Generalizing to New Domains by Mapping Natural Language to Lifted LTL
IEEE International Conference on Robotics and Automation(2022)
摘要
Recent work on using natural language to specify commands to robots has grounded that language to LTL. However, mapping natural language task specifications to LTL task specifications using language models require probability distributions over finite vocabulary. Existing state-of-the-art methods have extended this finite vocabulary to include unseen terms from the input sequence to improve output generalization. However, novel out-of-vocabulary atomic propositions cannot be generated using these methods. To overcome this, we introduce an intermediate contextual query representation which can be learned from single positive task specification examples, associating a contextual query with an LTL template. We demonstrate that this intermediate representation allows for generalization over unseen object references, assuming accurate groundings are available. We compare our method of mapping natural language task specifications to intermediate contextual queries against state-of-the-art CopyNet models capable of translating natural language to LTL, by evaluating whether correct LTL for manipulation and navigation task specifications can be output, and show that our method outperforms the CopyNet model on unseen object references. We demonstrate that the grounded LTL our method outputs can be used for planning in a simulated OO-MDP environment. Finally, we discuss some common failure modes encountered when translating natural language task specifications to grounded LTL.
更多查看译文
关键词
mapping natural language,lifted ltl,new domains
AI 理解论文
溯源树
样例
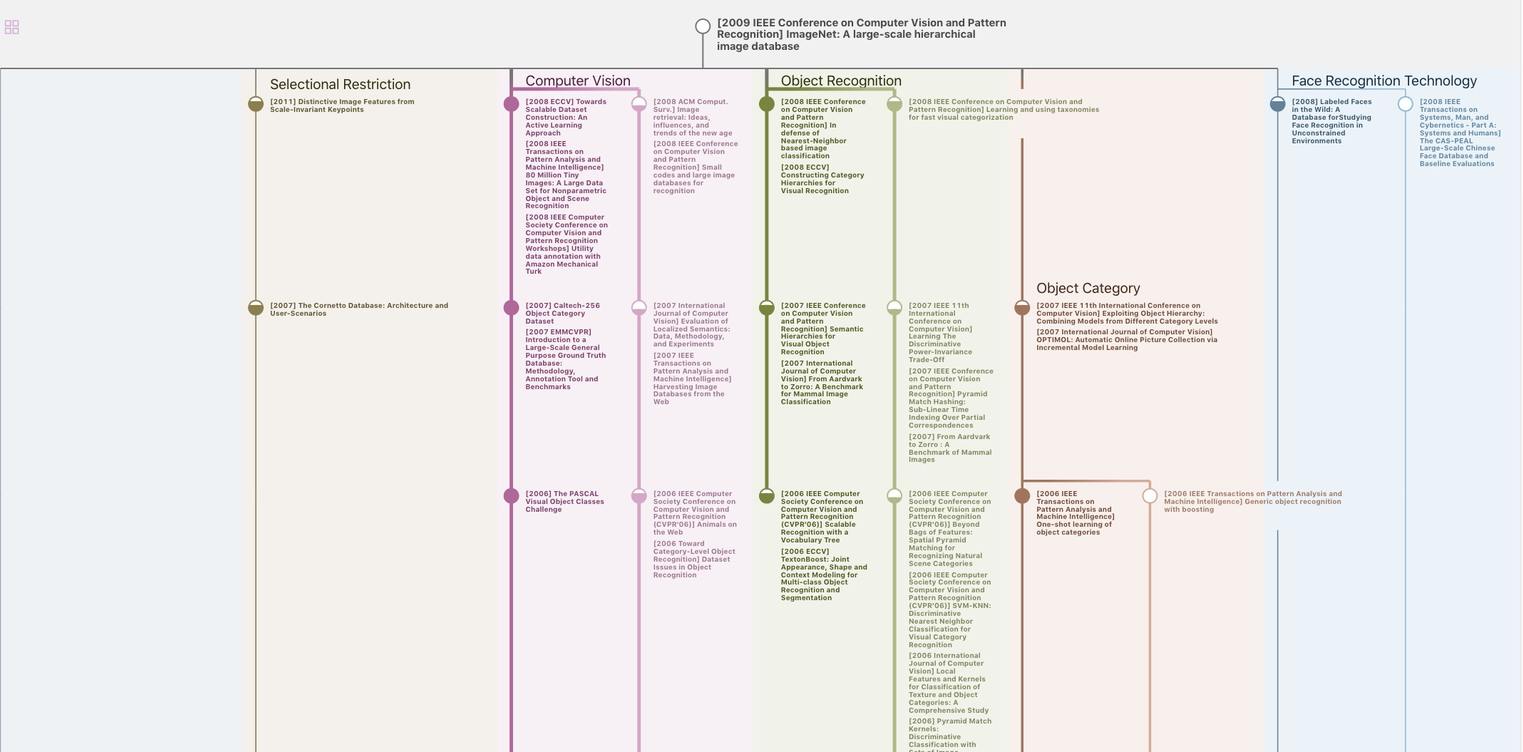
生成溯源树,研究论文发展脉络
Chat Paper
正在生成论文摘要