The springback penalty for robust signal recovery
APPLIED AND COMPUTATIONAL HARMONIC ANALYSIS(2022)
摘要
We propose a new penalty, the springback penalty, for constructing models to recover an unknown signal from incomplete and inaccurate measurements. Mathematically, the springback penalty is a weakly convex function. It bears various theoretical and computational advantages of both the benchmark convex l(1) penalty and many of its non-convex surrogates that have been well studied in the literature. We establish the exact and stable recovery theory for the recovery model using the springback penalty for both sparse and nearly sparse signals, respectively, and derive an easily implementable difference-of-convex algorithm. In particular, we show its theoretical superiority to some existing models with a sharper recovery bound for some scenarios where the level of measurement noise is large or the amount of measurements is limited. We also demonstrate its numerical robustness regardless of the varying coherence of the sensing matrix. The springback penalty is particularly favorable for the scenario where the incomplete and inaccurate measurements are collected by coherence-hidden or -static sensing hardware due to its theoretical guarantee of recovery with severe measurements, computational tractability, and numerical robustness for ill-conditioned sensing matrices. (C) 2022 Elsevier Inc. All rights reserved.
更多查看译文
关键词
Signal recovery, Compressed sensing, Penalty, Weakly convex, Difference-of-convex algorithm
AI 理解论文
溯源树
样例
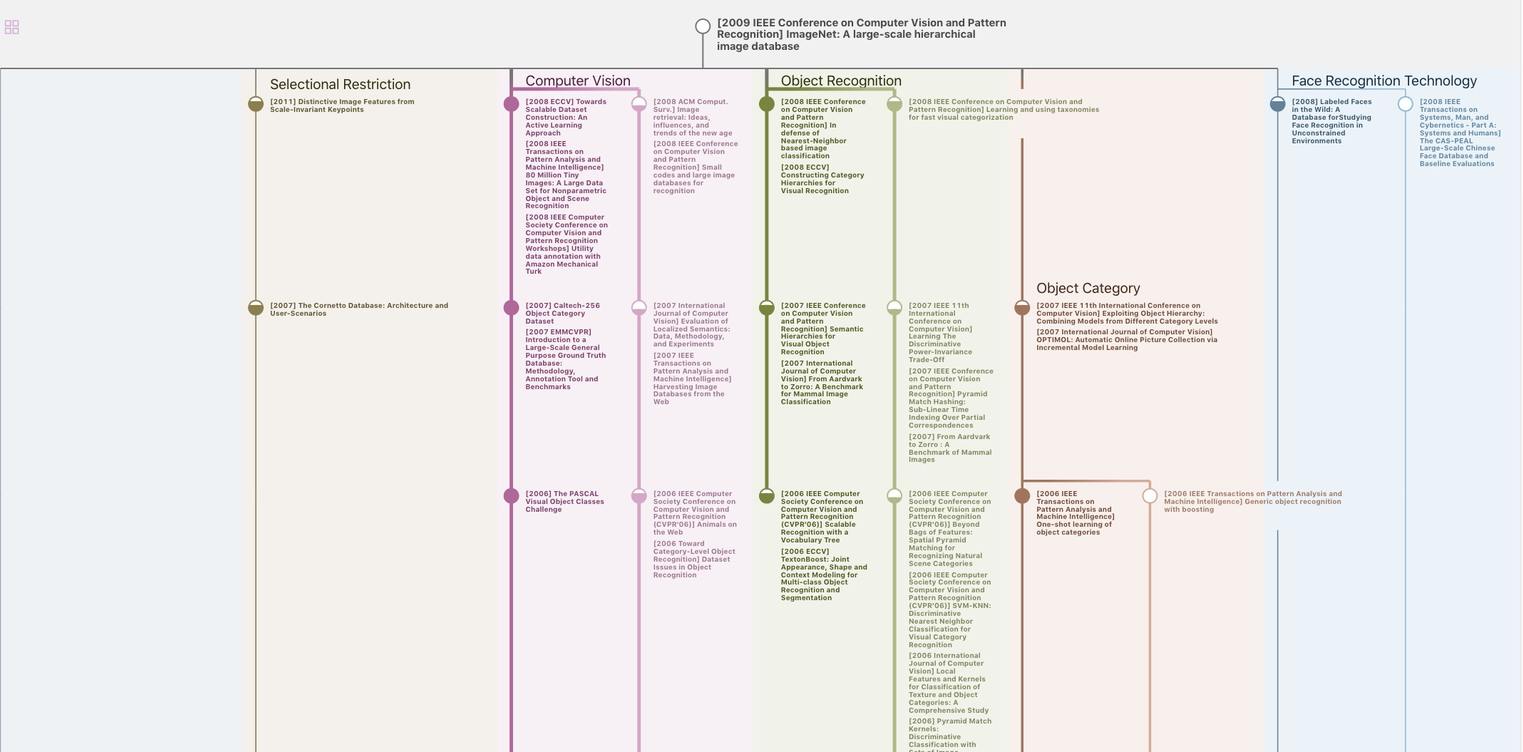
生成溯源树,研究论文发展脉络
Chat Paper
正在生成论文摘要