A Trust Crisis In Simulation-Based Inference? Your Posterior Approximations Can Be Unfaithful
arxiv(2022)
摘要
We present extensive empirical evidence showing that current Bayesian simulation-based inference algorithms can produce computationally unfaithful posterior approximations. Our results show that all benchmarked algorithms -- (Sequential) Neural Posterior Estimation, (Sequential) Neural Ratio Estimation, Sequential Neural Likelihood and variants of Approximate Bayesian Computation -- can yield overconfident posterior approximations, which makes them unreliable for scientific use cases and falsificationist inquiry. Failing to address this issue may reduce the range of applicability of simulation-based inference. For this reason, we argue that research efforts should be made towards theoretical and methodological developments of conservative approximate inference algorithms and present research directions towards this objective. In this regard, we show empirical evidence that ensembling posterior surrogates provides more reliable approximations and mitigates the issue.
更多查看译文
关键词
crisis,simulation-based
AI 理解论文
溯源树
样例
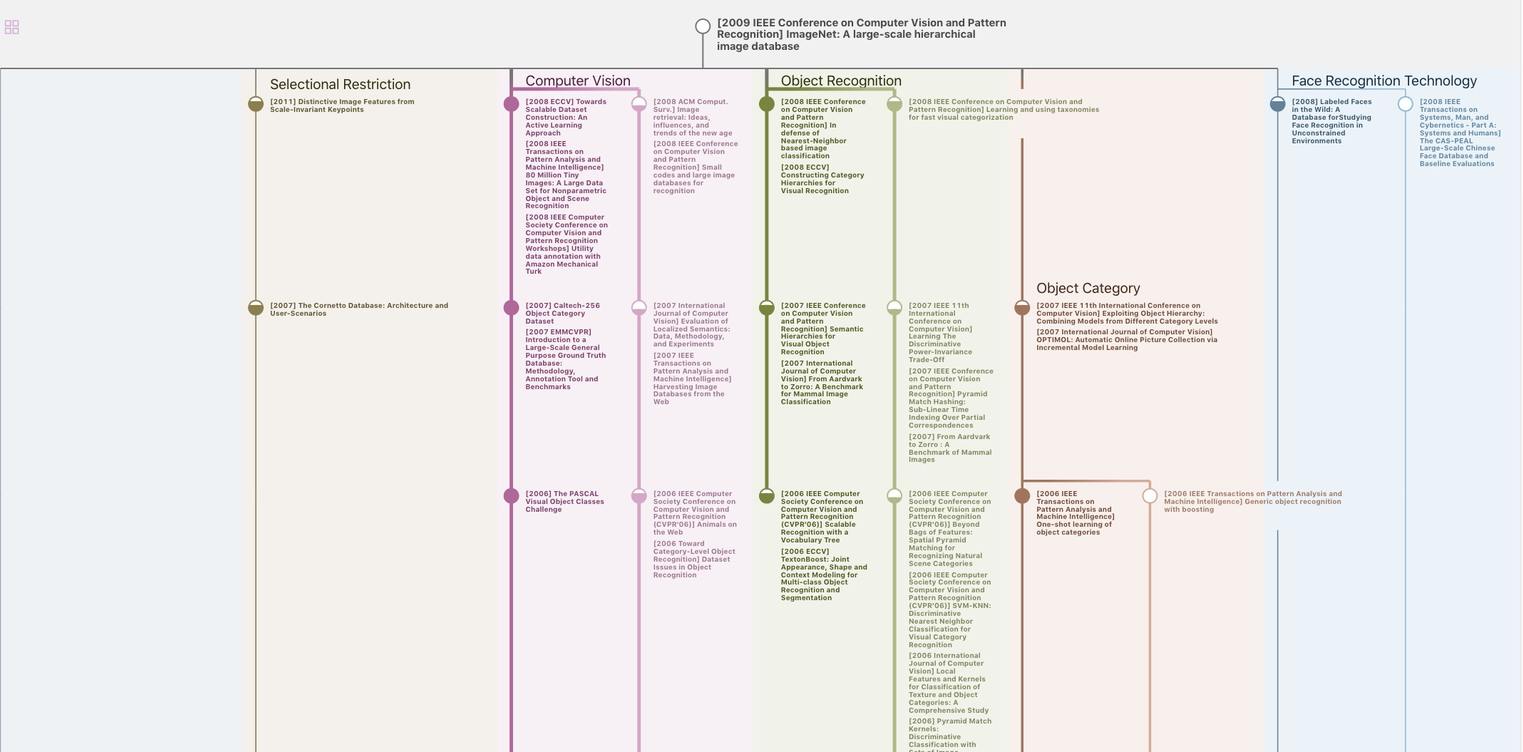
生成溯源树,研究论文发展脉络
Chat Paper
正在生成论文摘要