Adapting TTS models For New Speakers using Transfer Learning
arxiv(2021)
摘要
Training neural text-to-speech (TTS) models for a new speaker typically requires several hours of high quality speech data. Prior works on voice cloning attempt to address this challenge by adapting pre-trained multi-speaker TTS models for a new voice, using a few minutes of speech data of the new speaker. However, publicly available large multi-speaker datasets are often noisy, thereby resulting in TTS models that are not suitable for use in products. We address this challenge by proposing transfer-learning guidelines for adapting high quality single-speaker TTS models for a new speaker, using only a few minutes of speech data. We conduct an extensive study using different amounts of data for a new speaker and evaluate the synthesized speech in terms of naturalness and voice/style similarity to the target speaker. We find that fine-tuning a single-speaker TTS model on just 30 minutes of data, can yield comparable performance to a model trained from scratch on more than 27 hours of data for both male and female target speakers.
更多查看译文
关键词
tts models,transfer learning,new speakers
AI 理解论文
溯源树
样例
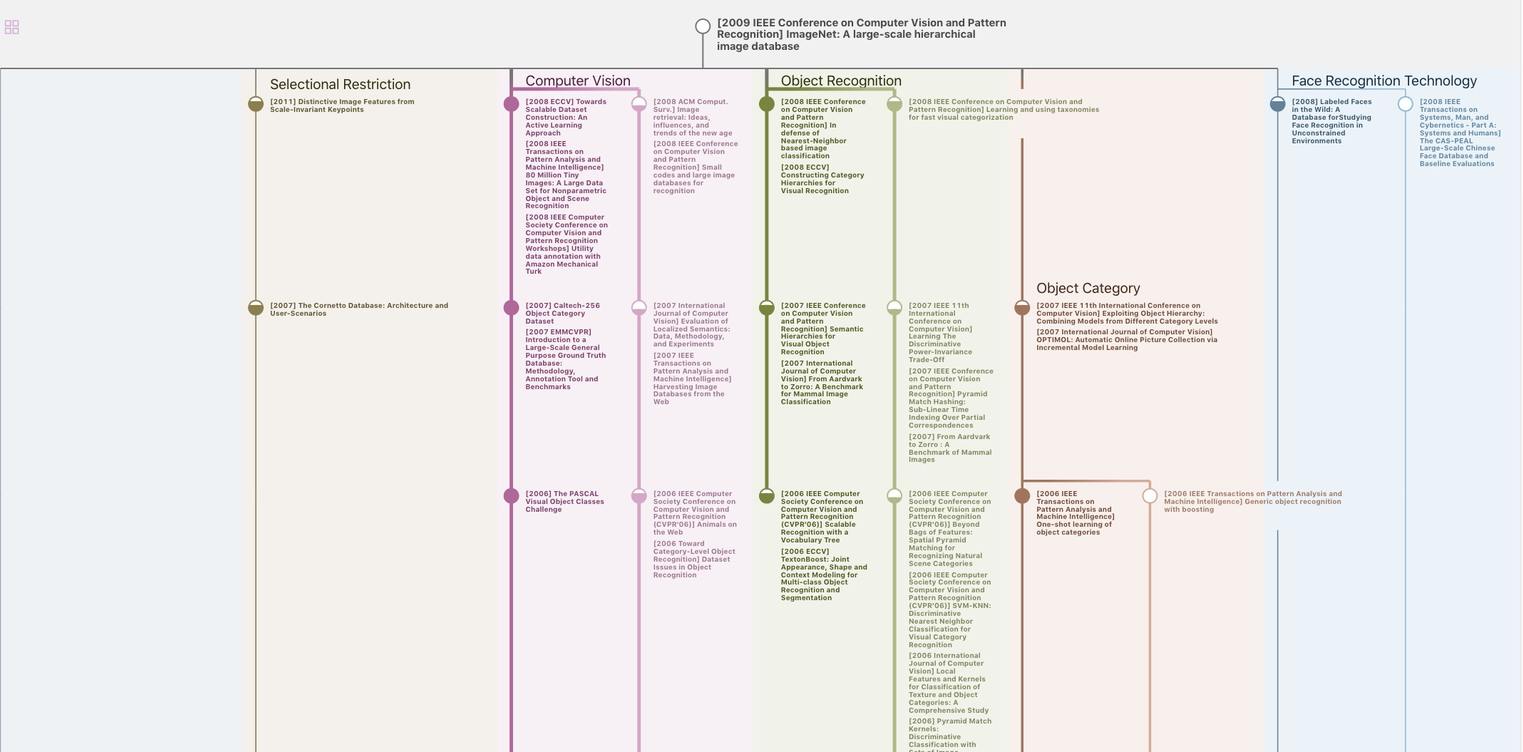
生成溯源树,研究论文发展脉络
Chat Paper
正在生成论文摘要