Retrieval-guided Counterfactual Generation for QA
PROCEEDINGS OF THE 60TH ANNUAL MEETING OF THE ASSOCIATION FOR COMPUTATIONAL LINGUISTICS (ACL 2022), VOL 1: (LONG PAPERS)(2022)
Abstract
Deep NLP models have been shown to be brittle to input perturbations. Recent work has shown that data augmentation using counterfactuals - i.e. minimally perturbed inputs - can help ameliorate this weakness. We focus on the task of creating counterfactuals for question answering, which presents unique challenges related to world knowledge, semantic diversity, and answerability. To address these challenges, we develop a Retrieve-Generate-Filter (RGF) technique to create counterfactual evaluation and training data with minimal human supervision. Using an open-domain QA framework and question generation model trained on original task data, we create counterfactuals that are fluent, semantically diverse, and automatically labeled. Data augmentation with RGF counterfactuals improves performance on out-of-domain and challenging evaluation sets over and above existing methods, in both the reading comprehension and open-domain QA settings. Moreover, we find that RGF data leads to significant improvements to robustness to local perturbations.(1)
MoreTranslated text
Key words
counterfactual generation,retrieval-guided
AI Read Science
Must-Reading Tree
Example
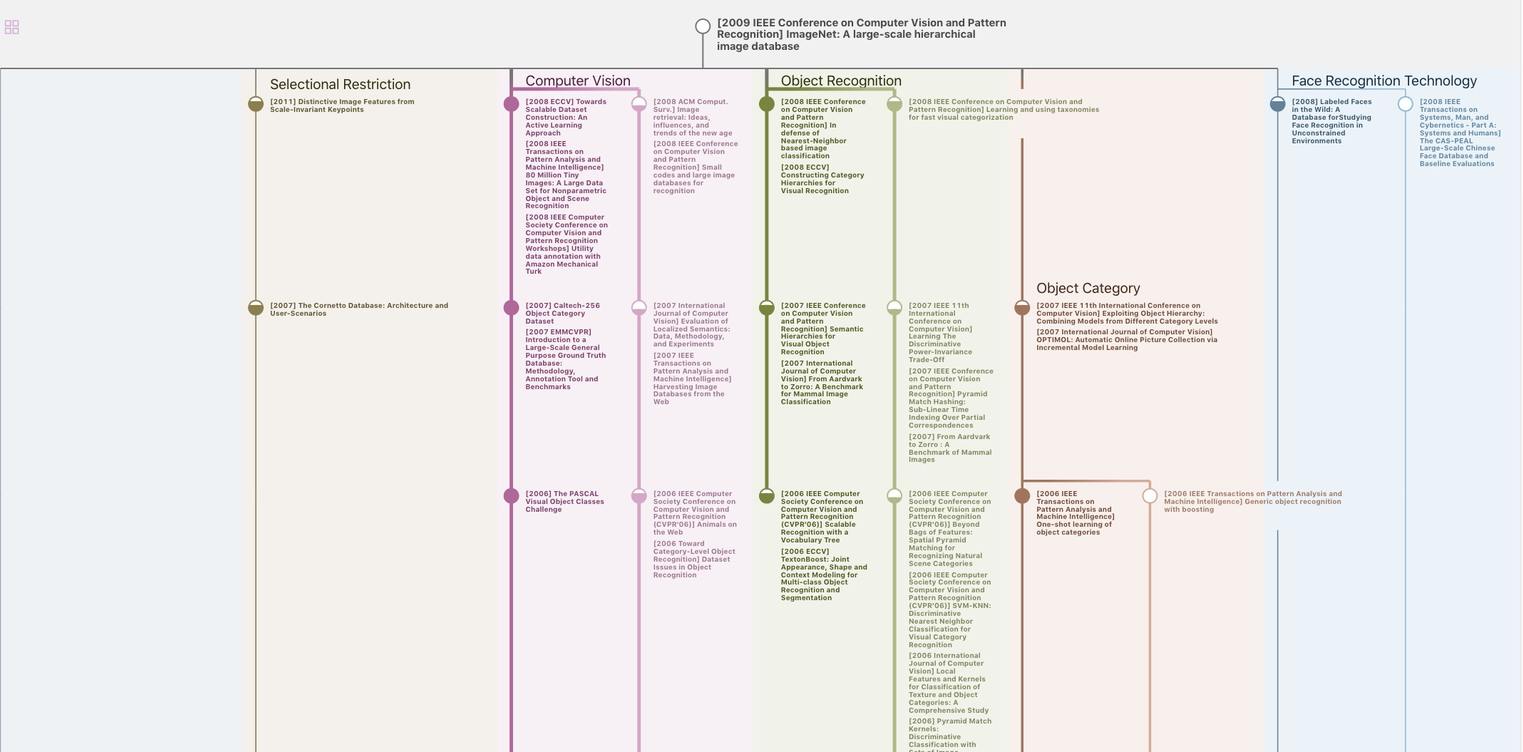
Generate MRT to find the research sequence of this paper
Chat Paper
Summary is being generated by the instructions you defined