Label-Aware Ranked Loss for Robust People Counting Using Automotive In-Cabin Radar.
IEEE International Conference on Acoustics, Speech, and Signal Processing (ICASSP)(2022)
摘要
In this paper, we introduce the Label-Aware Ranked loss, a novel metric loss function. Compared to the state-of-the-art Deep Metric Learning losses, this function takes advantage of the ranked ordering of the labels in regression problems. To this end, we first show that the loss minimises when datapoints of different labels are ranked and laid at uniform angles between each other in the embedding space. Then, to measure its performance, we apply the proposed loss on a regression task of people counting with a short-range radar in a challenging scenario, namely a vehicle cabin. The introduced approach improves the accuracy as well as the neighboring labels accuracy up to 83.0% and 99.9%: An increase of 6.7%and 2.1% on state-of-the-art methods, respectively.
更多查看译文
关键词
Deep Metric Learning,People Counting,Radar Signal Processing
AI 理解论文
溯源树
样例
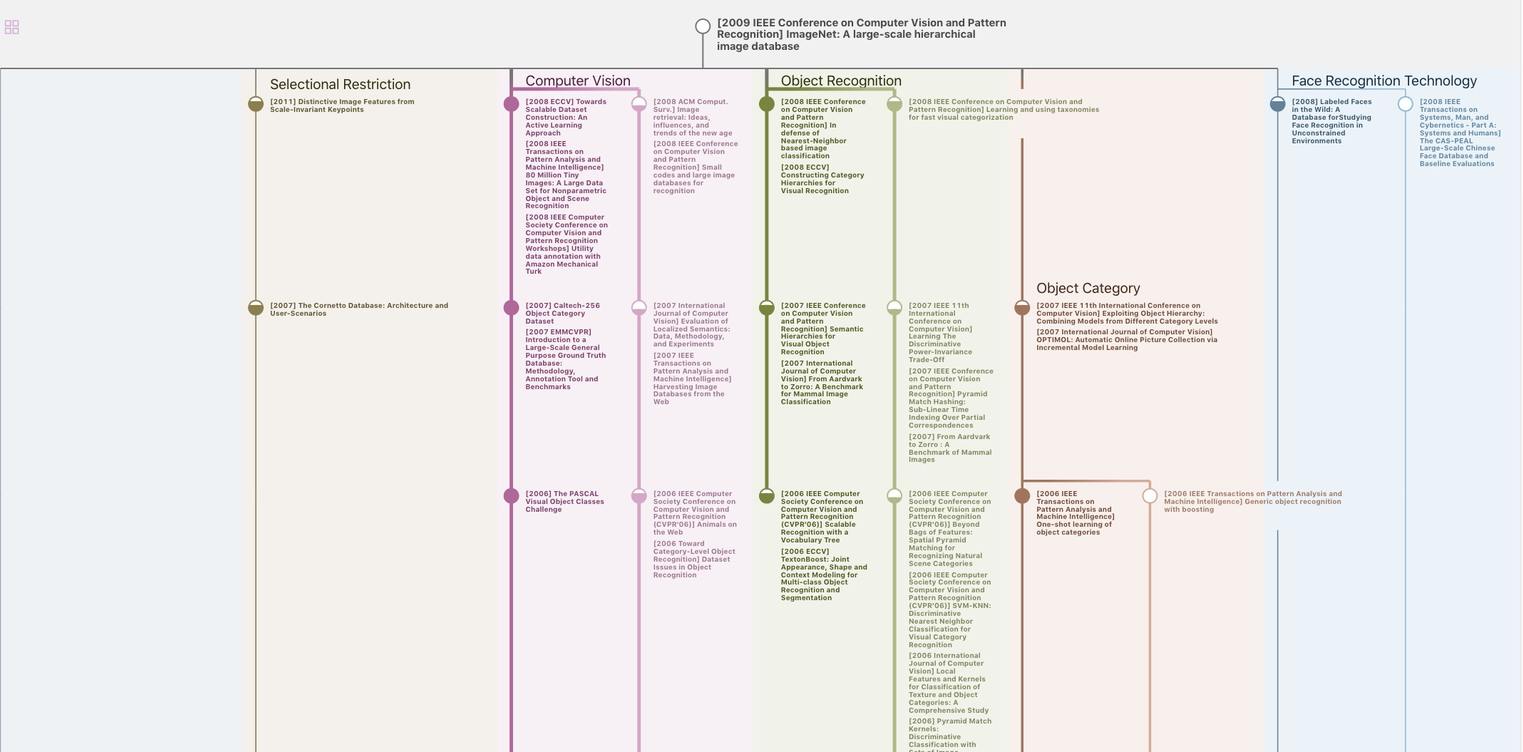
生成溯源树,研究论文发展脉络
Chat Paper
正在生成论文摘要