Towards Lifelong Learning of Multilingual Text-to-Speech Synthesis.
IEEE International Conference on Acoustics, Speech, and Signal Processing (ICASSP)(2022)
摘要
This work presents a lifelong learning approach to train a multilingual Text-To-Speech (TTS) system, where each language was seen as an individual task and was learned sequentially and continually. It does not require pooled data from all languages altogether, and thus alleviates the storage and computation burden. One of the challenges of lifelong learning methods is "catastrophic forgetting": in TTS scenario it means that model performance quickly degrades on previous languages when adapted to a new language. We approach this problem via a data-replay-based lifelong learning method. We formulate the replay process as a supervised learning problem, and propose a simple yet effective dual-sampler framework to tackle the heavily language-imbalanced training samples. Through objective and subjective evaluations, we show that this supervised learning formulation outperforms other gradient-based and regularization-based lifelong learning methods, achieving 43% Mel-Cepstral Distortion reduction compared to a fine-tuning baseline.
更多查看译文
关键词
Lifelong learning,Multilingual Text-To-Speech synthesis
AI 理解论文
溯源树
样例
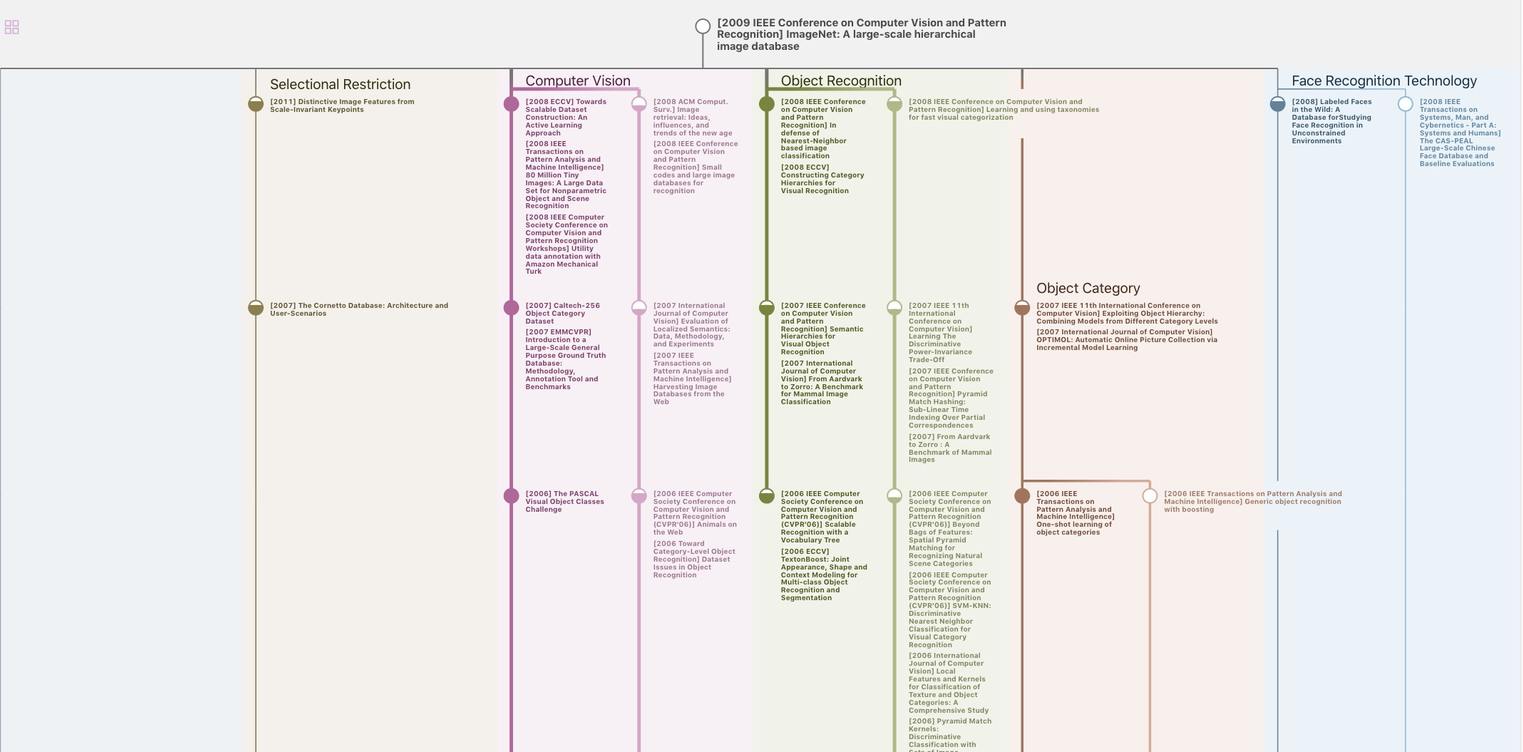
生成溯源树,研究论文发展脉络
Chat Paper
正在生成论文摘要