SCaLa: Supervised Contrastive Learning for End-to-End Speech Recognition
arxiv(2022)
摘要
End-to-end Automatic Speech Recognition (ASR) models are usually trained to optimize the loss of the whole token sequence, while neglecting explicit phonemic-granularity supervision. This could result in recognition errors due to similarphoneme confusion or phoneme reduction. To alleviate this problem, we propose a novel framework based on Supervised Contrastive Learning (SCaLa) to enhance phonemic representation learning for end-to-end ASR systems. Specifically, we extend the self-supervised Masked Contrastive Predictive Coding (MCPC) to a fully-supervised setting, where the supervision is applied in the following way. First, SCaLa masks variablelength encoder features according to phoneme boundaries given phoneme forced-alignment extracted from a pre-trained acoustic model; it then predicts the masked features via contrastive learning. The forced-alignment can provide phoneme labels to mitigate the noise introduced by positive-negative pairs in selfsupervised MCPC. Experiments on reading and spontaneous speech datasets show that our proposed approach achieves 2.84 and 1.38 points Character Error Rate (CER) absolute reductions compared to the baseline, respectively.
更多查看译文
关键词
speech recognition,automatic speech recognition,contrastive learning,end-to-end
AI 理解论文
溯源树
样例
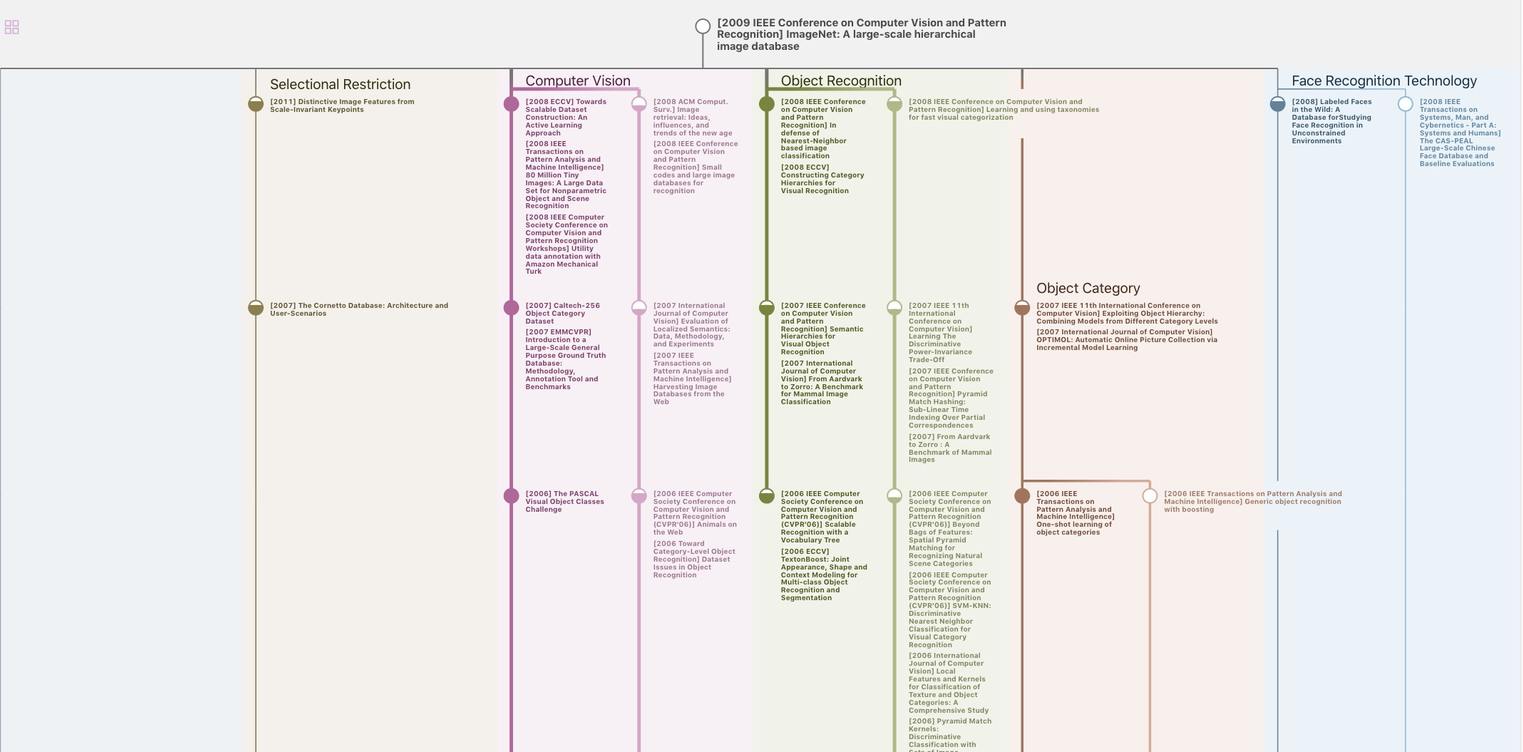
生成溯源树,研究论文发展脉络
Chat Paper
正在生成论文摘要