Connection Management xAPP for O-RAN RIC - A Graph Neural Network and Reinforcement Learning Approach.
ICMLA(2021)
摘要
Connection management is an important problem for any wireless network to ensure smooth and well-balanced operation throughout. Traditional methods for connection management (specifically user-cell association) consider sub-optimal and greedy solutions such as connection of each user to a cell with maximum receive power. However, network performance can be improved by leveraging machine learning (ML) and artificial intelligence (AI) based solutions. The next generation software defined 5G networks defined by the Open Radio Access Network (O-RAN) alliance facilitates the inclusion of ML/AI based solutions for various network problems. In this paper, we consider intelligent connection management based on the O-RAN network architecture to optimize user association and load balancing in the network. We formulate connection management as a combinatorial graph optimization problem. We propose a deep reinforcement learning (DRL) solution that uses the underlying graph to learn the weights of the graph neural networks (GNN) for optimal user-cell association. We consider three candidate objective functions: sum user throughput, cell coverage, and load balancing. Our results show up to 10% gain in throughput, 45-140% gain cell coverage, 20-45% gain in load balancing depending on network deployment configurations compared to baseline greedy techniques.
更多查看译文
关键词
Open Radio Access Networks,RAN Intelligent Controller,Graph Neural Networks,Deep Reinforcement learning,Connection Management,xAPP
AI 理解论文
溯源树
样例
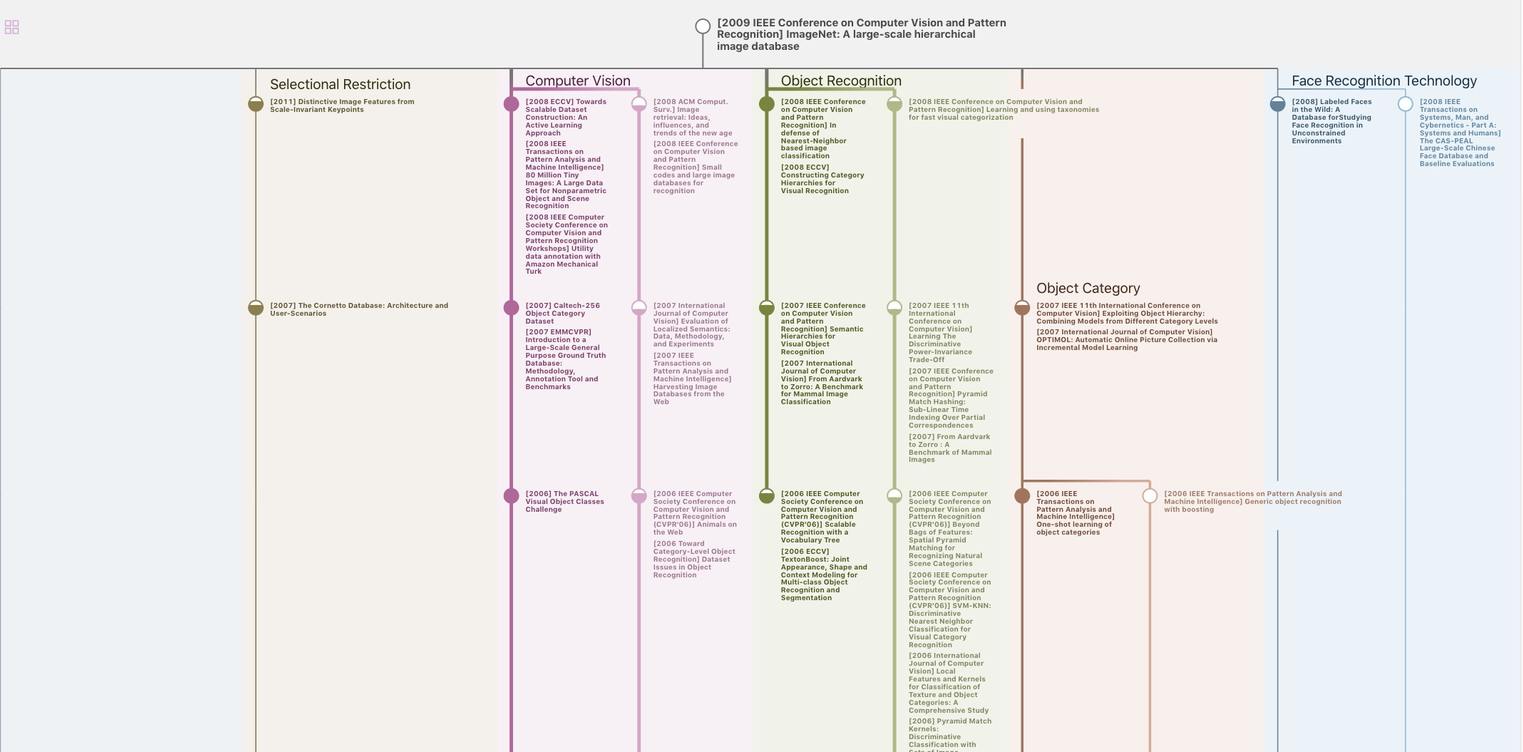
生成溯源树,研究论文发展脉络
Chat Paper
正在生成论文摘要