Uncertainty in Data-Driven Kalman Filtering for Partially Known State-Space Models
IEEE International Conference on Acoustics, Speech, and Signal Processing (ICASSP)(2022)
摘要
Providing a metric of uncertainty alongside a state estimate is often crucial when tracking a dynamical system. Classic state estimators, such as the Kalman filter (KF), provide a time-dependent uncertainty measure from knowledge of the underlying statistics, however, deep learning based tracking systems struggle to reliably characterize uncertainty. In this paper, we investigate the ability of KalmanNet, a recently proposed hybrid model-based deep state tracking algorithm, to estimate an uncertainty measure. By exploiting the interpretable nature of KalmanNet, we show that the error covariance matrix can be computed based on its internal features, as an uncertainty measure. We demonstrate that when the system dynamics are known, KalmanNet-which learns its mapping from data without access to the statistics-provides uncertainty similar to that provided by the KF; and while in the presence of evolution model-mismatch, KalmanNet pro-vides a more accurate error estimation.
更多查看译文
关键词
Kalman filter,deep learning,uncertainty
AI 理解论文
溯源树
样例
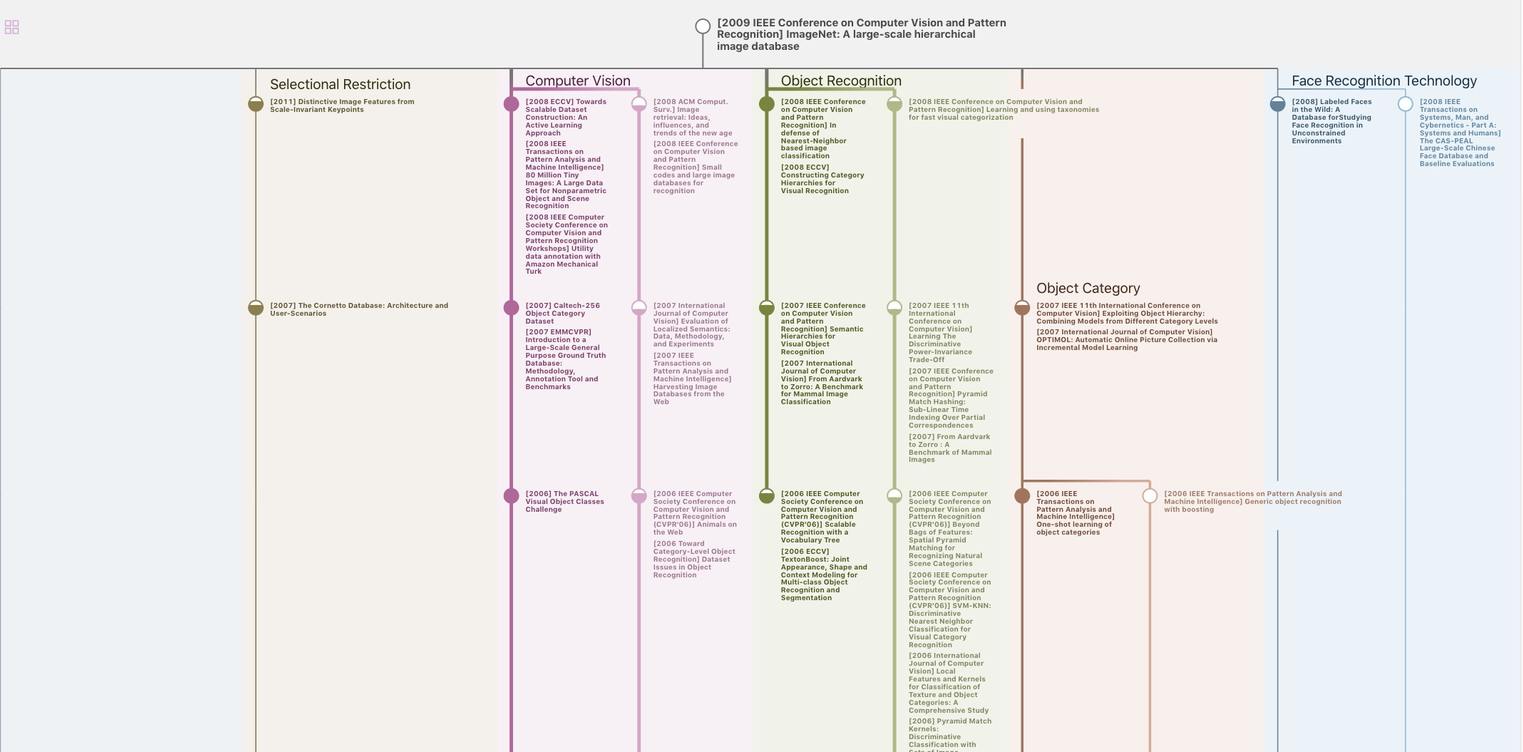
生成溯源树,研究论文发展脉络
Chat Paper
正在生成论文摘要