Data-Time Tradeoffs for Optimal k-Thresholding Algorithms in Compressed Sensing.
International Symposium on Information Theory (ISIT)(2022)
摘要
Optimal $k$-thresholding algorithms are a class of sparse signal recovery algorithms that overcome the shortcomings of traditional hard thresholding algorithms caused by the oscillation of the residual function. In this paper, we provide a novel theoretical analysis for the data-time tradeoffs of optimal $k$-thresholding algorithms. Both the analysis and numerical results demonstrate that when the number of measurements is small, the algorithms cannot converge; when the number of measurements is suitably large, the number of measurements required for successful recovery has a negative correlation with the number of iterations and the algorithms can achieve linear convergence. Furthermore, the theory presents that the transition point of the number of measurements is on the order of $k \log({en}/{k})$, where $n$ is the dimension of the target signal.
更多查看译文
关键词
Compressed sensing,sparse optimization,data-time tradeoffs,optimal k-thresholding
AI 理解论文
溯源树
样例
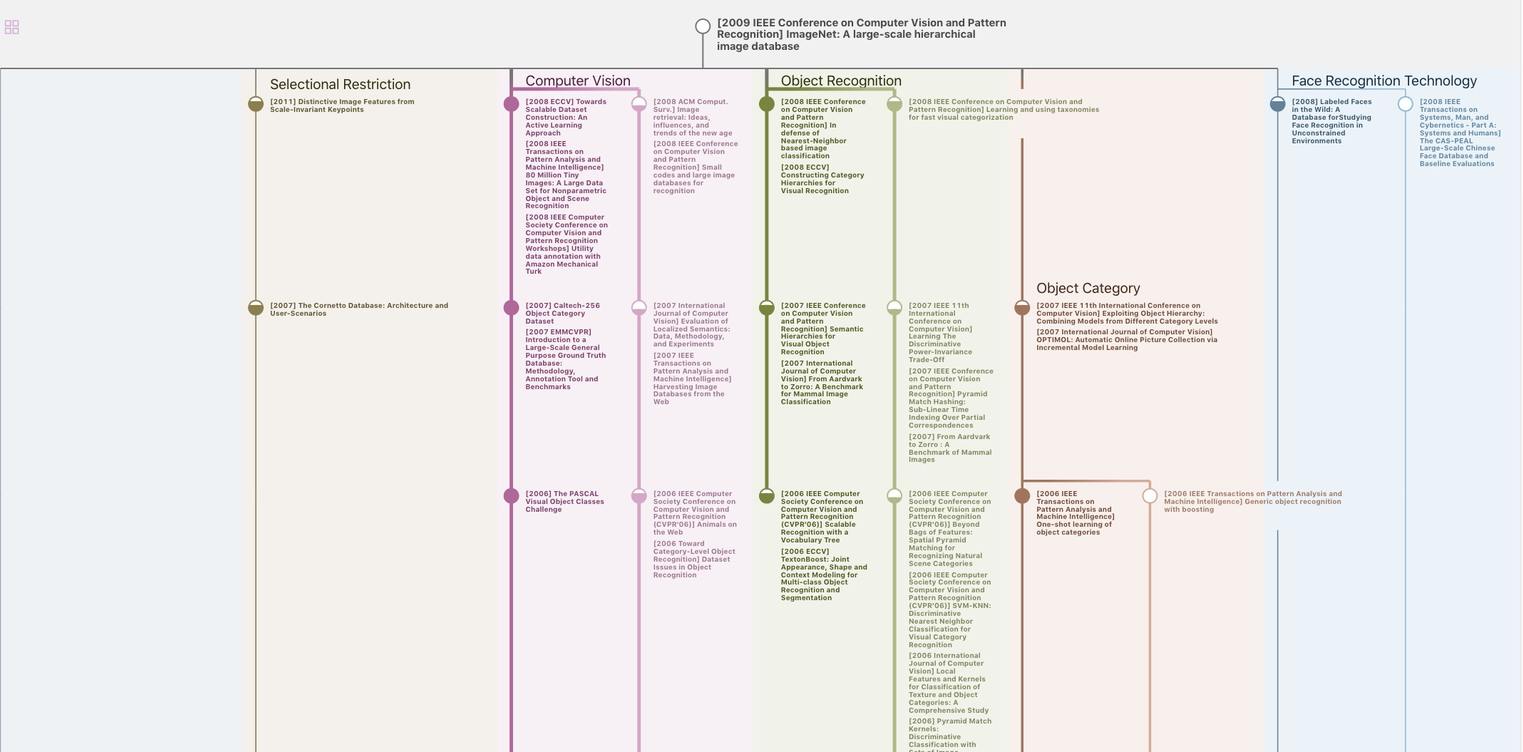
生成溯源树,研究论文发展脉络
Chat Paper
正在生成论文摘要