Persistent Homology with Improved Locality Information for more Effective Delineation
arxiv(2022)
摘要
Persistent Homology (PH) has been successfully used to train networks to detect curvilinear structures and to improve the topological quality of their results. However, existing methods are very global and ignore the location of topological features. In this paper, we remedy this by introducing a new filtration function that fuses two earlier approaches: thresholding-based filtration, previously used to train deep networks to segment medical images, and filtration with height functions, typically used to compare 2D and 3D shapes. We experimentally demonstrate that deep networks trained using our PH-based loss function yield reconstructions of road networks and neuronal processes that reflect ground-truth connectivity better than networks trained with existing loss functions based on PH. Code is available at https://github.com/doruk-oner/PH-TopoLoss.
更多查看译文
关键词
improved locality information
AI 理解论文
溯源树
样例
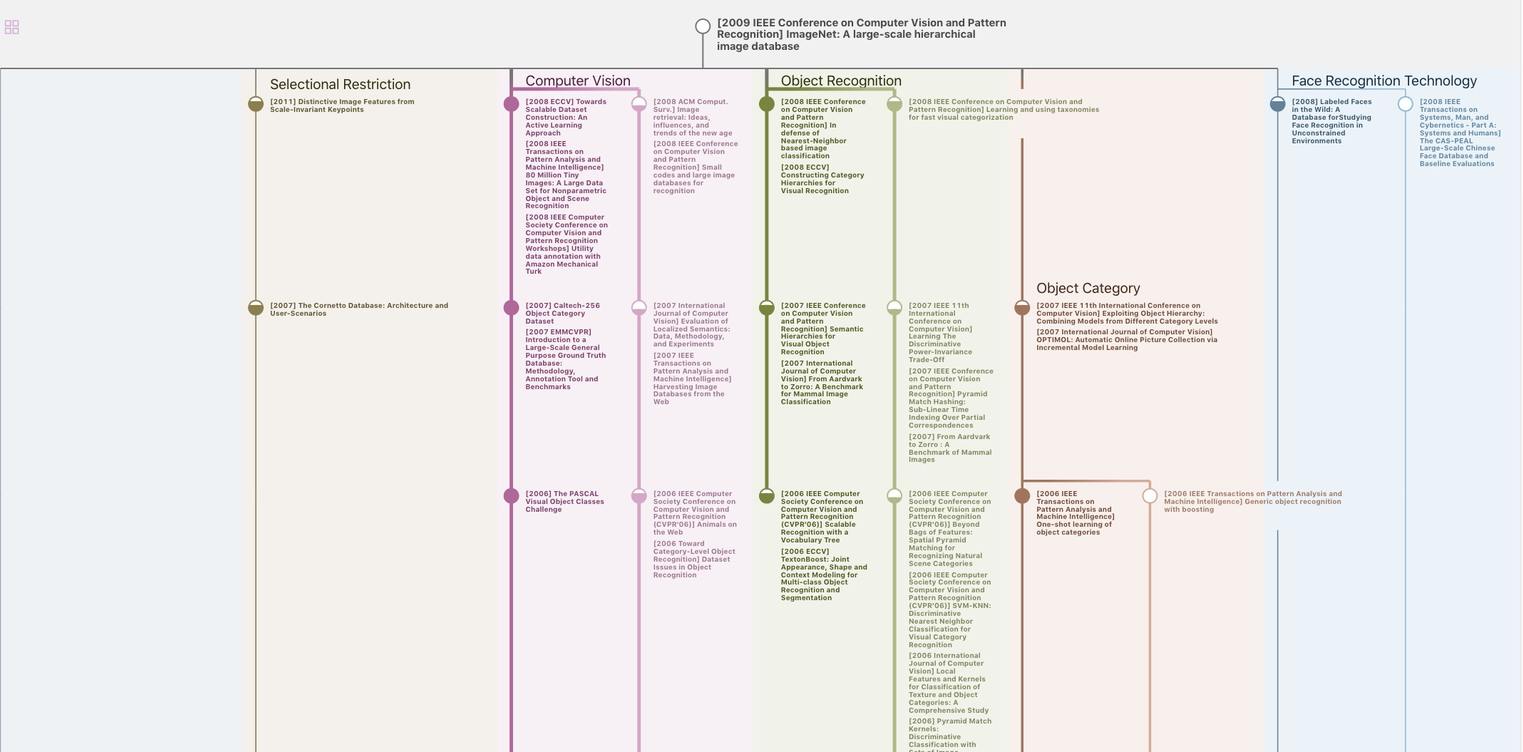
生成溯源树,研究论文发展脉络
Chat Paper
正在生成论文摘要