Coupling complementary strategy to flexible graph neural network for quick discovery of coformer in diverse co-crystal materials.
Nature communications(2021)
Abstract
Cocrystal engineering have been widely applied in pharmaceutical, chemistry and material fields. However, how to effectively choose coformer has been a challenging task on experiments. Here we develop a graph neural network (GNN) based deep learning framework to quickly predict formation of the cocrystal. In order to capture main driving force to crystallization from 6819 positive and 1052 negative samples reported by experiments, a feasible GNN framework is explored to integrate important prior knowledge into end-to-end learning on the molecular graph. The model is strongly validated against seven competitive models and three challenging independent test sets involving pharmaceutical cocrystals, π-π cocrystals and energetic cocrystals, exhibiting superior performance with accuracy higher than 96%, confirming its robustness and generalization. Furthermore, one new energetic cocrystal predicted is successfully synthesized, showcasing high potential of the model in practice. All the data and source codes are available at https://github.com/Saoge123/ccgnet for aiding cocrystal community.
MoreTranslated text
AI Read Science
Must-Reading Tree
Example
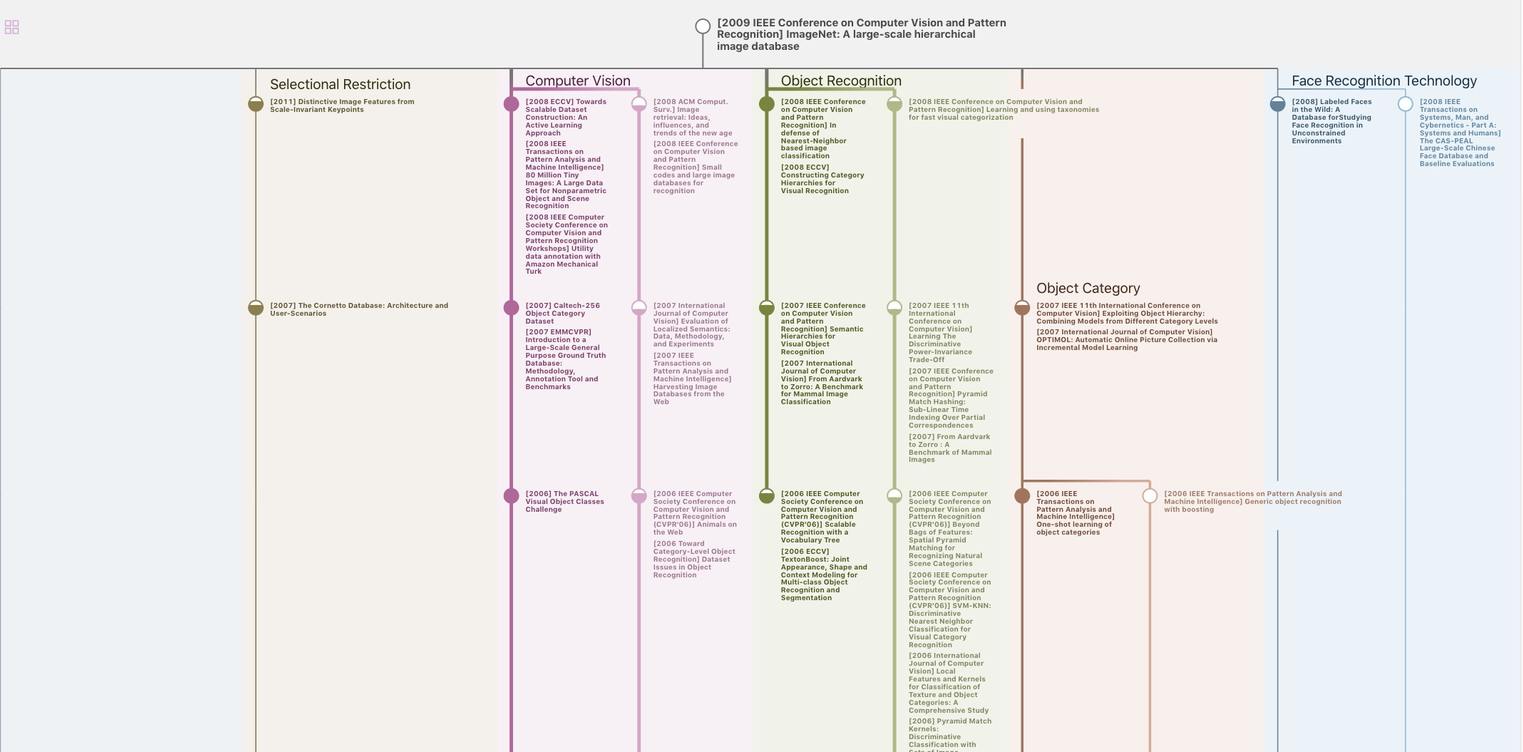
Generate MRT to find the research sequence of this paper
Chat Paper
Summary is being generated by the instructions you defined