Input Length Matters: An Empirical Study Of RNN-T And MWER Training For Long-form Telephony Speech Recognition
CoRR(2021)
Abstract
End-to-end models have achieved state-of-the-art results on several automatic speech recognition tasks. However, they perform poorly when evaluated on long-form data, e.g., minutes long conversational telephony audio. One reason the model fails on long-form speech is that it has only seen short utterances during training. This paper presents an empirical study on the effect of training utterance length on the word error rate (WER) for RNN-transducer (RNN-T) model. We compare two widely used training objectives, log loss (or RNN-T loss) and minimum word error rate (MWER) loss. We conduct experiments on telephony datasets in four languages. Our experiments show that for both losses, the WER on long-form speech reduces substantially as the training utterance length increases. The average relative WER gain is 15.7% for log loss and 8.8% for MWER loss. When training on short utterances, MWER loss leads to a lower WER than the log loss. Such difference between the two losses diminishes when the input length increases.
MoreTranslated text
Key words
mwer training,speech,long-form
AI Read Science
Must-Reading Tree
Example
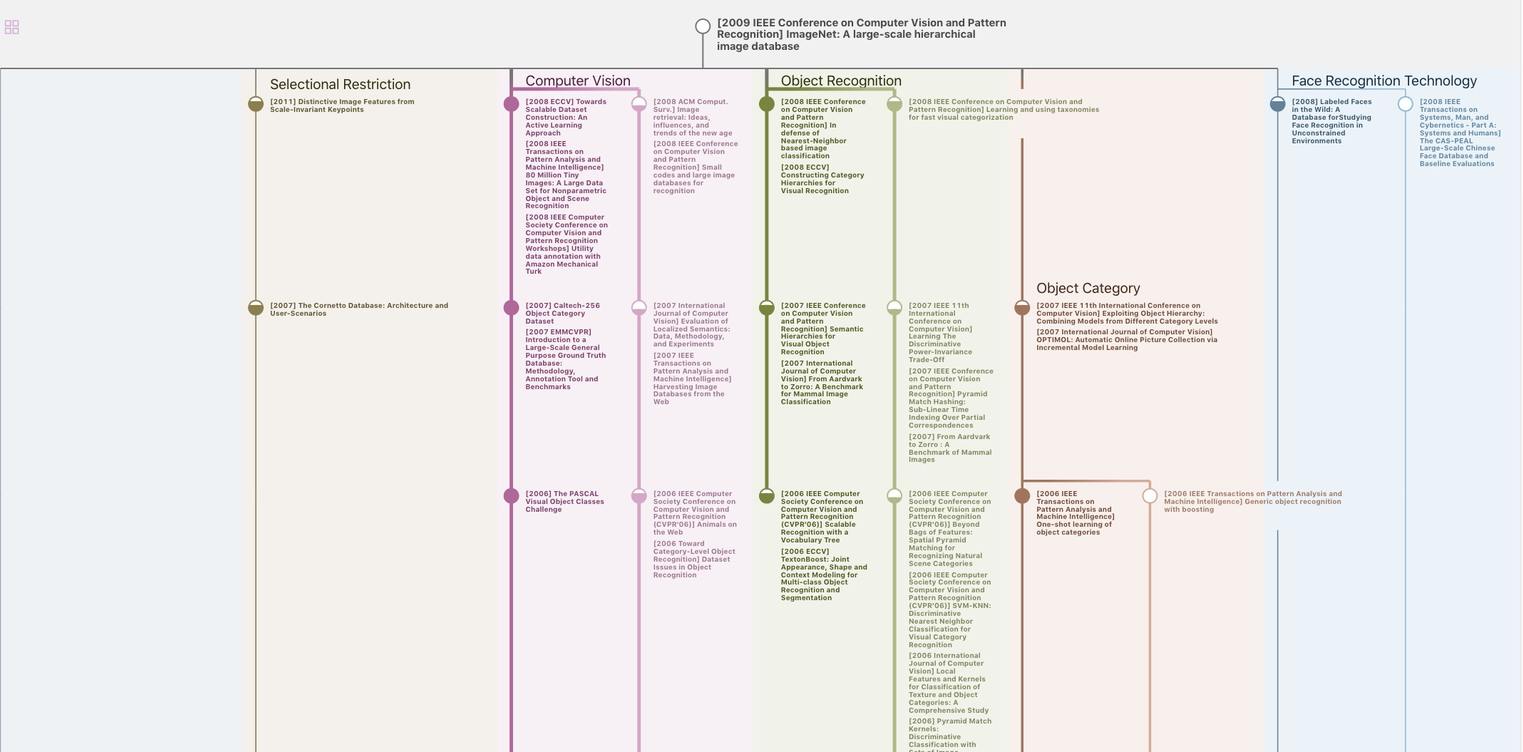
Generate MRT to find the research sequence of this paper
Chat Paper
Summary is being generated by the instructions you defined