Estimation and Inference of Extremal Quantile Treatment Effects for Heavy-Tailed Distributions
JOURNAL OF THE AMERICAN STATISTICAL ASSOCIATION(2023)
摘要
Causal inference for extreme events has many potential applications in fields such as climate science, medicine and economics. We study the extremal quantile treatment effect of a binary treatment on a continuous, heavy-tailed outcome. Existing methods are limited to the case where the quantile of interest is within the range of the observations. For applications in risk assessment, however, the most relevant cases relate to extremal quantiles that go beyond the data range. We introduce an estimator of the extremal quantile treatment effect that relies on asymptotic tail approximation, and use a new causal Hill estimator for the extreme value indices of potential outcome distributions. We establish asymptotic normality of the estimators and propose a consistent variance estimator to achieve valid statistical inference. We illustrate the performance of our method in simulation studies, and apply it to a real data set to estimate the extremal quantile treatment effect of college education on wage.
更多查看译文
关键词
Treatment Effects,Causal Inference
AI 理解论文
溯源树
样例
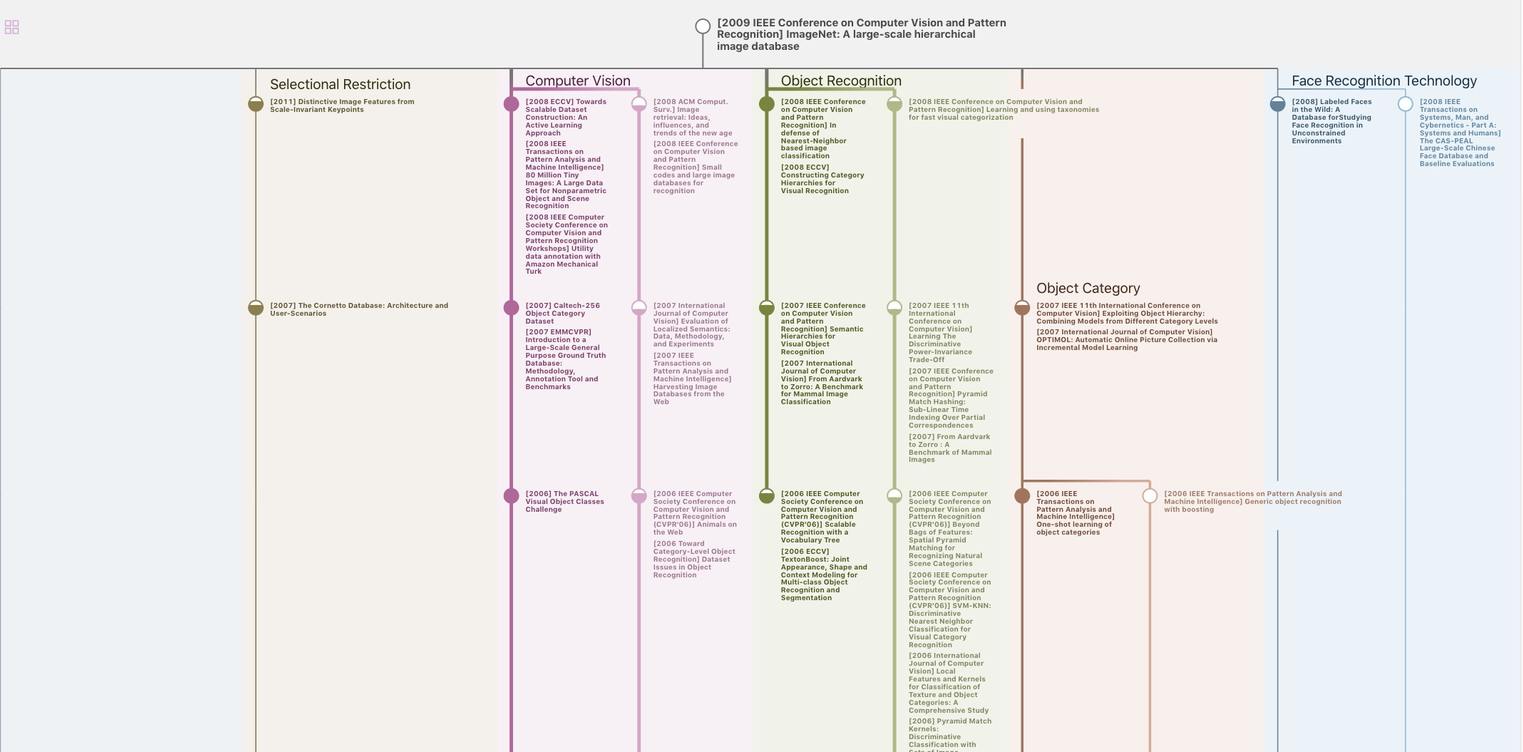
生成溯源树,研究论文发展脉络
Chat Paper
正在生成论文摘要