively easy, data labelling, particularly in the case of medical imaging where expertise is required, is expensive. This has led to the investigation of active learning methods to improve the effectiveness of choosing which data should be prioritised for labelling. While new algorithms and methodologies continue to be introduced for active learning, each reporting improved performance, one key aspect that can be overlooked is the underlying data distribution of the dataset. Many active learning papers are bench-marked using curated datasets with balanced class distributions. This is not representative of many real-world scenarios where the data acquired can be heavily skewed towards a certain class. In this paper, we evaluate the performance of several established active learning techniques on an unbalanced dataset of 15153 chest X-Ray images, forming a more realistic scenario. This paper shows that the unbalanced dataset has a significant impact on the performance of certain algorithms, and should be considered when choosing which active learning strategy to implement.
更多查看译文
关键词
active learning techniques,medical image classification
AI 理解论文
溯源树
样例
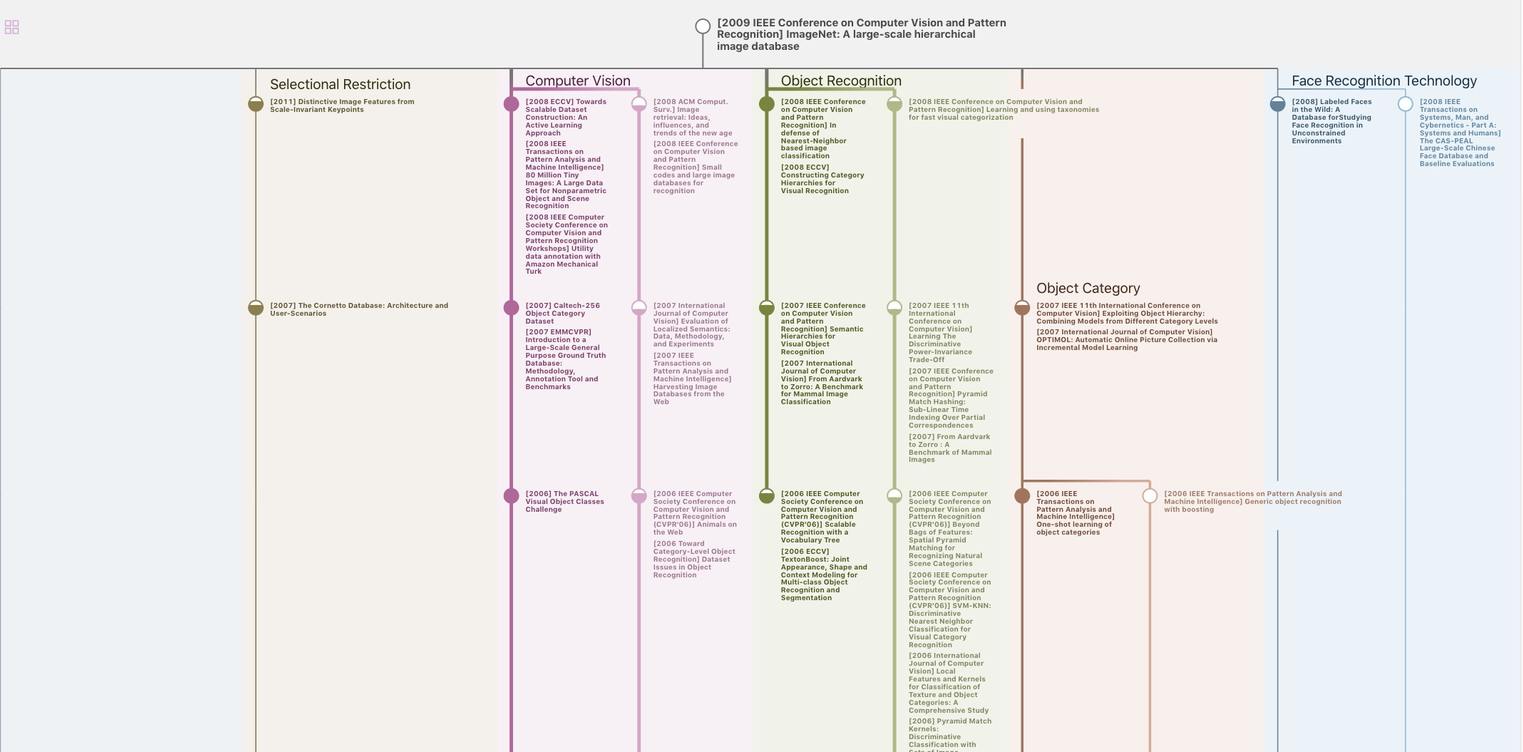
生成溯源树,研究论文发展脉络
Chat Paper
正在生成论文摘要