InSAR Displacement Time Series Mining - A Machine Learning Approach.
IGARSS(2021)
摘要
Interferometric Synthetic Aperture Radar (InSAR)-derived surface displacement time series enable a wide range of applications from urban structural monitoring to geohazard assessment. With systematic data acquisitions becoming the new norm for SAR missions, millions of time series are continuously generated. Machine Learning provides a framework for the efficient mining of such big data. Here, we focus on unsupervised mining of the data via clustering the similar temporal patterns and data-driven displacement signal reconstruction from the InSAR time series. We propose a deep Long Short Term Memory (LSTM) autoencoder model which can exploit temporal relations in contrast to the commonly used shallow learning methods, such as Uniform Manifold Approximation and Projection (UMAP). We also modify the loss function to allow the quantification of uncertainties in the time series data. The two approaches are applied to the Lazufre Volcanic Complex located at the central volcanic zone of the Andes and thereby compared.
更多查看译文
关键词
InSAR,Time Series,Unsupervised Learning,Latent Representation Learning,Autoencoders,Sequence Models,Deep Learning,Clustering
AI 理解论文
溯源树
样例
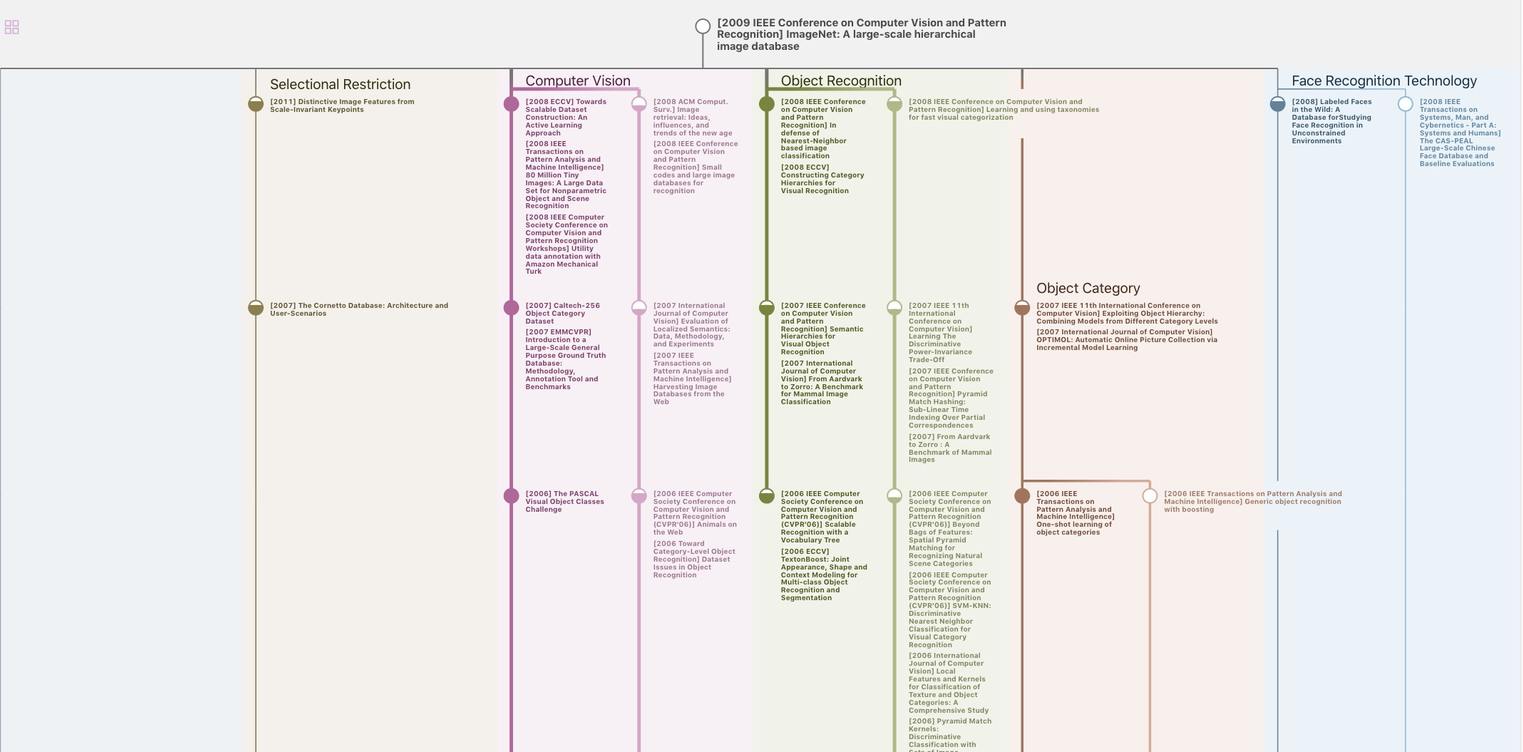
生成溯源树,研究论文发展脉络
Chat Paper
正在生成论文摘要