Protecting Data Privacy in Federated Learning Combining Differential Privacy and Weak Encryption.
SciSec(2021)
摘要
As a typical application of decentralization, federated learning prevents privacy leakage of crowdsourcing data for various training tasks. Instead of transmitting actual data, federated learning only updates model parameters of server by learning multiple sub-models from clients. However, these parameters may be leaked during transmission and further used by attackers to restore client data. Existing technologies used to protect parameters from privacy leakage do not achieve the sufficient protection of parameter information. In this paper, we propose a novel and efficient privacy protection method, which perturbs the privacy information contained in the parameters and completes its ciphertext representation in transmission. Regarding to the perturbation part, differential privacy is utilized to perturb the real parameters, which can minimize the privacy information contained in the parameters. To further camouflage the parameters, the weak encryption keeps the ciphertext form of the parameters as they are transmitted from the client to the server. As a result, neither the server nor any middle attacker can obtain the real information of the parameter directly. The experiments show that our method effectively resists attacks from both malicious clients and malicious server.
更多查看译文
关键词
Federated learning, Privacy, Differential privacy, Weak encryption.
AI 理解论文
溯源树
样例
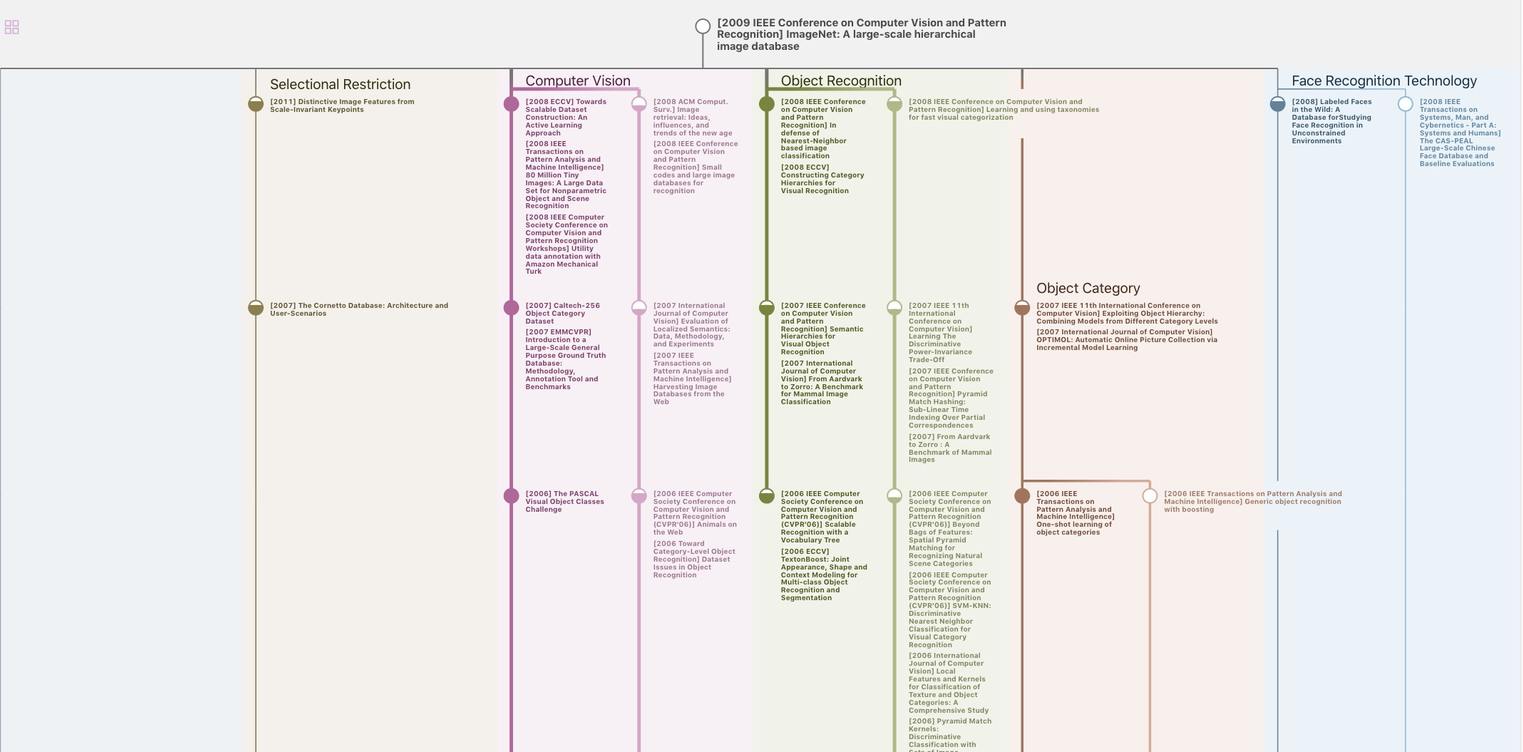
生成溯源树,研究论文发展脉络
Chat Paper
正在生成论文摘要