Efficient Image Registration Network for Non-Rigid Cardiac Motion Estimation.
MLMIR@MICCAI(2021)
摘要
Cardiac motion estimation plays an essential role in motion-compensated cardiac Magnetic Resonance (MR) image reconstruction. In this work, we propose a robust and lightweight self-supervised deep learning registration framework, termed MRAFT, to estimate non-rigid cardiac motion. The proposed framework combines an efficient architecture with a novel degradation-restoration (DR) loss term, and an enhancement mask derived from a pre-trained segmentation network. This framework enables the prediction of both small and large cardiac motion more precisely, and allows us to handle through-plane motion in a 2D registration setting via the DR loss. The quantitative and qualitative experiments on a retrospective cohort of 42 in-house acquired 2D cardiac CINE MRIs indicate that the proposed method outperforms the competing approaches substantially, with more than 25% reduction in residual photometric error, and up to 100x faster inference speed compared to conventional methods.
更多查看译文
关键词
efficient image registration network,motion,non-rigid
AI 理解论文
溯源树
样例
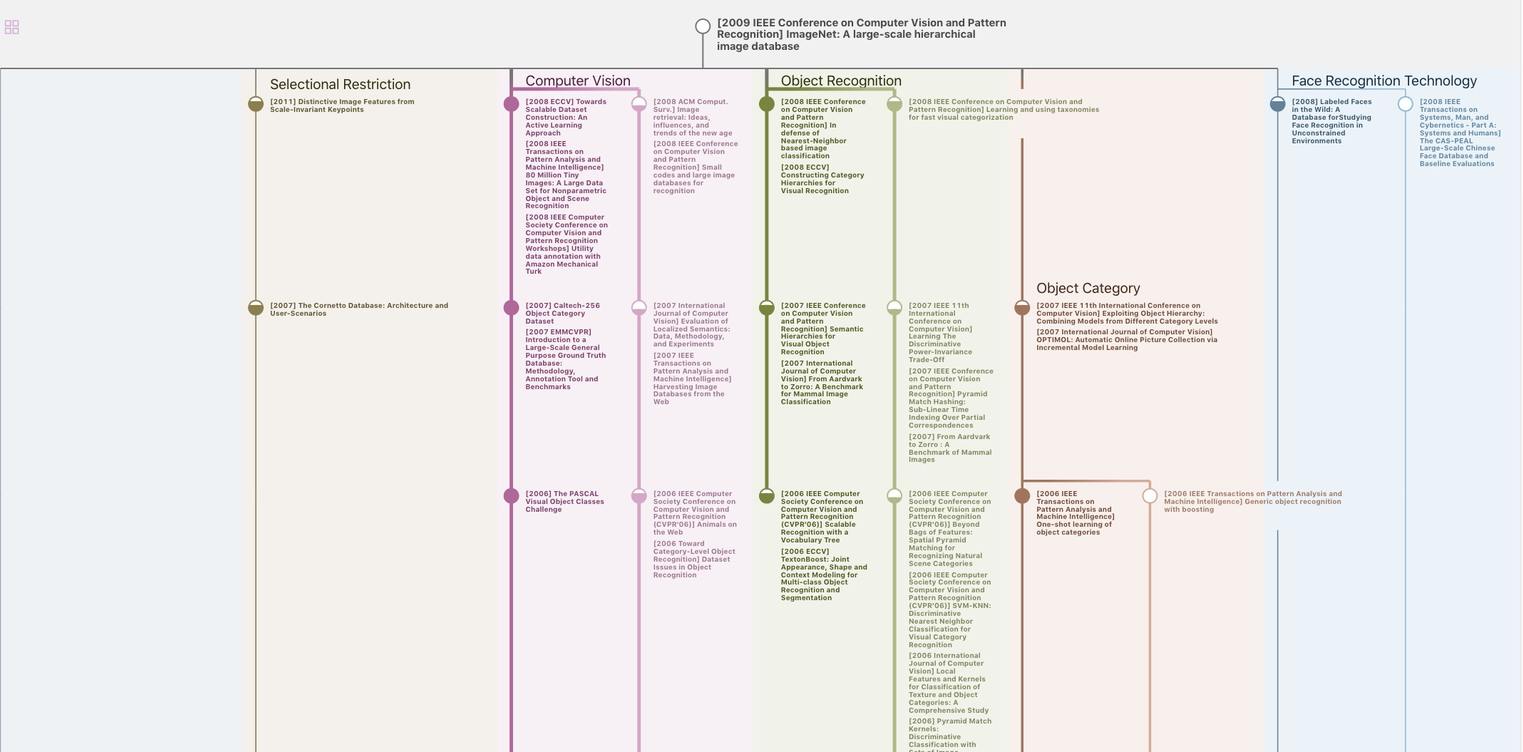
生成溯源树,研究论文发展脉络
Chat Paper
正在生成论文摘要