Machine learning for percolation utilizing auxiliary Ising variables
PHYSICAL REVIEW E(2022)
摘要
Machine learning for phase transition has received intensive research interest in recent years. However, its application in percolation still remains challenging. We propose an auxiliary Ising mapping method for the machine learning study of the standard percolation as well as a variety of statistical mechanical systems in correlated percolation representation. We demonstrate that unsupervised machine learning is able to accurately locate the percolation threshold, independent of the spatial dimension of system or the type of phase transition, which can be first-order or continuous. Moreover, we show that, by neural network machine learning, auxiliary Ising configurations for different universalities can be classified with a high confidence level. Our results indicate that the auxiliary Ising mapping method, despite its simplicity, can advance the application of machine learning in statistical and condensed-matter physics.
更多查看译文
关键词
percolation,machine learning
AI 理解论文
溯源树
样例
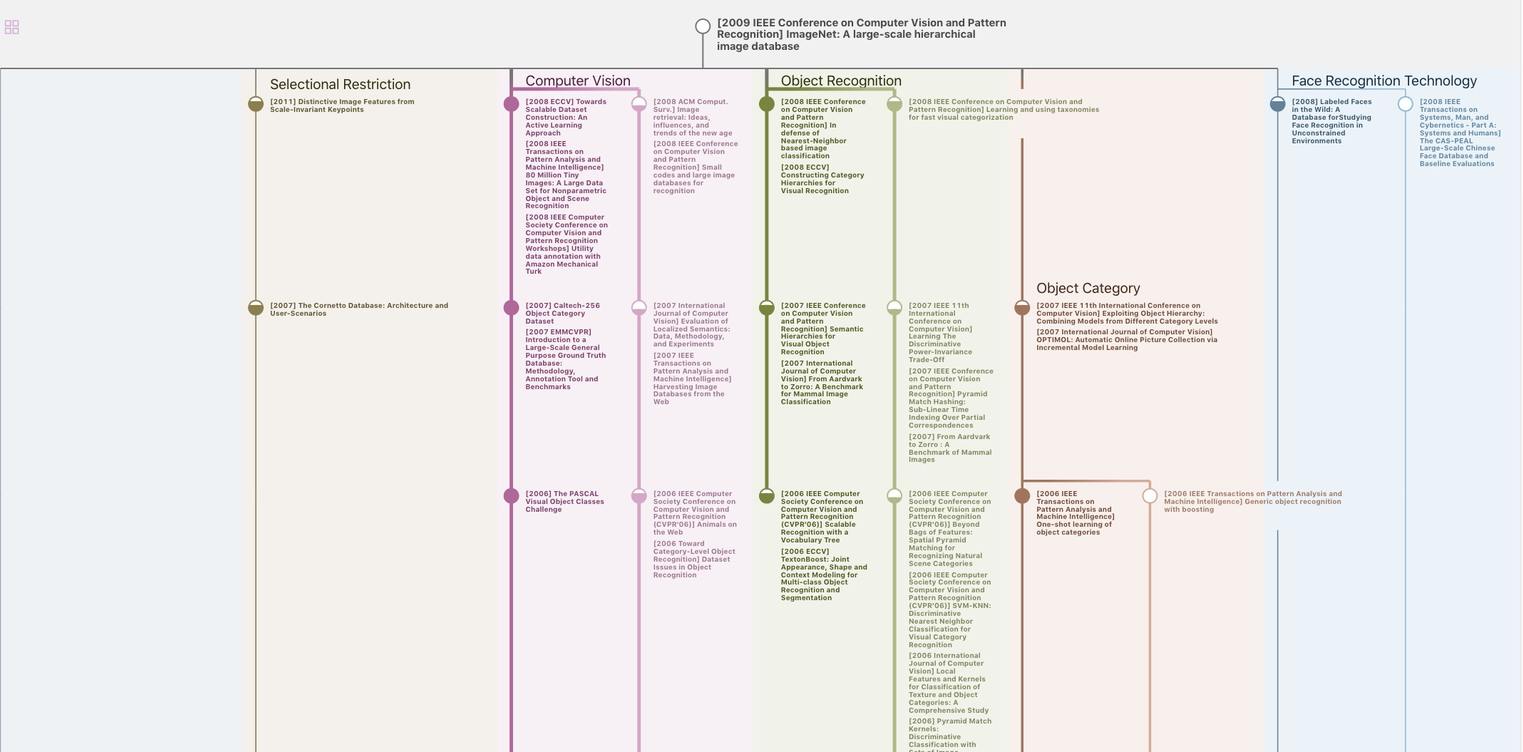
生成溯源树,研究论文发展脉络
Chat Paper
正在生成论文摘要