TRUNet: Transformer-Recurrent-U Network for End-to-end Multi-channel Reverberant Sound Source Separation
Conference of the International Speech Communication Association (INTERSPEECH)(2022)
Abstract
In recent years, many deep learning techniques for single-channel sound source separation have been proposed using recurrent, convolutional and transformer networks. When multiple microphones are available, spatial diversity between speakers and background noise in addition to spectro-temporal diversity can be exploited by using multi-channel filters for sound source separation. Aiming at end-to-end multi-channel source separation, in this paper we propose a transformerrecurrent-U network (TRUNet), which directly estimates multi-channel filters from multi-channel input spectra. TRUNet consists of a spatial processing network with an attention mechanism across microphone channels aiming at capturing the spatial diversity, and a spectro-temporal processing network aiming at capturing spectral and temporal diversities. In addition to multi-channel filters, we also consider estimating single-channel filters from multi-channel input spectra using TRUNet. We train the network on a large reverberant dataset using a proposed combined compressed mean-squared error loss function, which further improves the sound separation performance. We evaluate the network on a realistic and challenging reverberant dataset, generated from measured room impulse responses of an actual microphone array. The experimental results on realistic reverberant sound source separation show that the proposed TRUNet outperforms state-of-the-art single-channel and multi-channel source separation methods.
MoreTranslated text
Key words
sound source separation, deep learning, transformers, multi-channel filtering, spatial filtering
AI Read Science
Must-Reading Tree
Example
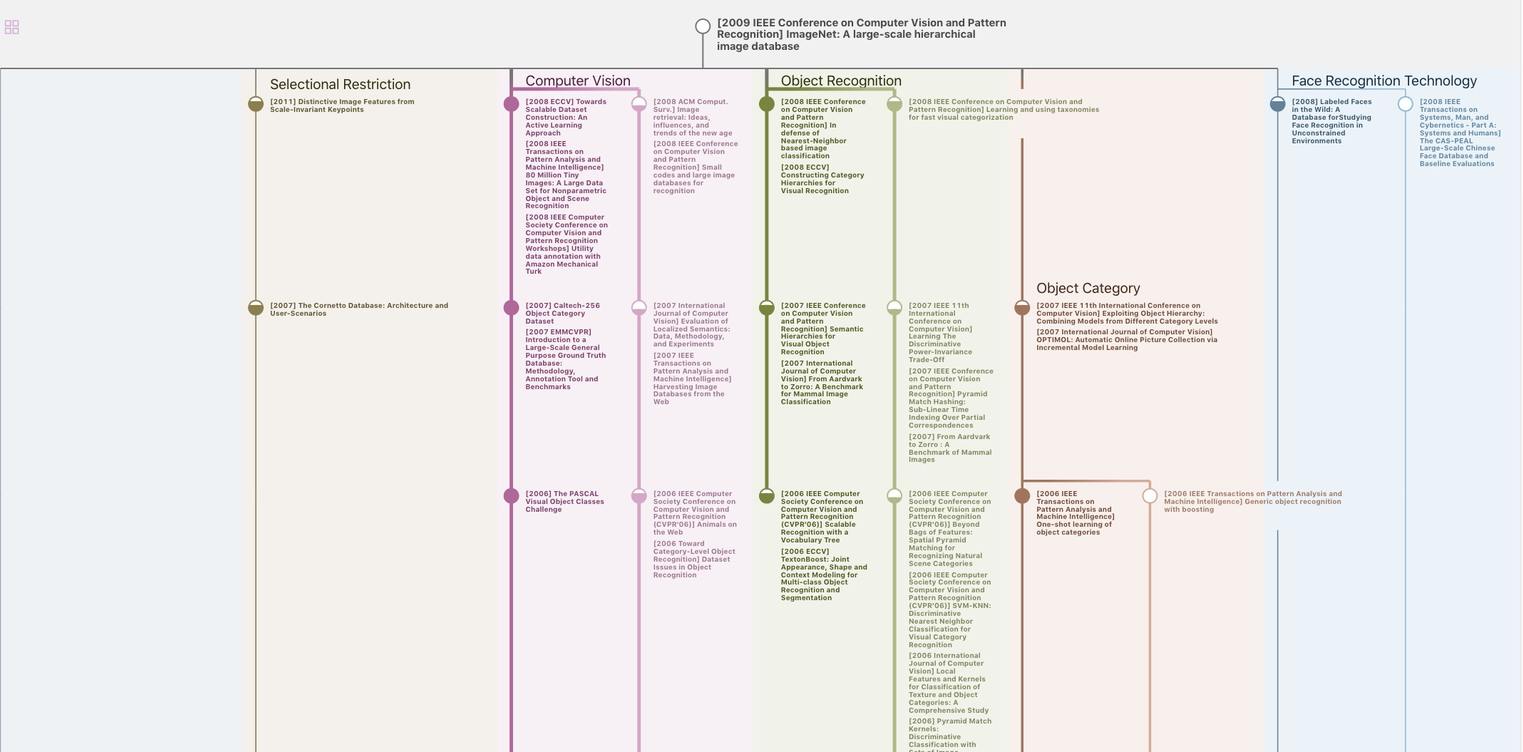
Generate MRT to find the research sequence of this paper
Chat Paper
Summary is being generated by the instructions you defined